Harnessing AI's Potential in Life Sciences Innovations
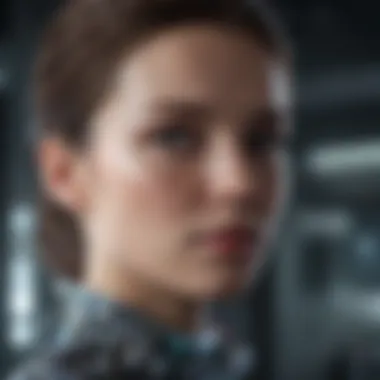
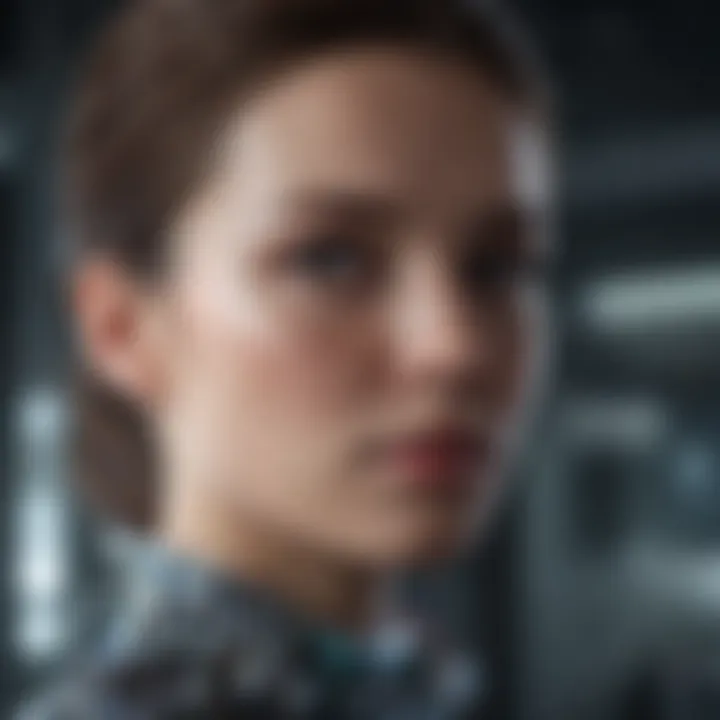
Intro
Artificial intelligence (AI) is reshaping various sectors, and the life sciences are no exception. Its growing influence is evident in many critical areas such as drug discovery, genomics, diagnostics, and personalized medicine. The intricacies involved in biological and clinical data are vastly expanded by AI methodologies. This potential not only accelerates research but also enhances outcomes in patient care and treatment efficacy.
The extent of AI's integration into life sciences demands a closer examination of its role. Understanding both its applications and implications is crucial for practitioners, researchers, and educators. This overview will guide the reader through the various facets of AI in life sciences, revealing how it can transform our approach to research and healthcare.
Article Overview
Purpose of the Article
This article serves to illuminate the transformative impact of AI in the life sciences. It examines AI’s multifaceted applications while addressing the challenges and ethical considerations that arise from its use. By analyzing both advancements and limitations, this piece aims to enrich the understanding of AI's potential and to underline the need for responsible implementation.
Relevance to Multiple Disciplines
AI's role in life sciences intertwines with several disciplines, making it relevant for a diverse audience. This includes:
- Biotechnology: AI optimizes processes in genetic engineering and biomanufacturing.
- Pharmaceuticals: Drug discovery processes benefit significantly from AI-enhanced predictive analysis.
- Healthcare: Enhanced diagnostics and treatment personalization improve patient care.
As AI tools advance, their influence on research trends and clinical outcomes continues to grow across various fields.
Research Background
Historical Context
The concept of AI dates back to the mid-20th century, but its practical applications gained traction in the 21st century. The convergence of big data, advanced algorithms, and computational power has accelerated AI development in life sciences, fostering innovative research techniques.
