A Deep Dive into Omics Data Analysis Techniques
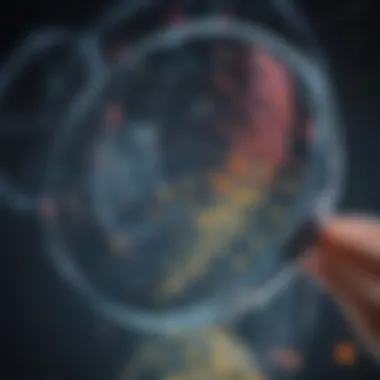
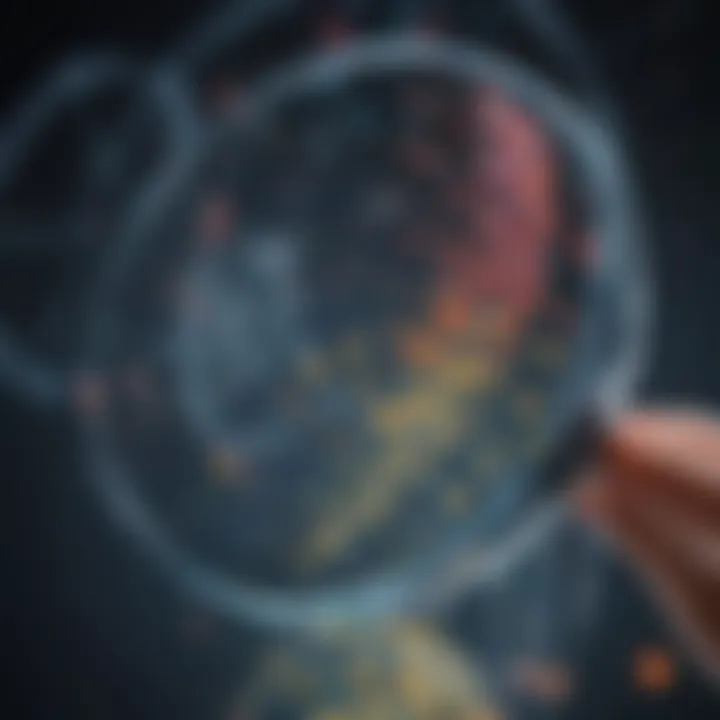
Intro
In recent decades, omics data analysis has morphed into a cornerstone of biological research, steering our understanding of complex biological systems. This growing discipline offers powerful insights across numerous fields including genomics, proteomics, metabolomics, and transcriptomics. As research in these areas expands, so does the volume of data produced, drowning us in a sea of information. To navigate this, experts employ a variety of analytical techniques and frameworks that can accommodate the intricacies of biological data.
The implementation of omics technologies and methodologies allows researchers to investigate biological questions with unprecedented granularity. This exploration doesn't just cater to scientists—it impacts educators, healthcare professionals, and anyone intrigued by life sciences, emphasizing the necessity for interdisciplinary collaboration. Here, we will fetch a closer examination of the structure and relevance of this timely subject, dissecting it to provide a clearer picture of its significance in advancing knowledge and personalizing medicine.
Article Overview
Purpose of the Article
This piece aims to unravel the complexities involved in omics data analysis. By dissecting various methodologies and tools used in the field, we hope to present a guide that is both practical and informative. The intent is to clarify the inherent challenges researchers face, while also spotlighting innovative techniques that are steadily reshaping the landscape of biological inquiry.
Relevance to Multiple Disciplines
Omics research is not just the concern of geneticists or medical practitioners; it bridges a variety of disciplines. Here are a few areas where omics data analysis plays a significant role:
- Medical Research: Enhancing personalized treatment plans for individuals.
- Agricultural Science: Improving crop resilience and yield through genomic insights.
- Environmental Studies: Understanding ecological impacts and biodiversity.
Through this article, we dissect the relevance across these domains, not merely as a passing interest, but as a necessity for future advancements.
