AI in Biomedical Engineering: Transforming Healthcare
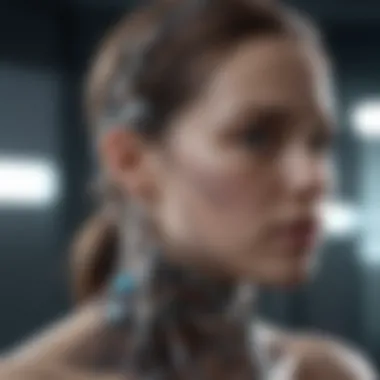
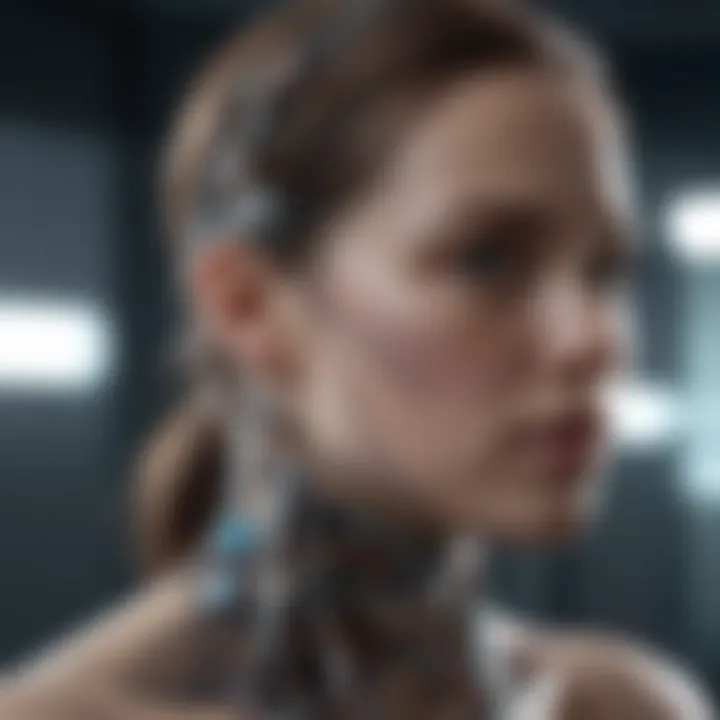
Article Overview
Purpose of the Article
This article aims to explore the significant role of artificial intelligence (AI) in the realm of biomedical engineering. AI technologies are not just enhancing existing processes; they are transforming the very foundations of healthcare and research methodologies. Through detailed analysis, this article will highlight the various applications of AI, particularly in areas like diagnostics and treatment planning. Additionally, it will touch on the ethical considerations surrounding these technologies, offering a well-rounded perspective for those involved in these fields.
Relevance to Multiple Disciplines
The intersection of AI and biomedical engineering is an area of tremendous interest and importance. This relationship has gained attention not only in healthcare but also in sectors such as data science, engineering, and ethical studies. AI provides tools that can improve patient outcomes and enhance research accuracy. Individuals from different backgrounds—students, educators, researchers, and professionals—will find valuable insights applicable to their specific disciplines, fostering interdisciplinary collaboration and understanding.
Research Background
Historical Context
The development of biomedical engineering dates back several decades, with roots in both medicine and engineering. Initially, the focus was primarily on mechanical devices and diagnostic instruments. However, advancements in computing and data processing were pivotal in shaping the future of healthcare. The emergence of AI technologies has accelerated this evolution, allowing for more complex analysis and interpretations of health data.
Key Concepts and Definitions
To appreciate the impact of AI in biomedical engineering, it is essential to understand some key concepts:
- Artificial Intelligence: Refers to automated algorithms capable of learning from data. These systems can recognize patterns, make decisions, and predict outcomes based on historical data.
- Biomedical Engineering: This field combines principles from various engineering disciplines with medical and biological sciences to develop technologies that improve healthcare delivery and outcomes.
- Machine Learning: A subset of AI that focuses on the development of algorithms that allow computers to learn from and make predictions based on data inputs.
- Deep Learning: A more complex form of machine learning that employs neural networks to analyze vast amounts of data for tasks such as image and speech recognition.
The subsequent sections will delve deeper into specific applications of AI in diagnostics and treatment, explore ethical implications, and discuss future trends in this exciting field.
Preface to AI in Biomedical Engineering
The intersection of artificial intelligence (AI) and biomedical engineering is reshaping the landscape of healthcare today. AI technologies possess the potential to improve efficiency in diagnostics, treatment, and research methodologies. Understanding the importance of AI in this field is crucial for grasping how healthcare and medical research can dramatically evolve.
Defining Biomedical Engineering
Biomedical engineering is a multidisciplinary field combining elements of engineering with medical and biological sciences. It focuses on designing and developing systems and products that improve medical care and enhance patient diagnostics. By utilizing principles of biology, materials science, chemistry, and physics, biomedical engineers aim to provide innovative solutions to healthcare challenges. This discipline includes a wide variety of subfields such as imaging, biomaterials, and tissue engineering, which showcase the synergy between engineering and medicine.
The Role of AI in Modern Medicine
AI is increasingly recognized for its vital role in modern medicine. The integration of AI algorithms significantly enhances decision-making processes in clinical settings. With its ability to analyze vast datasets, AI can identify patterns and trends that may be overlooked by human practitioners. For instance, diagnostic tools powered by AI can process medical images with remarkable precision, thus leading to faster diagnoses and better patient outcomes. Furthermore, AI's capacity for predictive analytics allows healthcare providers to foresee potential health issues and personalize treatment plans.
"AI not only aids accuracy but also streamlines operations in healthcare systems, allowing professionals to allocate time to direct patient care."
"AI not only aids accuracy but also streamlines operations in healthcare systems, allowing professionals to allocate time to direct patient care."
