Exploring the Current Status of Artificial Intelligence
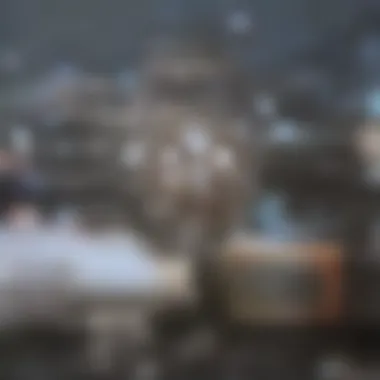
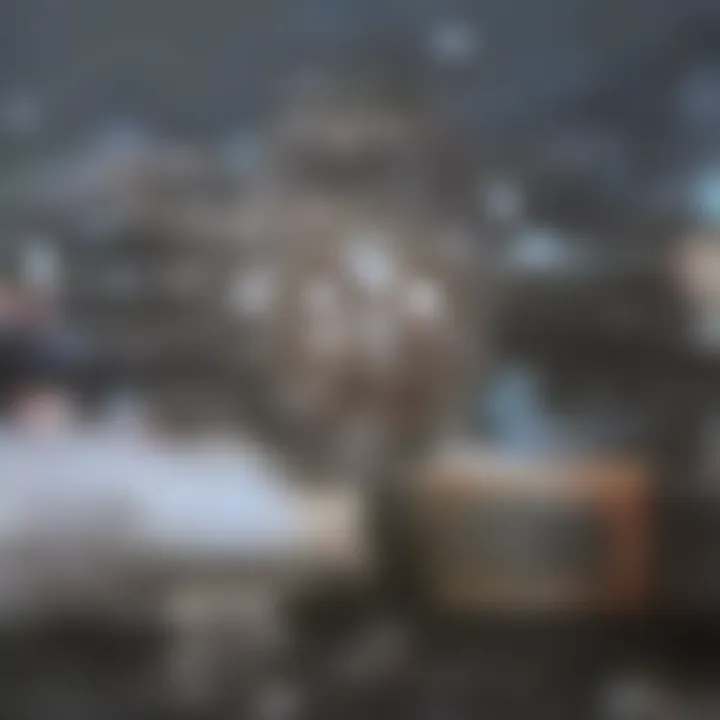
Intro
Artificial Intelligence (AI) stands at the forefront of technological evolution, transforming societies and industries. Its journey from conceptual theory to practical applications opens new avenues for both research and industry practices. The purpose of this article is to provide a comprehensive overview of the current landscape of AI, showcasing its advancements, exploring its diverse applications, and examining the ethical challenges it faces in various sectors.
Article Overview
Purpose of the Article
The purpose of this article is to clarify the multifaceted nature of AI today. It intends to inform readers—students, researchers, educators, and professionals—about the latest breakthroughs and real-world applications of AI technologies. Furthermore, the article sheds light on the implications of machine learning and deep learning within various contexts, fostering a critical understanding of their relevance and risks.
Relevance to Multiple Disciplines
Artificial intelligence does not exist in isolation. Its impact resonates across numerous domains such as healthcare, finance, education, and transportation. Understanding AI enables professionals in these fields to leverage its potential and address the associated ethical and practical challenges. This exploration aims to link AI's technological progress with its application, thus, it is relevant to practitioners and theorists alike.
Research Background
Historical Context
The concept of AI dates back to the mid-20th century, with pioneers like Alan Turing laying foundational ideas about machines mimicking human intelligence. Initially, early AI programs tackled simple tasks based on rule-based systems. As technology evolved, so did AI methodologies. This historical progression is vital as it underscores how far AI has come, and it sets the stage for contemporary advancements.
Key Concepts and Definitions
To discuss the status of AI effectively, clear definitions are essential. Understanding terms like machine learning, which refers to algorithms that improve automatically through experience, and deep learning, a subset of machine learning focused on neural networks, is crucial. These definitions frame the subsequent analysis and contextualize the various discussions on AI.
Preface to Artificial Intelligence
Artificial Intelligence plays a crucial role in today's technological landscape. Understanding AI is not just necessary for tech experts but also for professionals across various fields. Its impact spans various domains such as healthcare, finance, manufacturing, and education. The importance of AI derives from its capacity to analyze vast amounts of data, recognize patterns, and automate processes, leading to more efficient operations.