Research Background
Historical Context
The inception of omics research began in the late 20th century, primarily focused on genomics—the comprehensive study of an organism's entire set of genes. The dopamine of discovery that accompanied the Human Genome Project in the 1990s kicked the field into high gear. Over the years, this foundational work opened doors to the burgeoning fields of proteomics, transcriptomics, and metabolomics, paving paths to untold insights.
Key Concepts and Definitions
To fully grasp the scope of omics data analysis, it is important to clarify some key terms:
- Genomics: The study of the structure, function, evolution, and mapping of genomes.
- Proteomics: Focused on the large-scale study of proteins, particularly their functions and structures.
- Transcriptomics: Involves the study of the transcriptome, the complete set of RNA transcripts produced by the genome at any one time.
- Metabolomics: The study of the set of metabolites in an organism, reflecting its phenotypic expression.
Together, these areas of study encompass the complete biology of an organism, highlighting the need for integrated analysis.
Omics data is not merely a collection of isolated studies; it is a woven fabric that displays the interdependence of genetic, protein-based, and metabolic networks in living systems.
Omics data is not merely a collection of isolated studies; it is a woven fabric that displays the interdependence of genetic, protein-based, and metabolic networks in living systems.
Each layer offers insights that are crucial in understanding the wider context of life sciences, guiding research advances that are applicable in, say, cancer treatment or disease prevention, making it clear that omics isn't just a trend; it's a revolution.
Prologue to Omics Data Analysis
Omics data analysis has become a cornerstone of modern biological research, profoundly influencing various scientific fields. With its roots in genomics, proteomics, transcriptomics, and metabolomics, this multifaceted discipline offers the tools needed to decode complex biological systems. By integrating large-scale datasets, researchers are empowered to unveil patterns and interactions that were once elusive. Thus, delving into omics data analysis is not merely an academic pursuit; it is an essential step toward understanding life at a molecular level.
The relevance of omics data analysis in today's research landscape cannot be overstated. As technology has advanced, the amount of data generated has surged, making sophisticated analytical techniques indispensable. The insights drawn guide critical discoveries in disease mechanisms, therapeutic interventions, and even advancements in personalized medicine. In an era where targeted treatments and preventive strategies are on the rise, navigating the world of omics becomes increasingly vital.
Definition and Scope
Omics data analysis encompasses the systematic study of various biological molecules, aiming to understand their roles, interactions, and functions within greater biological contexts. The term "omics" refers to the comprehensive analysis of these large-scale datasets, typically produced by high-throughput techniques. In many cases, it is segmented into distinct fields:
- Genomics focuses on the comprehensive study of the genome, providing insights into genetic variation and expression.
- Proteomics examines the entire set of proteins, revealing their functions, structures, and interactions.
- Transcriptomics involves the study of RNA molecules, offering valuable information on gene expression patterns.
- Metabolomics delves into the metabolic profiles, elucidating biochemical pathways and cellular processes.
Understanding these distinctions is crucial, as they collectively contribute to the overall picture of biological functioning. Each omic layer provides context that informs research hypotheses and experimental designs.
Historical Context
The journey of omics data analysis has been shaped by technological advancements and theoretical breakthroughs. It all began with the Human Genome Project in the late 20th century, an ambitious endeavor that aimed to map the entire human genome. This initiative marked a significant shift in biological research, laying the groundwork for genomics as a recognized discipline.
Following the genome project, researchers turned their attention to other omic fields. Proteomics emerged in the late 1990s when innovations in mass spectrometry enabled researchers to analyze complex protein mixtures. Then came transcriptomics in the early 2000s, utilizing microarray technology to quantify gene expression. As these technologies evolved, so too did analytical methods, giving rise to bioinformatics—a critical area that combines computer science with biological data.
Today, the interplay of these fields continues to yield revolutionary insights. Omics data analysis has moved from being a niche focus to a foundational element of biomedicine and health research. As we look to the future, we find ourselves at the forefront of integrating these vast datasets, leveraging new methodologies, and addressing the challenges that lie ahead.