The benefits of AI encompass a range of areas. From improving patient safety through automated monitoring systems to enhancing the development process of pharmaceuticals, AI technologies are becoming essential in modern healthcare delivery. However, the real impact of AI manifests when it bridges the gap between biomedical engineering and healthcare, fostering a collaborative environment aimed at patient welfare.
Applications of AI in Diagnostics
The integration of artificial intelligence in diagnostics marks a significant advancement in how healthcare is approached and delivered. As medical technologies progress, the need for accurate, fast, and reliable diagnostic tools becomes ever more critical. AI technologies facilitate this transformation by enhancing both the efficiency and accuracy of disease detection and monitoring.
Image Analysis and Interpretation
In the realm of diagnostics, image analysis is a key area where AI has shown remarkable promise. Medical imaging modalities such as X-rays, MRIs, and CT scans generate vast amounts of data. Traditional analysis by radiologists can be time-consuming and subjective, which may lead to inconsistencies in interpretations. AI algorithms, particularly those based on deep learning, can assist in interpreting medical images more quickly and accurately. These algorithms are capable of detecting subtle patterns that may not be easily visible to the human eye.
The benefits of AI in image analysis include:
- Enhanced Accuracy: AI tools can reduce the chances of misdiagnosis by identifying abnormalities more reliably.
- Speed of Diagnosis: AI can process images in real-time, which is crucial for urgent cases where time is of the essence.
- Consistency: AI systems provide uniform analysis, minimizing variations that can arise from human interpretation.
The application of AI to image interpretation has been seen in several successful implementations, such as the identification of tumors in radiological scans or the detection of diabetic retinopathy from retinal images. However, it is important to note that while AI can aid in diagnosis, it does not replace the need for human expertise; instead, it acts as a support tool that radiologists can utilize to make more informed decisions.
Predictive Analytics in Patient Diagnosis
Predictive analytics stands as another cornerstone of AI applications in diagnostics. By analyzing historical data and identifying risk factors, AI can predict the likelihood of various health outcomes. This is especially beneficial in managing chronic diseases where early intervention can significantly improve patient outcomes.
AI models analyze large datasets from electronic health records and incorporate various factors such as:
- Patient Demographics: Age, sex, and ethnicity can influence health risks.
- Medical History: Prior conditions can inform predictions about future health issues.
- Lifestyle Factors: Diet, exercise, and smoking habits provide context for health assessments.
These predictive insights enable healthcare providers to:
- Implement Proactive Measures: Knowing a patient's risk level for diseases such as diabetes or heart disease allows for preventive strategies to be put in place.
- Customize Treatment Plans: AI can help in tailoring interventions that align with an individual's health profile.
- Improve Resource Allocation: Understanding patient risks can assist healthcare systems in distributing resources efficiently, directing attention to higher-risk individuals.
The use of predictive analytics in patient diagnosis not only enhances the precision in treatments but also boosts overall healthcare system efficiency. As more data becomes available, the potential for AI to fine-tune diagnostic processes will only increase, propelling the biomedical field forward.
AI in Treatment Planning
The integration of artificial intelligence (AI) into treatment planning is a significant advancement in biomedical engineering. Treatment planning involves crafting effective strategies tailored to individual patient needs based on various clinical data points. AI enhances this process by providing intelligent systems that can analyze vast amounts of data rapidly. This capability results in more precise treatment protocols, improves clinical outcomes, and optimizes resource allocation in healthcare settings.
AI technologies streamline the decision-making process in treatment planning. For instance, algorithms can process historical data, identify patterns, and provide personalized recommendations. This results in a more individualized approach to therapy, ensuring that each patient receives care that aligns with their unique health profile.
In this section, we will explore two key areas within AI in treatment planning: Personalized Medicine and AI Algorithms, as well as AI in Drug Development. These areas reflect the current landscape of healthcare where AI is crucial in reshaping therapeutic strategies.
Personalized Medicine and AI Algorithms
Personalized medicine represents a paradigm shift in healthcare, focusing on tailoring treatment strategies based on individual patient characteristics. AI algorithms play a central role in this process. By analyzing genomic data, clinical histories, and lifestyle factors, AI can identify which treatments are most likely to be effective for a specific patient.
- Genome Sequencing: Advanced AI models analyze genetic data, allowing healthcare providers to understand how genetic variations affect drug metabolism and treatment response.
- Predictive Modeling: These algorithms can predict disease progression and response to treatments based on past outcomes, increasing the chance of successful interventions.
The use of AI in personalized medicine also leads to cost efficiency. Healthcare systems can reduce expenditure on ineffective treatments. By focusing on interventions that have higher efficacy for individual patients, resources are used more judiciously, leading to better outcomes without unnecessary financial burden.
AI in Drug Development
The drug development process traditionally entails long timelines and high costs. AI is changing this by introducing computational methods that accelerate drug discovery and development.
Using AI technologies, researchers can:
- Analyze biological data: AI algorithms help in identifying potential drug targets by understanding proteins' structures and functions within the body.
- Predict drug interactions: Machine learning models can forecast how new drugs might interact with existing medications or biological pathways.
- Reduce trial and error: By applying AI, drug candidates can be selected and optimized for specific diseases, saving time and resources during clinical trials.
Robotics and AI in Surgery
Robotics and artificial intelligence (AI) are making notable strides in the field of surgery. The incorporation of these technologies seeks to enhance the precision, efficiency, and outcomes of surgical procedures. This section will provide an overview of the relevance and impact that robotics and AI have on surgical practices today.
The Emergence of Surgical Robots
Surgical robots have transitioned from concept to reality, enabling surgeons to perform complex procedures with elevated dexterity. Systems like the da Vinci Surgical System illustrate how robotics augment traditional techniques. The ability to maneuver instruments with precision and access smaller spaces is a game changer for minimally invasive procedures.