As AI technologies continue to advance, they change how businesses operate and interact. Organizations are increasingly relying on AI-driven solutions for decision making, enhancing customer experiences, and optimizing workflows. This section aims to provide insights into the fundamental concepts of AI and illuminate its historical context.
Definition and Scope of AI
Artificial Intelligence refers to the simulation of human intelligence in machines that are programmed to think and learn. The scope of AI encompasses a variety of subfields such as machine learning, natural language processing, robotics, and computer vision. These areas combine to enable machines to perform tasks that traditionally require human intelligence.
Key features of AI include:
- Learning: The ability to improve performance over time based on experience.
- Reasoning: The capacity to draw conclusions and make informed decisions.
- Problem-solving: The capability to address complex situations and overcome challenges.
AI can be divided into two broad categories: narrow AI and general AI. Narrow AI is designed to perform specific tasks, such as voice recognition or image classification. In contrast, general AI would possess human-like cognitive abilities across a wide range of tasks, a concept still largely theoretical.
History and Evolution of AI Technologies
The evolution of AI dates back to the mid-20th century. The term "artificial intelligence" was coined in 1956 during a conference at Dartmouth College. Early developments focused on symbolic reasoning and problem solving, leading to initial successes in game playing and mathematical problem solving.
Throughout the decades, several key milestones can be observed:
- 1960s – Early Programs: The creation of programs like ELIZA revolutionized natural language processing by simulating conversations.
- 1980s – Expert Systems: AI research expanded with expert systems that aided decision-making in specific fields, notably in medical diagnostics.
- 2000s – Machine Learning Resurgence: With access to large datasets and improved computational power, machine learning began to flourish, enabling AI systems to learn from data and enhance their performance.
Each phase of AI's history has contributed to the current sophistication of technologies available today. Overall, the development of artificial intelligence reflects a growing understanding of both the potentials and limitations inherent in replicating human cognition.
Current Trends in AI Research
The landscape of artificial intelligence is rapidly evolving. Understanding the current trends in AI research is important for grasping both the capabilities and limitations of these technologies. Researchers are not only optimizing existing algorithms but also exploring new avenues, making the field more dynamic than ever. This section highlights significant advancements and their implications for various sectors.
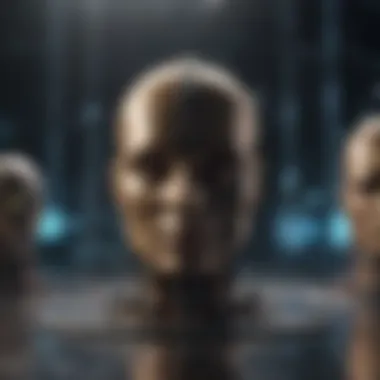
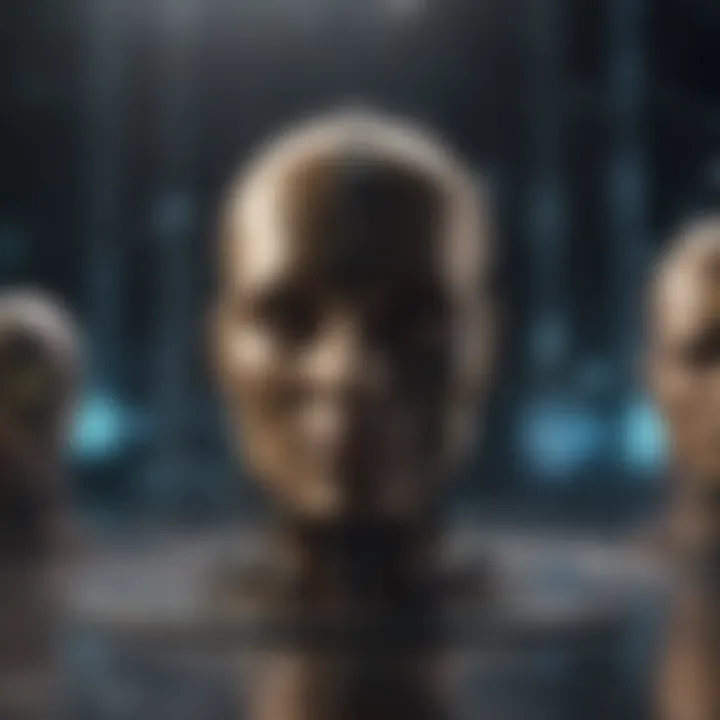
Advancements in Machine Learning
Machine learning has seen remarkable progress in recent years. These advancements enhance the ability of systems to learn from data without explicit programming. One key element is the introduction of algorithms that can process vast amounts of data efficiently. This efficiency allows for better predictions and decisions in various applications, from healthcare to finance.