Types of Omics
Omics data analysis is a broad field, encompassing a multitude of disciplines that focus on different biological molecules. Understanding the various types of omics is vital for researchers and practitioners alike as it lays the groundwork for advanced studies and applications in genetics, medicine, and agriculture. This section will examine the distinct categories: genomics, proteomics, transcriptomics, and metabolomics, elaborating on their significance and implications in today’s scientific landscape.
Genomics Overview
Genomics refers to the study of an organism's entire genome, including its structure, function, evolution, and mapping. With the advent of high-throughput sequencing technologies, genomics has become a cornerstone of biological research. It allows scientists to delve into genetic variations, uncover mutations linked to diseases, and explore hereditary traits. Learning about genomics is crucial for many reasons:
- Disease Associations: By identifying genetic markers, researchers can link certain genes to diseases like cancer, diabetes, and heart conditions.
- Understanding Evolution: Genomics sheds light on evolutionary relationships between species, providing insights into how they adapt to their environments.
- Agricultural Improvements: Genetic modification and selective breeding have been enhanced through genomic studies, leading to crops with better yields and disease resistance.
Proteomics Essentials
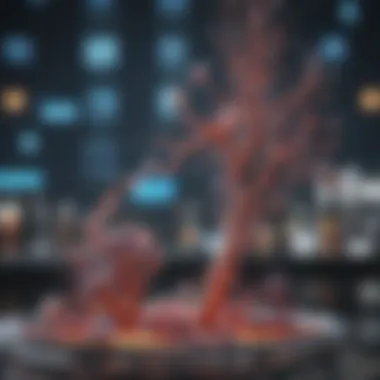
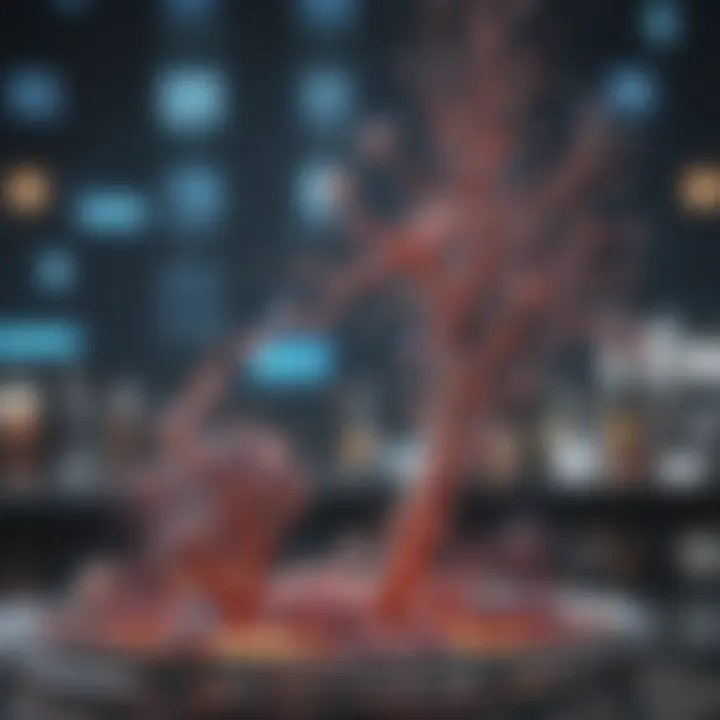
Proteomics is the large-scale study of proteins, which are vital for essentially every biological function. The field has blossomed due to advances in mass spectrometry and bioinformatics. Understanding proteomics has several key elements:
- Protein Functions: By analyzing protein expression and modification, researchers can gain insights into cellular processes and signaling pathways.
- Biomarker Discovery: Proteomics is instrumental in identifying biomarkers for diseases, which can aid in earlier diagnosis and targeted therapy.
- Drug Development: The interaction between drugs and proteins is fundamental in pharmacology, and proteomic studies facilitate the discovery of new therapeutic targets.
Transcriptomics Insights
Transcriptomics focuses on the transcriptome, the complete set of RNA transcripts produced by the genome under specific circumstances. This discipline plays a significant role in understanding gene expression patterns:
- Gene Regulation: Transcriptomic analyses reveal how genes are turned on and off in response to various environmental stimuli or developmental processes.