Benefits of surgical robots include:
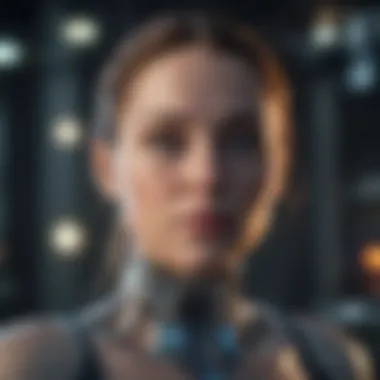
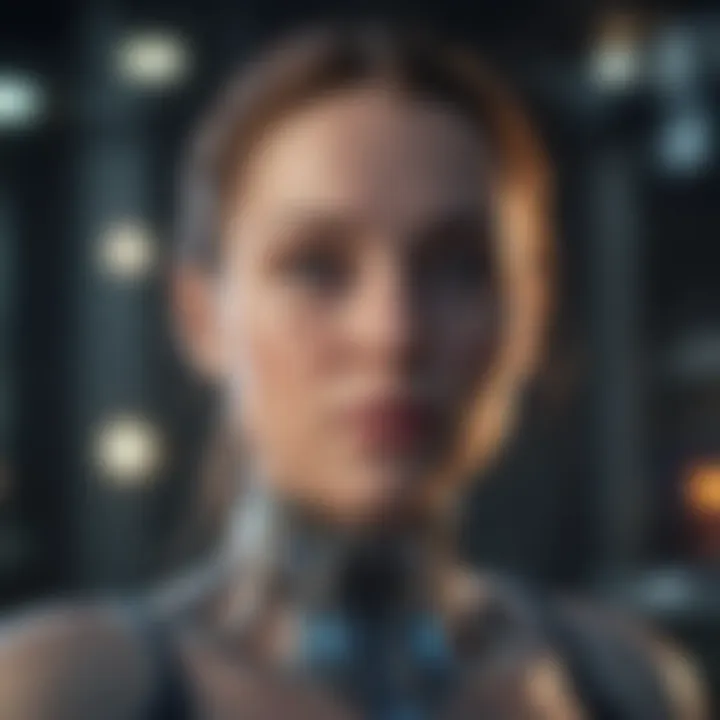
- Improved Accuracy: Robots offer enhanced control in movements, aiding surgeons in reducing human error.
- Minimal Mishaps: These technologies showcase a decrease in complication rates due to their inherent stability.
- Shortened Recovery Times: As a result of minimally invasive operations, patients benefit from quicker recovery and less pain.
Moreover, these systems can be equipped with AI functionalities. They not only assist surgeons but also provide simulations that improve skills through training.
AI-Assisted Surgical Procedures
AI is paving the way for smarter surgical applications. By integrating AI with surgical robots, healthcare professionals experience a new landscape of possibilities. AI’s role is multifaceted, from pre-surgical planning to real-time surgical assistance.
Key functionalities of AI in surgery include:
- Data Analysis: AI can analyze vast amounts of patient data and imaging before surgery, improving the clarity of surgical plans.
- Real-Time Decisions: During procedures, AI tools can process information and offer suggestions, enhancing the decision-making process.
- Predictive Capabilities: By evaluating past patient outcomes and data, AI algorithms can forecast potential complications, making the surgeries more tailored and safe.
"AI technologies in surgical settings not only improve the precision but also enhance overall patient safety during operations."
"AI technologies in surgical settings not only improve the precision but also enhance overall patient safety during operations."
As surgery evolves, the collaboration between robotics and AI addresses modern medical demands. Surgeons today are better equipped to handle intricate situations, reducing risks and enhancing patient care.
In summary, both robotics and AI play crucial roles in advancing surgical innovation. Their emergence in surgical practices enables a combination of efficiency and effectiveness, which ultimately aims to enhance patient outcomes.
AI Technologies in Biomedical Research
The intersection of AI and biomedical research has gained significant importance in recent years. The application of AI technologies enhances our ability to process and analyze large amounts of biological data efficiently. Researchers are increasingly utilizing these technologies to gain insights into complex biological phenomena and improve healthcare outcomes.
One major advantage of AI technologies in this field is their capacity for data mining and machine learning. This not only accelerates the research process but also allows for a more comprehensive understanding of disease mechanisms.
Data Mining and Machine Learning
Data mining involves extracting valuable information from large datasets. In biomedical research, it plays a crucial role in uncovering patterns that can inform experimental designs and clinical decisions. Machine learning algorithms can analyze diverse types of data, including genomics, proteomics, and even clinical records.
Here are some of the key benefits of integrating data mining and machine learning in biomedical research:
- Increased Efficiency: Machine learning algorithms can analyze complex data much faster than traditional methods.
- Improved Accuracy: These technologies reduce human error in data interpretation by relying on statistical patterns instead of subjective judgment.
- Predictive Modeling: AI can help predict disease outcomes based on genetic and clinical data, aiding in the design of personalized treatment plans.
Using these advancements, researchers can not only identify new biomarkers for diseases, but also validate their findings more robustly.
Modeling Biological Processes
Modeling biological processes is another critical aspect of AI technologies in biomedical research. Here, AI methods create simulations that can replicate biological systems. This can range from cellular processes to entire metabolic pathways. By employing algorithms that mimic biological interactions, scientists gain a deeper understanding of how these systems function.
The advantages of modeling biological processes include:
- Insights into Disease Mechanisms: AI-generated models allow researchers to test hypotheses about disease progression and response to treatments without needing extensive laboratory work.
- Drug Discovery: AI models can simulate how drugs interact with biological targets, expediting the drug development process significantly.
- Experimental Designs: Researchers can use models to predict outcomes in laboratory experiments, refining their hypotheses and making more informed decisions.
"AI technologies are not just tools in biomedical research; they are reshaping the future of how we understand and interact with biological systems."
"AI technologies are not just tools in biomedical research; they are reshaping the future of how we understand and interact with biological systems."