Among the advancements, reinforcement learning has gained attention. In this method, agents learn optimal actions through trial and error, which is particularly useful in robotics and game AI. Furthermore, the integration of machine learning frameworks like TensorFlow and PyTorch have simplified model building, making it more accessible for researchers.
Another important aspect is the emergence of automated machine learning (AutoML). This trend enables users with limited expertise to develop predictive models. AutoML tools automatically select the best algorithms and tune parameters, hence democratizing access to machine learning capabilities.
Deep Learning Breakthroughs
Deep learning, a subset of machine learning, drives many leading innovations today. It relies on neural networks with many layers, thereby enabling the processing of complex data structures. Breakthroughs in this area have fueled advancements in image recognition, speech recognition, and various other domains.
Notably, convolutional neural networks (CNNs) have achieved outstanding results in computer vision tasks. Systems can now analyze images with accuracy comparable to, or sometimes better than, humans. Likewise, recurrent neural networks (RNNs) have transformed how we visualize and interpret sequences, enhancing natural language processing.
These breakthroughs have led to practical applications, such as self-driving cars and advanced medical imaging. The implications for industry and society are profound, as these technologies continue to reshape our world.
Natural Language Processing Innovations
Natural language processing (NLP) has undergone significant transformations. Recent innovations have improved how machines understand human language, bridging the gap between human communication and computer systems. These advancements have resulted in more sophisticated chatbots, translation services, and voice-activated assistants.
One of the major trends in NLP is the development of transformer models. They outperform traditional models in various tasks by processing text in parallel rather than sequentially. This allows for a better understanding of context and nuance in language.
Notable implementations include OpenAI's GPT-3 and Google's BERT. These models have raised the standard for what is achievable in NLP. They effectively generate human-like text and comprehend subtle meanings.
In sum, the current trends in AI research not only demonstrate the advancements in technology but also raise essential questions about ethical use and societal impact. By examining these developments, we can appreciate how far AI has come and contemplate its future trajectory.
Applications of AI Across Industries
Artificial Intelligence has transcended its early experimental phases and now finds itself interwoven in various sectors. The application of AI technologies enables industries to enhance efficiency, optimize operations, and even revolutionize customer experiences. Understanding these applications is crucial, as they not only reflect the current state of AI but also determine the trajectory of future advancements and business strategies.
Healthcare and Medical AI
AI is becoming increasingly vital in the healthcare sector. It allows for improved diagnostics, personalized treatment plans, and operational efficiency.
- Diagnostic Tools: AI algorithms, especially those utilizing machine learning, can analyze medical images with high accuracy. For instance, Radiology employs AI tools to detect anomalies in X-rays and MRIs far quicker than human radiologists. This can lead to faster diagnoses and, hence, better patient outcomes.
- Predictive Analytics: Anticipating health risks through predictive analytics is another stronghold for AI. By assessing vast amounts of patient data, AI can recognize patterns that predict disease outbreaks or individual patient incidents, allowing for preemptive care strategies.
- Drug Discovery: AI aids pharmaceutical companies in drug discovery processes by simulating drug interactions and predicting outcomes. This accelerates the development timeline and decreases costs significantly.
AI in Financial Services
In the financial sector, AI drives efficacy and security, making transactions smoother and fraud detection strong.
- Automated Trading: Investment firms utilize algorithms to analyze market trends and execute trades at speeds unattainable by human traders. This contributes not only to increased profits but also to market stability.