Key Concepts and Definitions
Understanding AI in this context requires familiarity with certain concepts:
- Machine Learning: A subset of AI where algorithms learn from data to improve predictive accuracy.
- Deep Learning: A more complex form of machine learning using neural networks, crucial for interpreting large datasets.
- Natural Language Processing: Enables machines to understand and generate human language, essential for analyzing scientific literature and clinical notes.
These definitions form the foundation for discussing AI's applications and implications in life sciences.
Intro to Artificial Intelligence in Life Sciences
Artificial Intelligence (AI) is becoming a crucial player in the life sciences. The integration of AI allows for advancements that were previously thought impossible. It enhances processes in drug discovery, genomics, diagnostics, and more. With its potential to process vast amounts of data, AI offers innovative solutions to complex problems. This article aims to establish the importance of this topic while highlighting its relevance across various disciplines.
The discussion of AI in life sciences addresses several specific elements. These include the efficiency AI brings to research and development, the depth of analysis it allows, and its ability to offer personalized solutions for patients. On the technical side, AI systems can analyze data patterns much quicker than humans, which significantly speeds up research timelines. Overall, the benefits of AI lie not only in enhancing scientific research but also in improving patient outcomes.
However, the deployment of AI in life sciences carries important considerations. Issues such as data privacy, ethical implications, and the need for accurate data interpretation must not be overlooked. Awareness of these issues is essential as AI continues to develop. The responsible application of AI technology is crucial to ensure that advancements in life science are beneficial for all stakeholders involved.
"AI is reshaping the landscape of life sciences, but challenges must be addressed to harness its full potential."
"AI is reshaping the landscape of life sciences, but challenges must be addressed to harness its full potential."
Applications of AI in Life Sciences
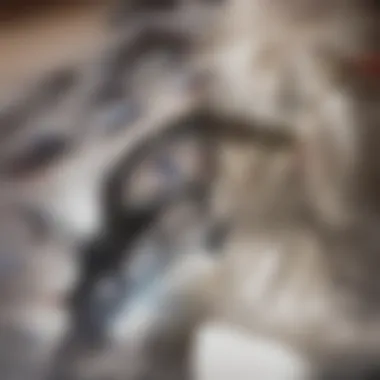
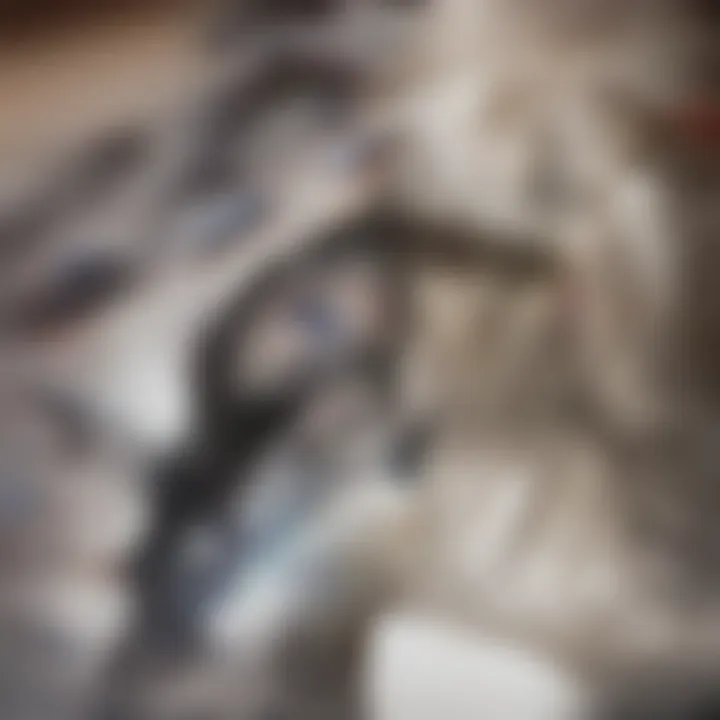
The integration of artificial intelligence in life sciences has become crucial in advancing various fields. AI technologies provide tools to analyze vast amounts of data quickly and accurately. As a result, they enable more informed decision-making and enhance the research process. This section delves into specific applications of AI, outlining its role in reshaping drug discovery, genomics, medical diagnostics, and personalized medicine.