- Disease Mechanisms: By studying transcriptomic changes in disease states, researchers can identify potential therapeutic targets and comprehend disease mechanisms more deeply.
- Comparative Studies: It enables comparisons between different tissues or conditions, illuminating how transcriptional variation influences biological outcomes.
Metabolomics Fundamentals
Metabolomics examines the complete set of metabolites within a biological sample, providing a holistic view of metabolic activities. This branch of omics is crucial because:
- Metabolic Profiling: It allows scientists to profile metabolic alterations in response to drugs, diseases, or nutritional changes, offering vital information for personalized medicine.
- Biomarker Identification: Similar to proteomics, metabolomics can identify unique metabolic signatures that are indicative of specific diseases.
- Systems Biology: Integrating metabolomics with other omics data helps create a comprehensive picture of biological processes, enhancing our understanding of life's complexities.
"Omics approaches involve comprehensive assessment of biological systems that can provide a window into the intricate machinery of life."
"Omics approaches involve comprehensive assessment of biological systems that can provide a window into the intricate machinery of life."
Data Acquisition Techniques
In the realm of omics data analysis, acquiring robust and reliable data is the very backbone of any successful investigation. Without a solid foundation in data acquisition, you might as well be building a castle on sand. Each type of omics data—whether it be genomics, proteomics, or metabolomics—requires specific techniques tailored to its unique characteristics. The implications of using proper acquisition methods are vast, ranging from improved data quality to the enhanced ability to discern intricate biological details.
Sample Collection Protocols
Getting samples is a crucial step in any omics study. It's not just about scooping up biological material; it's about doing it right to ensure the integrity of the samples. Depending on the study, procedures might differ widely, but they usually fall into a few overarching categories.
- Source of Samples: This can include blood, tissue, or even environmental samples. The choice depends on your research question.
- Handling Guidelines: Following strict protocols minimizes contamination, which can skew results. For instance, if you’re collecting human tissue, you need to ensure that the samples are preserved immediately after collection.
- Storage Conditions: Samples should be stored under conditions that prevent degradation. For many biological samples, freezing is necessary to halt enzymatic activities that could alter the data.
Being diligent about these protocols can be the difference between results that sparkle and those that fizzle out into noise.
High-Throughput Sequencing Methods
Moving on to the nitty-gritty, high-throughput sequencing methods have revolutionized how we approach genomics. These advanced technologies allow researchers to sequence DNA and RNA at unprecedented speeds and scales. Here are some pointers on why they matter:
- Efficiency: The ability to process millions of sequences in one run has drastically cut down the time researchers need to wait for results.
- Depth of Data: With technologies like Illumina and Pacific Biosciences, scientists can uncover genetic variation that was previously overlooked.
- Cost-Effectiveness: As the technology matures, costs continue to plummet, making these once-expensive methods accessible to even small laboratories.
However, rushing into high-throughput sequencing can lead to challenges in data management and analysis. The sheer volume of data demands robust bioinformatics tools—without them, one might find themselves up a creek without a paddle.
Mass Spectrometry Applications
Let’s not gloss over mass spectrometry, a game-changer in proteomics. This technique allows for the identification and quantification of proteins in a sample, revealing a wealth of information about biological processes. Here’s what to take into account:
- Versatility: From identifying post-translational modifications to quantifying proteomes, mass spectrometry serves many purposes.
- Sensitivity: It can detect even trace amounts of proteins, which is essential when studying complex mixtures.
- Speed: Modern mass spectrometers can analyze samples in real-time, providing insights faster than ever.
Mass spectrometry does come with its hurdles, though, such as the need for skilled technicians and complex data interpretation. Yet, when executed effectively, it can bring forth a treasure trove of insights.
"Proper techniques in data acquisition are not just advisable; they are imperative for the reliability of omics data analysis."
"Proper techniques in data acquisition are not just advisable; they are imperative for the reliability of omics data analysis."
In summary, mastering the data acquisition techniques sets the stage for the entire omics investigation, ensuring that the resulting data are not just accurate but rich with potential research pathways.