Ethical Considerations of AI in Healthcare
The integration of artificial intelligence (AI) into healthcare brings significant advancements but also raises important ethical questions. Understanding these considerations is essential for fostering responsible development and deployment of AI technologies in biomedical engineering. When used appropriately, AI can enhance health outcomes, improve patient safety, and streamline research processes. However, without addressing ethical concerns, these advancements may perpetuate harms or exacerbate existing inequalities.
Key aspects of ethical considerations in AI include:
- Protecting patient rights and maintaining trust.
- Balancing innovation with safety and ethical implications.
- Ensuring transparency in how AI models operate and make decisions.
Addressing these ethical implications is vital for stakeholders involved in the development and implementation of AI. Health professionals and researchers must prioritize ethical frameworks to guide their work, especially as AI becomes more embedded in healthcare systems.
Privacy and Data Security
In the age of digital information, privacy and data security are paramount. AI systems in healthcare often require access to sensitive patient data, which can lead to potential breaches. Hospitals and research institutions must implement robust security measures to protect this information from unauthorized access, misuse, or theft. Failure to safeguard patient data can result in significant legal ramifications and damage to institutional reputations.
strategies to enhance privacy include:
- Data Anonymization: Techniques to remove personal identifiers from datasets to mitigate risks.
- Encryption: Protecting sensitive information through complex algorithms.
- Access Controls: Implementing strict permissions to ensure that only authorized personnel can view or manipulate patient data.
Educating staff on data security protocols is equally important. This creates a culture of awareness that minimizes the risks associated with data handling.
Bias and Fairness in AI Algorithms
Bias in AI algorithms poses a serious ethical challenge. AI systems can unintentionally reflect societal biases present in their training data. This can lead to discriminatory practices in diagnostics or treatment recommendations, disproportionately affecting marginalized groups. Bias detection and correction are necessary steps in the development of fair and equitable AI solutions.
To combat bias effectively, the following practices should be adopted:
- Diverse Datasets: Ensuring that training datasets represent a broad spectrum of demographics, reducing the risk of systemic bias.
- Regular Audits: Conducting periodic evaluations of AI systems to identify biases in outputs and performance metrics.
- Involvement of Stakeholders: Including diverse stakeholders in the design and analysis of AI systems promotes various perspectives, which can enhance fairness.
The commitment to creating unbiased AI not only fosters social responsibility but also enhances the credibility and reliability of AI tools in healthcare. By actively addressing issues of bias and fairness, the field can harness AI's potential in a socially responsible manner.
"Ethical considerations are not just a regulatory obligation; they are fundamental to ensuring trust and integrity in AI applications."
"Ethical considerations are not just a regulatory obligation; they are fundamental to ensuring trust and integrity in AI applications."
Future Directions of AI in Biomedical Engineering
The exploration of Future Directions of AI in Biomedical Engineering is essential in understanding the ongoing developments in healthcare. As technology evolves, so does the potential for artificial intelligence to influence biomedical engineering practices. The application of AI methods could significantly enhance outcomes in diagnostics, patient care, and research methodologies. These future trends hold promise for more precise and effective healthcare solutions.
Emerging Trends in AI Research
In recent years, numerous emerging trends have become apparent in AI research related to biomedical engineering. One notable trend is the development of advanced machine learning algorithms, such as deep learning, which are being utilized to analyze complex medical data. These algorithms can uncover patterns that are not easily identifiable by human experts. The capability to process large datasets, like genomic sequences or imaging studies, could lead to breakthroughs in personalized medicine.
Moreover, natural language processing is gaining traction, allowing AI systems to interpret and analyze medical literature and patient records effectively. This can streamline clinical workflows and assist healthcare professionals in decision-making processes. There is also increasing interest in reinforcement learning applications, where AI systems learn optimal strategies for treatment plans based on patient responses.
In addition to these advancements, collaboration among interdisciplinary teams is becoming more common. Engineers, clinicians, and data scientists are joining forces to tackle complex medical challenges. This collaborative approach is driving innovation and improving the implementation of AI technologies in biomedical contexts.
Integration of AI with Other Technologies
The integration of AI with other emerging technologies presents a unique opportunity to advance the biomedical field. For instance, combining AI with big data analytics significantly enhances predictive modeling capabilities. Medical professionals can anticipate patient needs by analyzing trends in extensive datasets, improving resource allocation and patient outcomes.
Moreover, AI-powered wearable devices are revolutionizing patient monitoring. These devices collect real-time health data, which can be analyzed using AI algorithms to provide insights into individual health trends. This allows for proactive management of chronic conditions, leading to better patient engagement and adherence to treatment plans.
Telemedicine is another area where AI and technology converge. AI can enhance remote consultations by automatically analyzing patient data before appointments, providing clinicians with tailored recommendations and improving the overall patient experience.
"As AI continues to advance, the collaborative integration of these technologies will mark a crucial turning point in biomedical engineering and healthcare delivery."

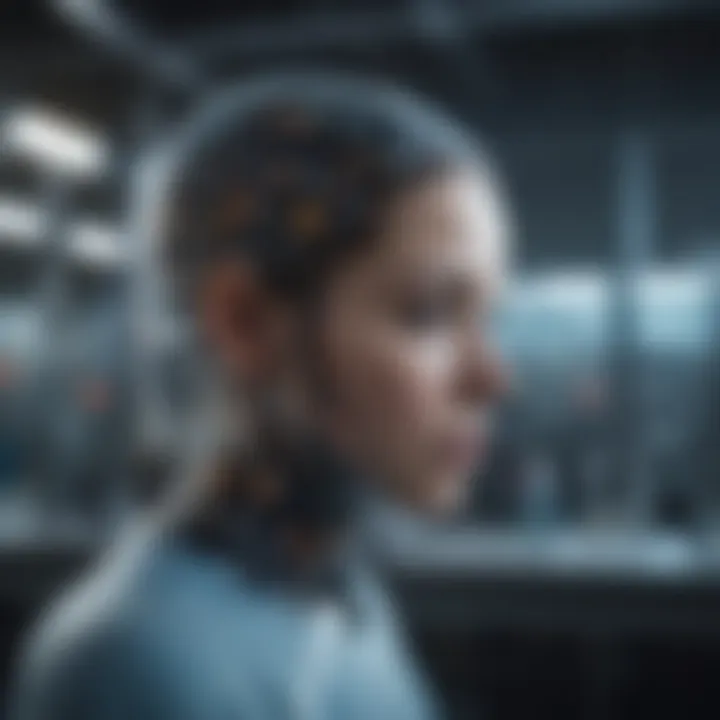
"As AI continues to advance, the collaborative integration of these technologies will mark a crucial turning point in biomedical engineering and healthcare delivery."