Drug Discovery and Development
AI in Target Identification
Target identification is a fundamental step in the drug discovery process. AI can analyze biological data to pinpoint potential targets for new drug compounds. One key characteristic of AI in this context is its ability to process large data sets rapidly. This speed and efficiency make it an appealing choice for researchers aiming to enhance target discovery.
However, AI may face challenges such as reliance on high-quality data. Issues of data quality could lead to incorrect target identification, impacting drug development efforts.
Virtual Screening Techniques
Virtual screening is another application of AI in drug discovery. This method employs computational techniques to evaluate large libraries of compounds to identify promising candidates for further testing. The significance of virtual screening lies in its ability to reduce the time and cost involved in the hit identification phase. A unique feature of this approach is that it allows researchers to model interactions between compounds and biological targets effortlessly.
Despite its benefits, virtual screening can be limited by its reliance on pre-existing knowledge and models. This can occasionally lead to overlooking novel compounds that may have therapeutic potential.
Predictive Modeling in Clinical Trials
Predictive modeling uses AI to forecast outcomes in clinical trials, determining which factors are most likely to lead to success. It can analyze previous trial data to identify trends and potential pitfalls. This ability to synthesize historical data is a key characteristic of predictive modeling, making it a valuable asset in the design of clinical trials.
Nonetheless, the accuracy of predictive models is highly dependent on the quality of input data. Any inaccuracies can lead to misleading predictions, complicating the clinical trial process.
Genomics and Genetic Research
AI for Genome Sequencing
AI plays a pivotal role in genome sequencing, improving both speed and accuracy. By automating the analysis of sequencing data, AI allows researchers to focus on interpreting results rather than the labor-intensive aspects of sequencing. The rapid processing capability of AI is its standout feature, making it a popular choice in genomic research.
However, the dependence on AI tools raises concerns about whether they can fully capture the complexities of genomic data and the inherent biological variations.
Analysis of Genomic Data
The analysis of genomic data is enhanced by AI algorithms that can handle vast volumes of complex data efficiently. The unique feature here is AI's capacity to recognize patterns that may not be readily apparent to human researchers. This capability leads to profound insights, making AI invaluable in the study of genetic diseases.
Nonetheless, there are challenges in ensuring the quality and representativeness of the data being analyzed, which can influence the outcomes of AI analysis.
Identifying Genetic Variants
AI accelerates the identification of genetic variants by sifting through large-scale genomic data to highlight significant changes. The important characteristic here is the ability to distinguish meaningful genetic alterations from background noise. This precision is crucial for understanding disease mechanisms and tailoring treatments. However, the risk of generating false positives can occur, leading to potential misinterpretations of genetic data.
Medical Diagnostics
AI in Imaging Techniques
AI enhances imaging techniques, offering improved accuracy in interpreting medical images. It can quickly analyze X-rays, MRIs, and CT scans to detect anomalies that might be missed by the human eye. The critical advantage is the speed at which AI can process images, facilitating quicker diagnoses. However, the training data must be diverse and comprehensive; otherwise, biases can affect diagnostic accuracy.
Natural Language Processing for Patient Records
Natural Language Processing (NLP) leverages AI to extract and analyze information from unstructured patient records. This capability aids clinicians in making informed decisions based on comprehensive patient histories. The strength of NLP lies in its ability to transform narrative data into actionable insights. However, the limitations of NLP can come from variations in the way medical practitioners document information, which may hinder the accuracy of data extraction.
Early Detection of Diseases
AI systems have advanced the field of early disease detection significantly, allowing for quicker identification of conditions such as cancer. By examining patterns and changes in patient data, AI aids in recognizing early signs that may go unnoticed. The emphasis on proactive care through AI enhances patient outcomes. Nonetheless, over-reliance on these systems without thorough human validation can lead to misdiagnoses.