Analytical Techniques in Omics
Analytical techniques in omics are absolutely crucial for extracting meaningful information from the vast array of biological data generated through various omics technologies. As the field evolves, the methods employed for analyzing omics data continue to grow in sophistication and complexity. This section will explore different statistical approaches, bioinformatics tools, and the challenges of integrating data. Each of these elements carries significant implications for the accuracy and effectiveness of biological research.
Statistical Approaches
Statistical approaches form the backbone of omics data analysis. They provide the framework needed to make sense of complex datasets and draw valid conclusions from them. In the world of omics, where the sheer volume of data can be staggering, statistical techniques allow researchers to identify patterns and relationships that would otherwise remain obscured.
For instance, multiple testing corrections, such as the Bonferroni correction, are essential when analyzing gene expression data. Given that these datasets can contain thousands of entries, the probability of false positives increases significantly. Employing statistical methods helps mitigate this risk, allowing for more reliable results.
- Common Statistical Methods include:
- ANOVA (Analysis of Variance): Useful for comparing three or more groups.
- Regression Analysis: Helps in understanding relationships between variables.
- Machine Learning Techniques: Such as clustering and classification, these methods can uncover intricate patterns within large omics datasets.
The incorporation of these statistical approaches not only enhances data reliability but also makes it feasible to generate predictive models. Thus, robust statistical analysis becomes indispensable in validating hypotheses and supporting experimental findings.
Bioinformatics Tools
The age of omics has ushered in a suite of bioinformatics tools designed specifically for processing complex biological data. These tools facilitate data storage, manipulation, and analysis, enabling researchers to draw insights from potentially overwhelming quantities of information.
Popular bioinformatics tools include:
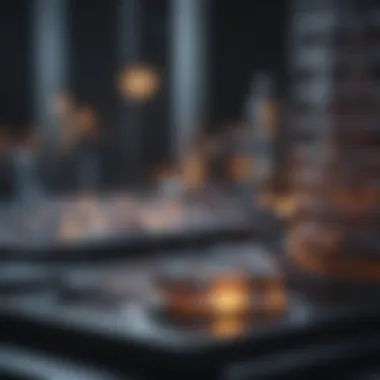
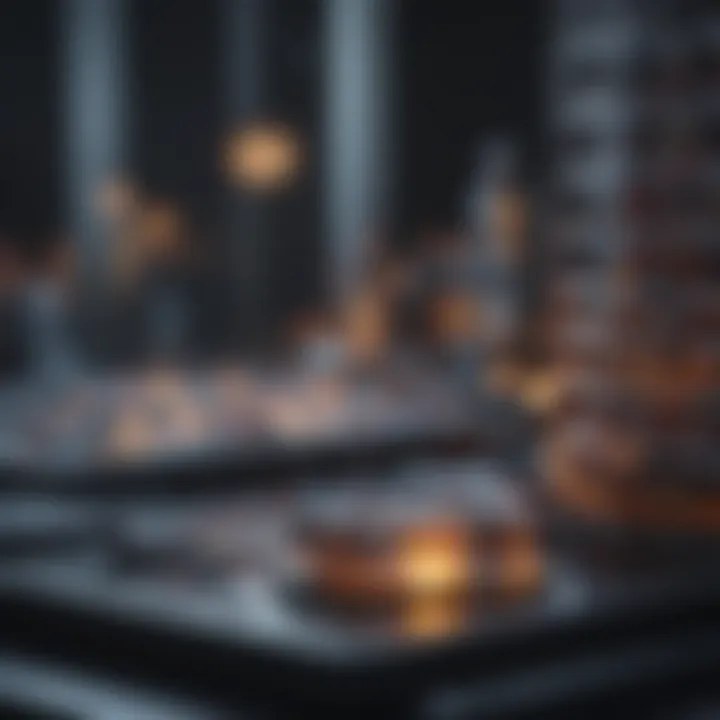
- Bioconductor: An open-source project that offers tools for the analysis and comprehension of high-throughput genomic data.
- Galaxy: A web-based platform that allows users to perform data integration and analysis without needing programming skills.