Challenges in Implementing AI in Biomedical Fields
The integration of artificial intelligence in biomedical engineering is promising, yet it comes with its own set of challenges. Understanding these obstacles is crucial for researchers and practitioners who aim to adopt AI technologies in healthcare settings. The significance on focusing on these challenges lies in creating a robust framework for implementation, ensuring safety and efficacy in AI applications.
Technical Barriers
One of the primary technical barriers faced in the implementation of AI is the complexity of biomedical data. Medical data is often unstructured and varies in format. For instance, imaging data from CT scans does not resemble structured data like patient records. The inconsistency in data quality can affect the performance of AI models, leading to unreliable outcomes.
Moreover, integrating AI systems with existing healthcare information systems can be a daunting task. Many hospitals and institutions operate on legacy systems that may not support modern AI tools. The integration process may require considerable investment in terms of both time and resources.
"A successful AI implementation requires not only advanced algorithms but also high-quality input data and infrastructure"
"A successful AI implementation requires not only advanced algorithms but also high-quality input data and infrastructure"
Additionally, data privacy and security concerns are paramount. AI systems usually require vast amounts of sensitive patient information. Ensuring compliance with data protection regulations, such as HIPAA in the United States, poses another layer of challenge to healthcare providers.
Regulatory Hurdles
Regulatory hurdles represent a significant challenge in the deployment of AI in the biomedical field. The rapid pace of AI development often outstrips existing regulatory frameworks, creating uncertainty for developers and institutions. In many cases, AI innovations do not fit neatly into existing categories defined by regulatory bodies. This can hinder the approval process and delay the availability of new solutions for patient care.
Different countries have varying regulations concerning medical devices and AI technologies. The lack of harmonization in these regulations can act as a barrier to global collaboration in research and technology transfer. For instance, what might be deemed safe and effective in one region may not pass the regulatory standards in another.
Furthermore, there is an ongoing debate about how to evaluate and validate AI-driven solutions. Questions on how to appropriately test the algorithms and what metrics to employ remain largely unresolved. Addressing these regulatory challenges is vital for fostering innovation while protecting patient safety.
Education and Training in AI and Biomedical Engineering
Education and training in the domain of AI and biomedical engineering is crucial for several reasons. As artificial intelligence technology becomes more pervasive in healthcare and medical research, professionals must be equipped with the skills and knowledge necessary to navigate this complex landscape. The integration of ethical considerations, hands-on training, and a strong theoretical foundation forms the backbone of a well-rounded education in this field.
With the rapid advancements in AI tools and technologies, it’s vital to create curriculums that reflect current trends and future predictions. Educators face the challenge of ensuring that their teaching material is up-to-date while also covering fundamental principles. Students in these programs should learn how to apply AI methods effectively in real-world biomedical scenarios, thereby enhancing their employability and readiness for professional challenges.
An emphasis on practical experience through case studies, internships, and collaborative projects can significantly enrich learning. Students can better understand how to use AI in diagnostics, treatment planning, and research by addressing actual problems encountered in the biomedical field. This hands-on approach allows for a deeper comprehension of theoretical knowledge and its applications.
Curriculum Development
Curriculum development plays a significant role in addressing the educational needs within AI and biomedical engineering. Programs must be multidisciplinary, combining aspects of engineering, computer science, biology, and medicine. A well-structured program would typically include courses on:
- Machine learning and data analytics
- Biomedical signal processing
- Robotics and automation in surgery
- Ethical issues in AI applications
Such a diverse curriculum ensures that students are prepared for the multifaceted challenges they may encounter in their careers. Incorporating project-based learning and real-world cases helps foster critical thinking and problem-solving skills, which are essential in this dynamic field.
Moreover, partnerships with industry leaders can facilitate access to the latest technologies and research. By creating links with organizations involved in AI research, educational institutions can keep their curriculum relevant and attuned to industry needs. This responsiveness can attract students and aid in the opportunity for future employment post-graduation.
Interdisciplinary Learning Opportunities
AI in biomedical engineering thrives on interdisciplinary collaboration. Educational programs should actively promote learning opportunities that span several disciplines. Courses that foster teamwork among students in engineering, medical, and data science fields can create a comprehensive environment for learning. This collaboration is essential for practical applications where engineers, medical professionals, and data scientists work together.
Workshops and seminars featuring speakers from diverse backgrounds can present students with a holistic view of the field. Interdisciplinary projects can also encourage innovative thinking and the exchange of ideas that may lead to breakthroughs in patient care and research methodologies.
"Investing in education and training equips individuals with the tools to unlock the potential of AI in healthcare and biomedical engineering."
"Investing in education and training equips individuals with the tools to unlock the potential of AI in healthcare and biomedical engineering."
By ensuring that the curriculum is adaptive and fosters interdisciplinary connections, we can facilitate the growth of a well-rounded workforce ready to tackle the upcoming challenges.
Case Studies of AI Implementation
Case studies of AI implementation in biomedical engineering serve as practical illustrations of how artificial intelligence is reshaping healthcare practices and research methodologies. They provide insights into effective applications, challenges faced during integration, and the ensuing benefits that AI brings to the medical field. These case studies bridge the gap between theoretical knowledge and real-world application, highlighting the potential of AI to improve patient care, streamline processes, and drive innovative research.