Personalized Medicine
Tailoring Treatments with Machine Learning
Machine learning algorithms can analyze patient data to develop personalized treatment plans. This approach takes into account a patient’s unique genetic makeup and health history. The primary advantage is the potential to improve treatment efficacy by targeting specific patient profiles. However, this method also faces challenges in data availability and potential privacy concerns.
AI in Patient Stratification
Stratifying patients based on their response to various treatments is another crucial application of AI. By analyzing vast datasets, AI can help identify which patient populations are most likely to benefit from specific therapies. The distinctive characteristic of this process is its precision in linking treatments to individual patient response. However, the potential for biased algorithms based on historical data must be carefully managed.
Monitoring Treatment Efficacy
AI supports ongoing monitoring of treatment efficacy by analyzing data from patients receiving care. This can lead to timely adjustments in treatment protocols depending on individual responses. The unique feature of this monitoring is its real-time feedback, improving patient management. However, maintaining patient engagement with these monitoring systems is necessary for successful implementation.
Challenges in Implementing AI in Life Sciences
The integration of Artificial Intelligence in life sciences brings significant opportunities for improving health outcomes and research efficiency. However, these benefits are coupled with notable challenges that can undermine the effectiveness and reliability of AI applications. This section explores critical challenges such as data privacy and security concerns, quality of data and bias issues, and the integration of AI with existing systems. Understanding these obstacles is essential as they can dictate the progress and adoption of AI technologies in this sector.
Data Privacy and Security Concerns
One of the foremost challenges in deploying AI within life sciences is safeguarding sensitive information. Health data, which often contains personally identifiable information, is subject to strict regulations like HIPAA in the United States. Violations can lead to severe consequences, including legal liabilities. When utilizing AI, organizations must ensure that their algorithms comply with privacy standards when processing patient data.
Additionally, the risk of data breaches poses a substantial concern. Hackers targeting healthcare institutions can lead to unauthorized access to sensitive patient records. This not only damages an organization's reputation but can also erode trust between patients and healthcare providers. As AI becomes more prevalent, there needs to be a robust framework to ensure data security, including encrypting data and employing secure cloud services.
Quality of Data and Bias Issues
The effectiveness of AI systems heavily relies on the quality of data they are trained on. If the data is incomplete, outdated, or not representative of the intended population, predictions can be misleading. In the context of life sciences, where nuanced factors can affect outcomes, this becomes particularly important. For example, if an AI model is trained primarily on data from one demographic group, it may perform poorly when applied to others, leading to unequal treatment recommendations.
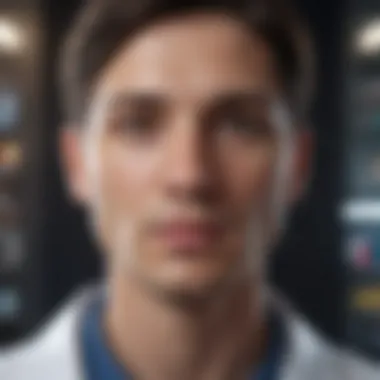
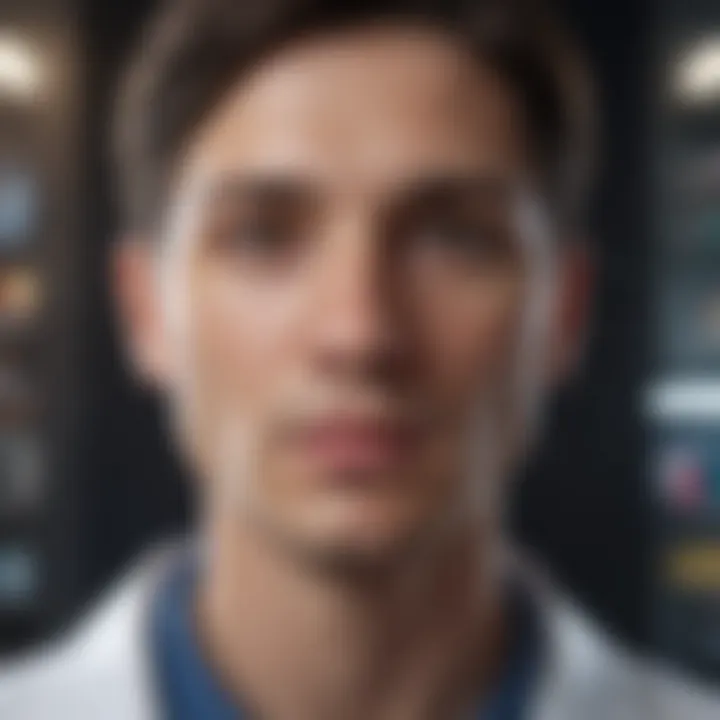
Furthermore, biases in datasets can propagate through AI algorithms, resulting in systematic disparities in healthcare. These biases can arise from various sources including historical inequalities in healthcare access and socio-economic disparities. To mitigate these risks, there must be a concerted effort to audit datasets for diversity and inclusiveness prior to any AI implementation.