- Risk Management: AI tools assess credit risks and predict defaults by processing large data sets on user behavior and other variables. This enables banks to make more informed lending decisions.
- Fraud Detection: The employment of AI in monitoring transactions helps in swiftly identifying fraudulent activities. Algorithms can flag unusual patterns instantly, safeguarding user accounts and assets.
AI in Manufacturing and Robotics
Manufacturing has witnessed a drastic transformation due to AI integration in robotics and process automation.
- Process Optimization: AI systems analyze production data to identify inefficiencies. Through predictive maintenance, they reduce downtime by ensuring that machines are serviced proactively rather than reactively.
- Quality Control: Machine learning models process images from product lines to detect defects that the human eye might miss. This enhances overall product quality and customer satisfaction.
- Supply Chain Management: AI tools facilitate better forecasting and inventory management, leading to reduced costs and optimized logistics within supply chains.
AI's Role in Education and Learning
The education sector is harnessing AI to create personalized learning experiences and administrative efficiencies.
- Personalized Learning: AI platforms analyze student performance data to tailor educational content according to individual learning speeds and styles. This fosters better engagement and improved educational outcomes.
- Administrative Automation: AI streamlines administrative tasks, including grading and attendance tracking, allowing educators to focus more on teaching.
- Virtual Learning Environments: Advanced AI systems power virtual classrooms, enhancing remote learning experiences and accessibility.
AI in Transportation and Autonomous Vehicles
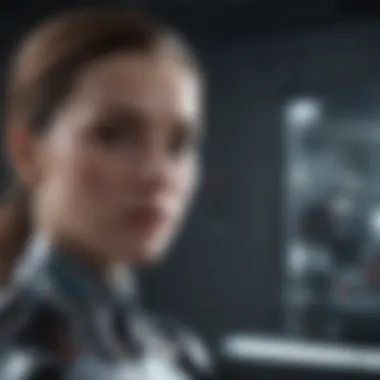
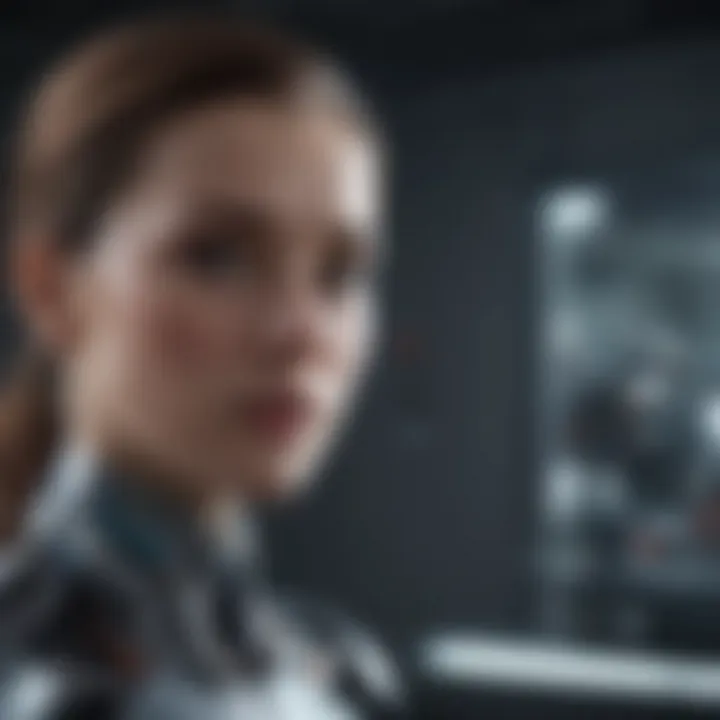
Transportation is another domain undergoing significant change due to AI applications. The rise of autonomous vehicles represents a significant achievement in this sector.
- Self-Driving Cars: AI algorithms in self-driving cars process real-time data from sensors to navigate safely, making transportation more efficient and reducing accidents.
- Traffic Management: AI analyzes traffic patterns and optimizes traffic signals in real-time, which can substantially reduce congestion in urban areas.
- Logistics Optimization: AI systems optimize routing in freight and delivery services, leading to reduced operational costs and timely delivery.