Stakeholders in healthcare—including medical professionals, researchers, and educators—benefit significantly from studying these cases. Understanding specific implementations aids in identifying best practices, guiding future projects, and establishing benchmarks for evaluating the efficacy of AI technologies. Furthermore, these case studies offer a foundation for evidence-based discussions about the future trajectory of AI in biomedicine, emphasizing that while challenges exist, the opportunities presented are substantial.
Successful AI Applications in Hospitals
Hospitals across the globe are increasingly adopting AI applications to enhance operational efficiency and patient outcomes. One noteworthy example is the use of IBM Watson in oncology. Watson assists oncologists by analyzing vast arrays of medical literature and clinical data to recommend tailored treatment options for cancer patients. This application significantly reduces the time required for oncologists to develop treatment plans, thus allowing for quicker interventions.
In addition to oncology, AI-powered diagnostic tools like Aidoc are transforming radiology. Aidoc uses deep learning algorithms to analyze medical images and flag abnormalities, such as brain hemorrhages, in real-time. This capability enables radiologists to prioritize urgent cases, ultimately improving patient prognosis through timely interventions.
The integration of AI in hospitals leads not only to enhanced diagnostic accuracy but also to streamlined workflow, contributing to better resource allocation and reduced costs.
Research Projects Utilizing AI Tools
Research projects employing AI tools exemplify the transformative role of these technologies in advancing biomedical science. A significant project involves the use of the DeepMind Health system for analyzing eye scans. This project has demonstrated that AI can accurately detect conditions like diabetic retinopathy at a level comparable to human experts. The implications of such findings could mean earlier detection and treatment, reducing the incidence of vision loss.
Another impactful research initiative is the collaboration between Google Health and healthcare institutions, which focuses on applying AI to predict patient deterioration. By analyzing electronic health records and various clinical data, the system can identify patterns that precede acute events. This predictive capability allows healthcare providers to take preemptive actions, thereby potentially saving lives.
Such AI-driven research projects highlight the value of interdisciplinary collaboration in science. They require input from data scientists, medical experts, and engineers to ensure that the models produced are clinically relevant and reliable. Their success speaks to the potential of AI in reshaping how research is conducted and how healthcare is delivered.
Interdisciplinary Collaboration in AI and Biomedical Engineering
Interdisciplinary collaboration is crucial in the field of AI and biomedical engineering. As these domains converge, they offer new insights and innovative solutions for complex healthcare challenges. The integration of diverse expertise fosters a comprehensive approach that enhances the effectiveness and efficiency of healthcare delivery.
In this context, collaboration between engineers and medical professionals stands out as essential. Engineers design algorithms and technologies, while medical professionals provide practical insights and clinical knowledge. This partnership ensures that the solutions developed are not only technically sound but also relevant to real-world medical problems. For instance, a surgical robot's design benefits greatly from input by surgeons who understand the nuances of specific procedures and patient needs.
Furthermore, interdisciplinary collaboration promotes the sharing of ideas and methodologies. By bridging the gaps between disciplines, teams can leverage collective knowledge for greater innovation. Engineers, for example, can learn about biological processes, while clinicians can understand advanced data analytics techniques. This shared understanding enhances the development of AI systems that can analyze medical images or predict patient outcomes with high accuracy.
The benefits of such collaboration are manifold. It leads to:
- Enhanced Innovation: Combining insights from different fields often leads to new ideas and approaches.
- Improved Patient Outcomes: Better-designed AI solutions can ultimately lead to more effective treatments and patient care.
- Holistic Understanding: A multidisciplinary approach encourages a comprehensive view of both technology and healthcare challenges.
Despite the obvious advantages, challenges remain in fostering effective collaboration. Cultivating mutual respect and understanding is critical. Often, engineers focus on technical aspects, while medical professionals may prioritize patient care. Finding common ground requires patience and persistence. Educational programs focusing on interdisciplinary learning can help bridge this gap and prepare future professionals for collaborative work.
In summary, interdisciplinary collaboration enhances AI's potential in biomedical engineering. By working together, engineers and medical professionals can create transformative solutions that improve healthcare outcomes. As the field evolves, embracing this collaborative spirit will be vital for continued success and innovation in the medical sector.
Partnerships between Engineers and Medical Professionals
Partnerships between engineers and medical professionals are pivotal in advancing AI applications in healthcare. Engineers bring technical knowledge about AI technologies, while medical professionals offer expertise on clinical needs and patient care.
The synergy between these two groups drives innovation. For example, engineers may develop predictive models for disease progression, but it's the insights from doctors that help refine these algorithms for practical use. This collaboration can lead to tools that are aligned with clinical workflows, making them easier for healthcare providers to implement.
Moreover, these partnerships facilitate hands-on training and education. Medical professionals can learn fundamental principles of AI, and engineers can gain insight into healthcare processes. This mutual learning results in a more integrated approach where technology solutions are tailored to the realities of clinical practice.