Integration with Existing Systems
Successfully implementing AI also involves integrating new technologies with legacy systems already in place. Many healthcare organizations rely on established protocols and software that may not be compatible with AI solutions. This can create friction and delays in workflows, undermining potential benefits.
The integration process often requires significant resources and expertise. There may also be resistance from staff accustomed to conventional methods of operation. Staff training is essential to ensure that they are comfortable and proficient in using AI tools effectively. Organizations should consider creating a step-by-step implementation plan that allows for gradual integration, minimizing disruption and easing transition.
"Failing to address these challenges can result in wasted resources, ineffective treatments, and a potential loss of public trust in AI and healthcare institutions."
"Failing to address these challenges can result in wasted resources, ineffective treatments, and a potential loss of public trust in AI and healthcare institutions."
Ethical Considerations of AI in Life Sciences
The integration of artificial intelligence in life sciences brings various ethical considerations that cannot be overlooked. Understanding and addressing these concerns is vital for the responsible development and utilization of AI technologies in the sector. Key discussions revolve around the implications of AI on employment, accountability in decision-making processes, and the essential concepts of patient consent and autonomy. Each of these subheadings highlights the broader consequences of introducing AI in areas such as research, diagnostics, and treatment.
Impact on Employment in the Sector
The rise of AI technology inevitably affects job roles in the life sciences. While AI can enhance productivity, it also raises fears about job displacement. Traditional roles in laboratories, research, and even clinical settings may diminish due to automation and AI efficiencies.
However, new job categories are likely to emerge, focusing on the oversight and management of AI. For instance:
- AI Developers who specialize in creating sophisticated algorithms.
- Data Analysts to interpret results generated by AI systems.
- Regulatory Officials to ensure compliance with ethical standards.
Emphasizing the need for re-training and up-skilling will be crucial in harmonizing this transition. It’s not just about replacing roles but also about evolving them in harmony with technological advances.
Accountability and Decision-making
Accountability is a central ethical concern when AI systems make autonomous decisions. In biomedical research and diagnostics, decisions made by AI can have significant consequences for patient safety and treatment outcomes.
Consider the following factors:
- Transparency in AI Operation: Understanding how AI algorithms reach specific conclusions must be clear to stakeholders involved.
- Human Oversight: Decisions should be reviewed by healthcare professionals to ensure appropriateness and relevance.
- Responsibility: Defining who is accountable when an AI system fails or leads to adverse outcomes is critical.
AI must not only be a solution provider but must adhere to standards that prioritize human interests. Ensuring robust frameworks for accountability protects both practitioners and patients.
Patient Consent and Autonomy
Patient consent is a cornerstone of ethical medical practice. The introduction of AI in patient diagnostics and treatment raises questions about how informed consent is obtained and what it means for patient autonomy. This involves understanding how AI systems process personal health data and the implications it may have for privacy.