The integration of AI across these diverse industries underscores its transformative impact on operational paradigms and service delivery. As these technologies continue to evolve, they promise to redefine traditional practices, enhancing performance and efficiency at all levels.
The integration of AI across these diverse industries underscores its transformative impact on operational paradigms and service delivery. As these technologies continue to evolve, they promise to redefine traditional practices, enhancing performance and efficiency at all levels.
Ethical and Societal Implications
The ethical and societal implications of artificial intelligence are critical in understanding how these technologies affect various aspects of life. As AI systems become more widely adopted, concerns surrounding bias, privacy, and employment are increasingly at the forefront of discussions within academic and professional communities. Addressing these implications is essential for ensuring that AI technology is developed and used responsibly.
Bias and Fairness in AI Algorithms
Bias in AI algorithms can lead to unfair and discriminatory outcomes. When AI systems are trained on data that reflects societal biases, they can perpetuate or even exacerbate these biases in real-world applications. This issue is particularly pronounced in sectors such as hiring, criminal justice, and healthcare, where AI systems are often used to make consequential decisions.
For instance, a recruitment AI might favor candidates from certain demographic backgrounds if its training data is primarily sourced from those demographics. Correcting bias and promoting fairness necessitates diverse data sets, transparency in algorithms, and regular audits of AI outcomes. More efforts are needed to identify and mitigate bias at every stage of AI development.
Data Privacy Concerns
Data privacy is another pressing ethical issue. AI requires vast amounts of data to learn and function effectively. This often includes personal information from individuals. The potential for data breaches hights the risks associated with privacy. Users may not fully understand how their data is being collected, used, or shared.
Regulations such as the General Data Protection Regulation (GDPR) in Europe aim to safeguard individual privacy rights. However, compliance is challenging, especially for smaller companies. It is crucial for organizations to establish transparent data practices and obtain consent from users. Ensuring data protection requires both technical measures and clear communication with users about their rights and the use of their data.
AI and Employment Dynamics
The relationship between AI and employment is multifaceted and often contentious. While AI has the potential to automate tasks, increase efficiency, and create new job opportunities, it also poses risks of job displacement. The sectors most vulnerable include manufacturing, transportation, and administrative roles, where automation can replace human labor.
On the other hand, AI also creates demands for new skills, fostering jobs in technology, AI ethics, and data analysis. Thus, it is important to focus on retraining and reskilling workers to adapt to the changing job market. Education systems must evolve to equip students with necessary skills that align with future job demands. The challenge is to balance technological advancement with the socio-economic impacts on workers.
Addressing the ethical considerations in AI is not merely a technical challenge; it requires a collective societal effort to shape regulations, practices, and education.
Addressing the ethical considerations in AI is not merely a technical challenge; it requires a collective societal effort to shape regulations, practices, and education.
Regulatory and Governance Challenges
The growth of artificial intelligence technology brings both opportunities and challenges. Regulatory and governance structures are essential to address these issues effectively. As AI becomes increasingly integrated into society, a framework for regulation helps ensure that developments in AI align with ethical norms and societal values. Often, lack of regulation can lead to risks, making governance a central focus for stakeholders.
The importance of understanding regulatory and governance challenges lies in their potential to mediate conflicting interests. Balancing innovation with ethical considerations is not straightforward. Effective governance can protect users while fostering an environment conducive to technological advancement. Without such frameworks, the unbridled progress of AI could have unintended negative consequences.
Current Regulatory Frameworks
Currently, regulatory frameworks for artificial intelligence vary across jurisdictions. In some regions, specific laws are developing, while in others, existing laws are being adapted to cover AI. For instance, the General Data Protection Regulation (GDPR) in the European Union sets clear guidelines for data use, which is critical for AI systems that rely on vast amounts of data.
In the US, the approach to AI regulation is less centralized, often relying on sector-specific guidelines. The Federal Trade Commission (FTC) has provided some recommendations concerning AI and data practices.
Key elements of existing frameworks include:
- Data Protection: Ensuring data privacy and security.
- Accountability: Defining who is responsible for AI-driven decisions.
- Transparency: Making algorithms understandable to users and stakeholders.