Role of Data Scientists in Biomedical Research
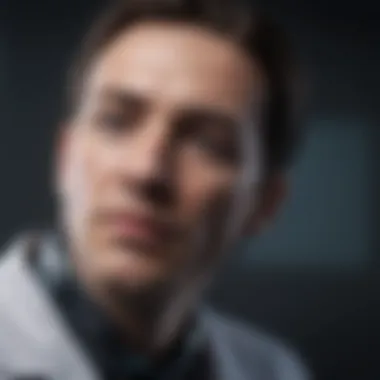
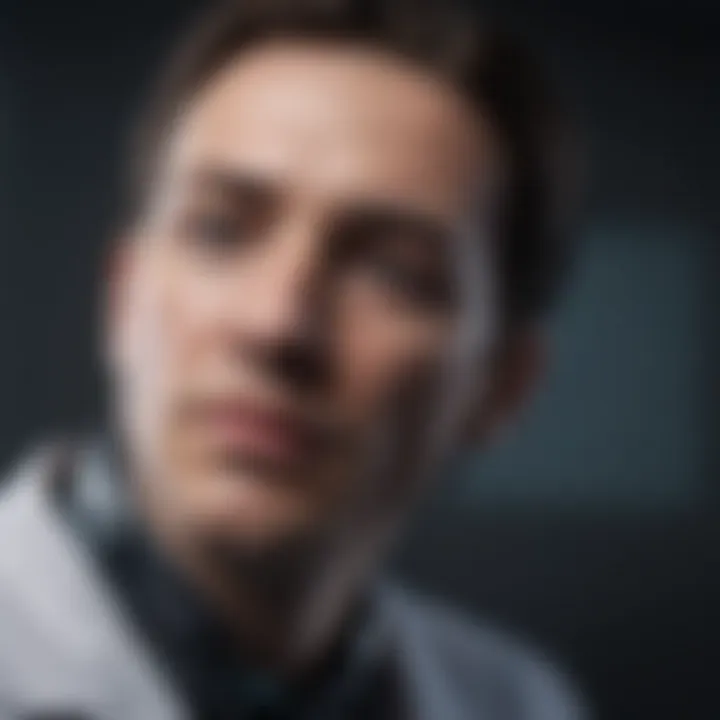
Data scientists play a significant role in biomedical research, acting as a bridge between raw data and actionable insights. They are skilled in extracting meaning from vast datasets, which is critical as biomedical research increasingly relies on data-driven methodologies.
In a healthcare setting, data scientists analyze diverse information, from patient records to genomic data, to identify patterns and correlations. This analysis can lead to breakthroughs in understanding disease mechanisms or optimizing treatment plans. By applying advanced techniques such as machine learning, data scientists enhance the predictive capabilities of AI systems in healthcare.
Furthermore, data scientists contribute to the design and implementation of AI models. They work closely with engineers to take theoretical algorithms and adapt them for real-world clinical data. This process is essential for ensuring that AI applications perform reliably and effectively when deployed in actual healthcare environments.
Ultimately, the role of data scientists is instrumental in transforming data into meaningful health insights, thus driving progress in biomedical research and improving patient outcomes.
Impact of AI on Patient Care
Artificial intelligence is transforming patient care by introducing advanced methods for improving health outcomes and enhancing the overall patient experience. The integration of AI technologies in healthcare settings provides numerous benefits. For instance, it helps in making more accurate diagnoses, selecting appropriate treatments, and monitoring patient conditions more effectively. Each of these components plays a vital role in defining how patients receive care today.
Improving Patient Outcomes
AI technologies have the capability to significantly elevate patient outcomes. By utilizing machine learning algorithms, medical professionals can analyze vast amounts of data to identify patterns that could be overlooked by human analysis alone. This results in more accurate diagnoses and tailored treatments. For example, predictive analytics can aid in detecting diseases at earlier stages, which is often critical for successful treatment.
- Utilizing AI in clinical workflows can streamline decision-making processes.
- Enhanced diagnostic accuracy reduces the likelihood of misdiagnosis.
- Real-time monitoring tools allow healthcare providers to adjust treatments promptly.
A remarkable example is IBM Watson, which assists oncologists in diagnosing cancer and suggesting treatment options based on a patient’s specific genetic profile. This personalized approach leads to better treatment plans and improved patient survival rates.
Enhancing Patient Experience with AI Tools
The integration of AI tools in healthcare environments also leads to an enhanced patient experience. Automated systems can simplify scheduling and follow-up processes, minimizing wait times and improving accessibility. Chatbots and virtual assistants provide patients with instant information, reducing anxiety about medical procedures.
Moreover, personalized communication through AI ensures that patient preferences are respected. This technology allows for tailored appointment reminders based on the individual’s routine, which enhances adherence to treatment plans.
- AI can assist in managing chronic conditions by providing regular health reminders.
- Patients can access medical information efficiently through AI-driven platforms.
- Tools such as telemedicine facilitated by AI software offer convenience and flexibility, especially for those in remote locations.
"AI is not merely a tool; it is a partner in enhancing patient care and outcomes."
"AI is not merely a tool; it is a partner in enhancing patient care and outcomes."
In summary, the impact of AI on patient care is profound. It not only offers the potential for improved clinical results but also redefines how patients interact with healthcare providers. This evolution, fostered by the application of AI technologies, leads to an overarching aim of better health for patients.
Global Perspectives on AI in Biomedical Engineering
Artificial intelligence (AI) is gaining traction globally, impacting various domains, including biomedical engineering. Understanding this influence is vital for grasping how healthcare systems evolve worldwide. The globalization of AI technologies fosters collaboration between researchers, healthcare providers, and technology developers. This interconnectedness offers diverse perspectives that enrich the field.
AI Initiatives Worldwide
Many countries are investing in AI initiatives to advance healthcare solutions. Research and development programs are being launched to harness AI's potential. For example, the United States has significant funding directed towards AI research in healthcare. Notable organizations, such as the National Institutes of Health, promote AI projects that focus on healthcare applications.
In Europe, initiatives like the European Health Data Space aim to integrate AI and data analysis for improving healthcare services. The European Union emphasizes ethical AI usage, shaping policies that enhance data security while maximizing AI benefits.
Asian countries, particularly China, demonstrate rapid progress in AI deployment within healthcare systems. Hospitals are utilizing AI for diagnostics and patient management. Furthermore, South Korea and Japan are developing AI safety protocols, reflecting their commitment to responsible AI integration in medical settings.
"Global initiatives in AI development are reshaping the landscape of biomedical engineering, enhancing patient care and research capabilities."
"Global initiatives in AI development are reshaping the landscape of biomedical engineering, enhancing patient care and research capabilities."