Key considerations include:
- Clear Communication: Patients should comprehend how AI impacts their care and what data is used.
- Informed Consent: Consent must be clearly documented, ensuring that patients understand the extent to which AI influences their treatment decisions.
- Respecting Autonomy: Patients should retain the right to make decisions regarding their treatment, even when an AI system provides recommendations.
The intersection of AI technology and patient care must be navigated carefully, balancing innovation with ethical standards to uphold the dignity and rights of individuals.
The ethical considerations surrounding AI in life sciences extend beyond technology, touching on vital human aspects such as respect for autonomy and the integrity of healthcare practices.
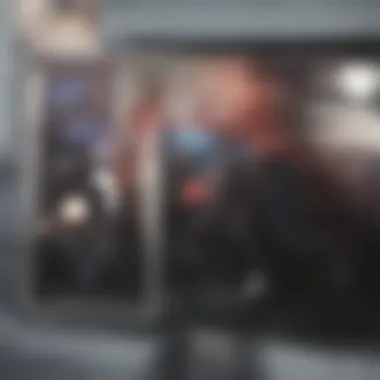
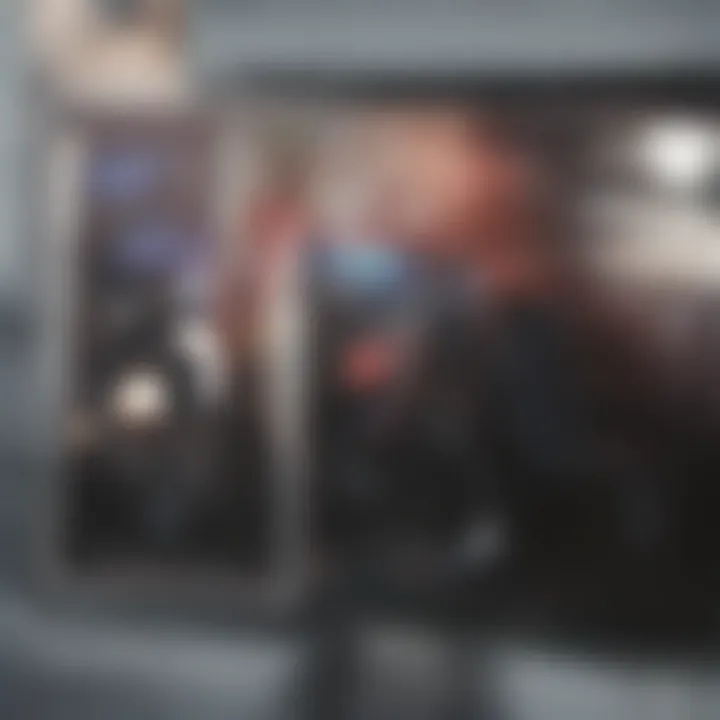
The ethical considerations surrounding AI in life sciences extend beyond technology, touching on vital human aspects such as respect for autonomy and the integrity of healthcare practices.
Future Trends of AI in Life Sciences
The integration of artificial intelligence into life sciences represents a pivotal shift in how research and clinical practices are approached. As technology continues to advance, the future of AI in this sector is filled with possibilities that could enhance discovery and improve patient outcomes. Understanding these future trends is crucial for stakeholders in the life sciences, including researchers, healthcare providers, and policymakers, as they strategize to harness AI's potential.
Emerging Technologies and Innovations
Deep Learning Advancements
Deep learning represents a significant aspect of artificial intelligence that leverages neural networks to analyze complex data. This technology has shown substantial contribution to areas such as image recognition, genomics, and predictive modeling. A key characteristic of deep learning is its ability to learn from vast amounts of data without specific programming, adapting its algorithms through exposure to new information.
One major benefit of deep learning advancements is their improved accuracy in diagnosing diseases from imaging data. For example, algorithms can now identify tumors in radiology scans with higher precision compared to traditional methods. However, reliance on deep learning can raise concerns about transparency in decision-making processes.