These components are crucial in fostering trust between technology providers and users, allowing for informed consent and ethical usage of AI systems.
International Cooperation in AI Legislation
International cooperation is becoming increasingly important in the realm of AI regulation. As AI technologies transcend borders, harmonizing regulations across different nations can enhance both security and efficiency. Countries are beginning to realize that unilateral regulations may not be effective in handling the global nature of AI.
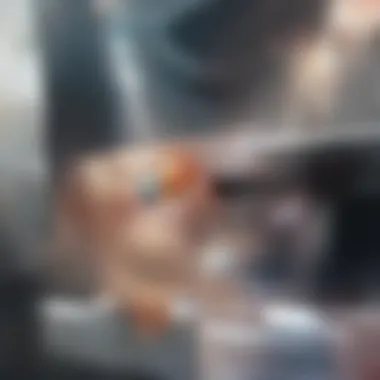
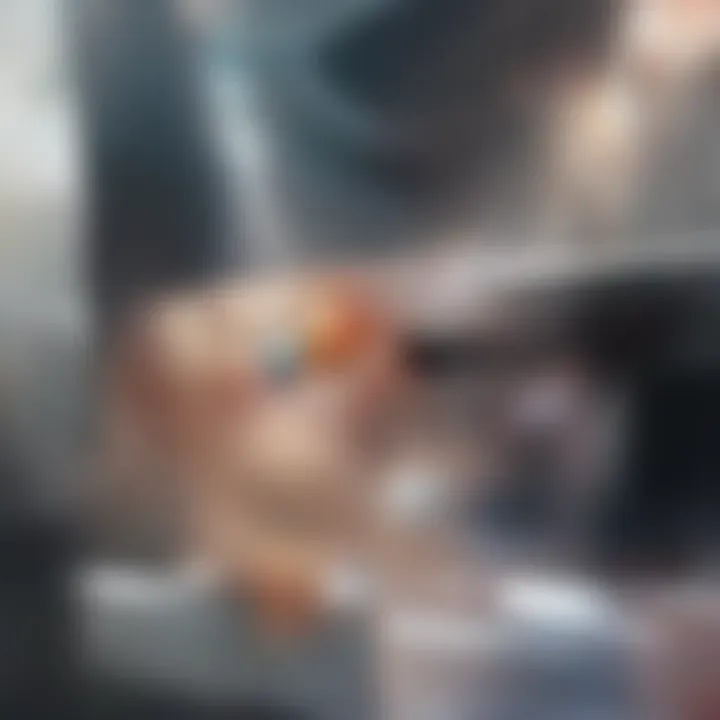
The development of common standards can facilitate collaboration while mitigating risks associated with divergent regulations. Initiatives such as the OECD’s AI Principles emphasize the need for global cooperation. These principles advocate for:
- Inclusivity: Engaging a diverse range of stakeholders in the legislative process.
- Shared Responsibility: Encouraging nations to work together on AI governance.
- Ethical Considerations: Focusing on human-centered AI development.
By collaborating, nations can establish frameworks that not only address local needs but also promote interoperability and innovation on a global scale.
"Effective governance of AI requires collective action. No single nation can address the challenges posed by AI in isolation."
"Effective governance of AI requires collective action. No single nation can address the challenges posed by AI in isolation."
In summary, as the AI landscape evolves, regulatory frameworks and international cooperation become increasingly vital. These developments are crucial to navigate the complexities of AI, ensure ethical deployment, and protect societal interests. Understanding these challenges allows stakeholders to engage proactively, shaping a future where technology serves humanity.
Future Directions in AI Development
The exploration of future directions in artificial intelligence holds a critical place in discussing its current status. As AI technologies continue to evolve, understanding the trajectory of these advancements is essential for students, researchers, educators, and professionals. This section examines pivotal aspects such as the integration of quantum computing, human-machine collaboration, and predictions for the upcoming decade, all while considering the implications these advancements might have on various industries.
The Role of Quantum Computing in AI
Quantum computing is emerging as a transformative force for artificial intelligence. Traditional computers process data in bits, either 0 or 1. In contrast, quantum computers utilize qubits, which can represent and process data in multiple states simultaneously. This capability allows quantum computing to handle complex calculations at unprecedented speeds.