Comparative Analysis of AI Development in Different Regions
The development of AI in biomedical engineering varies significantly across regions. Each area has distinct strengths and challenges, influenced by local resources, regulatory frameworks, and healthcare systems.
- North America: This region scores highly in AI research funding and technological infrastructure. Companies like Google and IBM lead with investments in AI tools for healthcare, focusing on improving diagnostics and treatment planning. The regulatory environment is relatively conducive, allowing rapid innovation.
- Europe: The emphasis here is on ethical AI use and patient privacy. European nations are spearheading initiatives that focus on integrating AI into existing medical practices while ensuring compliance with stringent regulations. This cautious approach balances innovation with public trust.
- Asia: Here, the pace is varied. Countries like China lead in adoption, providing AI-enabled solutions at scale. However, others may face challenges due to infrastructure gaps. Japan and India are focusing on developing localized AI solutions to cater to their unique healthcare needs.
In summary, understanding global perspectives on AI in biomedical engineering is crucial. The differences in AI initiatives and their applications reveal the overall potential for the healthcare sector, highlighting the need for collaboration to leverage AI effectively.
Funding and Support for AI Research in Biomedicine
Funding for artificial intelligence research in biomedicine is crucial. It drives innovation and furthers our understanding of complex health issues. Financial resources enable the development of advanced AI technologies, which can lead to better diagnostic tools and treatment strategies. Given the potential of AI to revolutionize healthcare, support from both government and private sectors is necessary.
AI applications in biomedicine require significant investment. Academic institutions and private firms often rely on funding to conduct research. This can include exploring machine learning algorithms, developing software for image analysis, or testing AI in clinical settings. Without funding, these projects may struggle to take shape or progress.
Benefiting from robust funding can accelerate technological advancements. These developments can improve healthcare delivery by increasing efficiency in hospitals, enhancing patient care, and reducing costs. Alongside this, funding can also foster collaboration between researchers, engineers, and healthcare professionals.
Investing in AI research is not only about technology but also about improving lives and healthcare outcomes.
Investing in AI research is not only about technology but also about improving lives and healthcare outcomes.
Government and Private Sector Contributions
Government contributions play a vital role in funding AI research. Many countries recognize the importance of AI in healthcare and have initiated programs to support related projects. For example, in the United States, the National Institutes of Health (NIH) has been financially backing various AI research initiatives aimed at tackling health-related challenges.
Private sector contributions also complement government efforts. Companies such as Google and IBM have invested heavily in AI research to advance medical technology. These corporations often collaborate with academic institutions, creating valuable partnerships that lead to innovative solutions in healthcare.
Investment from both sectors enables researchers to explore new possibilities in AI applications, including:
- Developing predictive analytics for early disease detection.
- Enhancing robotic-assisted surgery through AI integration.
- Improving patient management with personalized treatment plans.
Grants and Funding Opportunities
Grants are an essential avenue for funding AI research in biomedicine. Numerous organizations offer dedicated grants to support projects in this area. Research institutions can apply for these grants to explore innovative solutions, develop prototypes, or initiate clinical trials. Grants often come from:
- Government bodies, like the NIH or the European Union.
- Private foundations that focus on health innovation.
- Non-profit organizations aiming to improve public health through technology.
Besides grants, funding opportunities also include venture capital investments and public-private partnerships. These can provide significant capital for companies developing AI solutions. Startups in the biomedical field can particularly benefit from these resources to advance their projects swiftly while ensuring practical applications.
In summary, funding and support for AI research in biomedicine are paramount. They enable the exploration of new technologies and strategies that potentially transform healthcare. Collaboration across sectors, through grants and contributions, is key to unlocking the full potential of AI in this domain.
Finale
The significance of the conclusion in this article cannot be overstated. It serves as a pivotal summary of the insights gathered throughout the discourse on artificial intelligence in biomedical engineering. The exploration has provided detailed views on the advancements, applications, and implications of AI technologies within healthcare and research sectors. Key aspects include not only the immediate benefits but also the challenges that will shape the future of medical practices.
AI stands out as a remarkable tool that enhances diagnostics, streamlines treatment planning, and assists in innovative research methodologies. As this article highlighted, various sources of data are now accessible, enabling powerful predictive analytics that nurture informed decision-making. However, the potential inherent in AI is accompanied by ethical considerations that require ongoing focus and scrutiny, particularly in matters of privacy and algorithmic bias.
Recap of Key Insights
In summary, the integration of AI within biomedical engineering presents vast opportunities:
- Enhanced Diagnostics: Image analysis and predictive analytics have transformed diagnosis accuracy, leading to better patient outcomes.
- Personalized Medicine: By leveraging AI algorithms, treatment plans can be finely tailored to meet individual patient needs effectively.
- Research Advancements: Data mining and machine learning foster groundbreaking research, crucial for understanding complex biological processes.
- Global Interactions: Initiatives around the world demonstrate varied approaches and implementations, showcasing the global movement toward AI integration in healthcare.
These key insights reflect the broader narrative of transformation fueled by AI technologies, emphasizing the collaboration between disciplines as essential for progress.
The Road Ahead for AI in Biomedical Engineering
Looking forward, several directions can be identified for the future of AI in biomedical engineering:
- Ongoing Innovation: Continuous advancements in AI algorithms and technologies promise to unlock further potential in improving patient care and operational efficacy.
- Regulatory Frameworks: The development of clear regulations will be necessary to guide ethical AI implementations, ensuring safety and fairness in healthcare applications.
- Interdisciplinary Collaboration: The increasing need for collaboration between engineers, medical professionals, and data scientists will drive innovative solutions in the field.
- Emphasis on Education: Preparing future professionals with knowledge in AI, data analysis, and biomedical engineering will be crucial for sustaining progress.
In summary, the road ahead is filled with both promise and responsibility. By understanding the implications and collaboration required, stakeholders can navigate the rapidly evolving landscape of AI in biomedical engineering effectively.