AI and Big Data Analytics
The fusion of artificial intelligence and big data analytics is revolutionizing life sciences research. By processing and analyzing large datasets, AI can uncover patterns that are not readily visible to human researchers. A notable characteristic of AI in this context is its capacity to perform real-time data analysis, effectively providing insights during clinical trials or research phases.
This approach enables significant advancements in personalized medicine. For instance, AI can analyze patient data to determine the most effective treatment plans based on similar cases. However, challenges still exist, such as ensuring data integrity and addressing issues of data bias, which can affect AI's recommendations.
Collaborative AI Platforms
Collaborative AI platforms represent a new way researchers and institutions can work together to tackle complex problems in life sciences. These platforms facilitate data sharing and collaborative model building, making research efforts more efficient. A key feature of collaborative AI is its ability to integrate diverse datasets and methodologies from various sources, enhancing the overall research output.
The advantages of using collaborative platforms include accelerated research timelines and the pooling of expertise. However, these platforms must address challenges such as data privacy and the differing regulations across geographical regions more comprehensively.
Interdisciplinary Approaches to Research
Embracing interdisciplinary approaches is essential for fostering innovation in life sciences. Merging insights from fields such as bioinformatics, computational biology, and even social sciences can lead to groundbreaking discoveries. In research, collaboration is becoming paramount. As AI tools evolve, they will increasingly support a cross-disciplinary environment, enhancing knowledge sharing and creative problem-solving.
By promoting these collaborative efforts, the life science community can adapt quickly to new findings and improve methodologies. Continuous learning and adaptation will define the landscape of future life sciences, powered by artificial intelligence.
Summary and Culmination
The integration of Artificial Intelligence within the life sciences is not just a trend; it marks a significant metamorphosis in how research and clinical practices are conducted. This section seeks to encapsulate the salient findings outlined in earlier parts of the article, providing a coherent framework for understanding AI's multifaceted role in life sciences.
Synthesis of Key Insights
From the examination of various AI applications, it is clear that we are witnessing a paradigm shift. Drug discovery processes are being expedited through algorithms that analyze vast datasets for potential therapeutic compounds. In genomics, the ability of AI to decipher complex genetic information enhances our understanding of diseases and contributes to personalized treatments. Moreover, AI's impact on medical diagnostics, particularly through imaging and patient records analysis, signifies its potential for early disease detection and improved patient outcomes.
Some of the central insights drawn from this exploration include:
- Accelerated Processes: AI reduces the time taken from discovery to market for new drugs, potentially saving valuable resources.
- Precision and Accuracy: Enhanced data analysis leads to better genomic insights, which in turn supports tailored treatment strategies.
- Improvement in Diagnostics: AI techniques can identify anomalies in medical imaging that are often overlooked by human eyes.
These insights delineate a future where AI becomes indispensable in life sciences, driving innovations that were unimaginable a few decades ago.
Recommendations for Future Research
Looking ahead, there are several avenues for future research that should be explored to maximize the benefits of AI in the life sciences:
- Addressing Ethical Challenges: As AI continues to permeate the field, efforts must be directed toward understanding and mitigating ethical dilemmas surrounding privacy, bias, and accountability.
- Data Integration Solutions: Future studies should focus on creating streamlined systems that integrate AI with existing technologies to enhance usability and functionality in diverse settings.
- Longitudinal Studies: There is a need for comprehensive long-term studies assessing the outcomes of AI interventions on patient health to validate effectiveness.
- Cross-Disciplinary Collaborations: Encouragement of partnerships among AI specialists, biologists, and clinicians to foster innovative solutions and ensure a holistic approach to utilizing AI in life sciences.
Future research in these areas can ensure that AI not only advances life sciences but does so responsibly, aligning with societal values and health care standards. The way forward is not merely about technological advancements; it is also about fostering an environment where these technologies serve humanity's broader goals.