The intersection of quantum computing and AI is noteworthy for several reasons:
- Speed: Quantum computing can significantly accelerate machine learning processes. This acceleration means that AI systems can learn from vast amounts of data more efficiently.
- New Algorithms: Quantum algorithms can solve problems that are currently intractable for classical computers. This advancement opens doors to new applications in various fields including cryptography, optimization, and drug discovery.
- Enhanced Data Processing: AI algorithms can leverage quantum computing to analyze larger datasets effectively, which can lead to improved patterns recognition and predictive capabilities.
"+ Quantum computing has the potential to revolutionize everything from healthcare to transportation by enabling AI to operate at levels previously unimaginable."
"+ Quantum computing has the potential to revolutionize everything from healthcare to transportation by enabling AI to operate at levels previously unimaginable."
AI and Human-Machine Collaboration
The collaboration between humans and machines is a growing area of interest in the field of artificial intelligence. This partnership is changing how various tasks are executed and can enhance productivity across multiple sectors.
Key elements of this collaboration include:
- Augmented Decision-Making: AI can process vast amounts of information to support human decision-making. For instance, in healthcare, AI assists doctors by analyzing patient data to propose treatment options.
- Task Automation: While AI automates repetitive tasks, it allows humans to focus on more creative and strategic aspects of their jobs. This synergy can lead to greater job satisfaction and innovation.
- Feedback Loops: When humans and AI systems work together, they create a feedback loop. This means that as AI systems learn from human input, they continuously improve, leading to higher quality outcomes.
Predictions for AI in the Next Decade
Looking ahead, several predictions emerge regarding the advancement of artificial intelligence in the next decade. Several trends are likely to shape the landscape:
- Increased Integration in Daily Life: Expect AI to become more embedded in everyday situations, from personal assistants like Amazon Alexa to AI-driven household appliances.
- Advancements in Ethics and Governance: With AI's growth comes the need for stricter regulations. Ethical considerations will take center stage as societies grapple with the implications of AI in privacy, surveillance, and consent.
- Continued Investment: Billions are projected to flow into AI research, propelling innovation and development of new applications and tools.
- Workforce Transformation: Job roles will evolve as AI automation becomes prevalent. Employees will need to adapt to a changing job market, emphasizing the importance of reskilling and lifelong learning.
The End and Final Thoughts
The attribute of adaptability in AI systems underscores the necessity for continual adjustment in regulations and governance. As AI technology evolves, its potential for misuse or biased outcomes must be critically assessed. Thus, our understanding of AI's current landscape serves as a basis for future investigations and policy-making.
"AI is not just a tool; it reshapes how we approach problems and work together."
"AI is not just a tool; it reshapes how we approach problems and work together."
Summary of Key Insights
From this exploration of artificial intelligence, several key insights emerge:
- Advancements in AI Technologies: The pace of innovation in machine learning, deep learning, and natural language processing is accelerating rapidly.
- Applications Across Sectors: Industries like healthcare, finance, and education are integrating AI to improve accuracy and efficiency, showcasing transformative capabilities.
- Ethical Implications: Issues such as bias in algorithms and data privacy are critical considerations when discussing the societal impact of AI.
- Future Directions: The role of quantum computing and deep collaborations between humans and machines seems poised to redefine our understanding of intelligence itself.
Implications for Future Research
Future research in artificial intelligence is essential for several reasons:
- Ethical Frameworks: There is a dire need for robust ethical guidelines that can adapt to the evolving nature of AI technologies. Emerging frameworks must address bias, transparency, and accountability at all levels of AI deployment.
- Interdisciplinary Studies: The interplay between AI and other domains such as psychology, economics, and ethics should be a focus. This can illuminate the multidimensional challenges posed by AI systems.
- Technological Advancements: Continued exploration of technologies like quantum computing and blockchain can enhance the capabilities of AI. Researchers need to investigate how these technologies can lead to breakthroughs in AI applications.
- Societal Impact: Understanding AI’s effect on employment, education, and daily life is paramount. Research should aim to quantify these impacts and propose solutions to mitigate negative consequences.