- GSEA (Gene Set Enrichment Analysis): This tool determines whether a set of genes shows statistically significant differences between two biological states.
Each tool has its strengths and limitations, and the choice often depends on the specific needs of the project. Therefore, familiarity with multiple tools is beneficial for researchers aiming to optimize their data analysis workflows. The continuous development of bioinformatics tools reflects the dynamic nature of this field, helping to keep pace with rapidly evolving scientific questions.
Data Integration Challenges
Despite the advancements in analytical techniques, integrating diverse omics datasets remains a notable challenge. Datasets can vary greatly in terms of format, scale, and quality, which complicates their analysis. Without a unified framework for data integration, researchers may face hurdles in obtaining comprehensive insights from their investigations.
Common challenges include:
- Data Quality Issues: Poor quality can stem from numerous sources, including human error during sample collection and technical limitations of the employed technologies.
- Heterogeneity of Data Types: Merging data from genomics, transcriptomics, proteomics, and metabolomics requires careful considerations of how each type of data relates to the others.
- Computational Demands: The need for intensive computational resources can delay analyses and make it difficult for smaller labs to keep up with larger projects.
Given these challenges, it becomes clear that successful integration relies on collaborative efforts among professionals from different disciplines. This collaboration promotes shared learning and innovation, helping scientists navigate the complexities inherent in omics data analysis.
"The future of omics analysis lies in our ability to synchronize our approaches and technologies, bridging gaps between disciplines and advancing our understanding of biological systems."
"The future of omics analysis lies in our ability to synchronize our approaches and technologies, bridging gaps between disciplines and advancing our understanding of biological systems."
In summary, analytical techniques in omics play an essential role in making sense of complex biological data. By employing appropriate statistical methods, leveraging cutting-edge bioinformatics tools, and navigating integration challenges, researchers can enhance their findings, advancing the field of biology in significant ways.
Applications of Omics Data
The utilization of omics data isn't simply an academic exercise; it's becoming increasingly pivotal in several significant fields including healthcare, agriculture, and environmental studies. As scientists and researchers plunge into the depths of this vast data, the applications of omics data are revealing themselves to be both diverse and impactful. Not only does this data enhance our understanding of biological systems, but it also catalyzes advancements in treatments and practical applications that directly benefit society.
Disease Research Implications
At the heart of omics applications is their profound potential in disease research. By dissecting the genomic and proteomic landscapes, researchers can pinpoint the molecular underpinnings of various ailments. For instance, understanding the genetic variations associated with cancers can help in developing targeted therapies. This
"Precision medicine approach, driven by omic data, tailors treatment pathways based on individual genetic profiles."
"Precision medicine approach, driven by omic data, tailors treatment pathways based on individual genetic profiles."
Moreover, biomarkers identified through metabolomics can indicate disease susceptibility long before symptoms manifest. This proactive stance opens a new frontier, shifting the paradigm from reactionary medicine to anticipatory care. Such insights cultivate a deeper comprehension of disease mechanisms, leading to innovative treatment protocols that could elevate patient outcomes.
Personalized Medicine Strategies
Personalized medicine stands at the forefront of medical innovation, and omics data plays an instrumental role in its evolution. In essence, this practice aims to tailor medical treatments to individual characteristics, conditions, and preferences, shifting away from the one-size-fits-all model. The integration of genomics, proteomics, and other omics data allows for a detailed assessment of an individual's risk for certain diseases, their likely response to drugs, and even potential side effects.
For example, pharmacogenomics, a subset of genomics, examines how genes affect individual responses to drugs. By aligning medication choices with a patient’s genetic makeup, healthcare providers can enhance efficacy while minimizing adverse reactions, thus refining the treatment course. This method shows promise particularly in complex areas like oncology, where individual cancer profiles dictate very specific intervention strategies. The convergence of omics data in this context not only optimizes treatment efficacy but also fortifies the patient's engagement in their own healthcare journey, providing a sense of empowerment and agency.
Agricultural Enhancements
Moving beyond the confines of healthcare, the applications of omics data extend into the agricultural realm as well. With the global population on the rise, ensuring food security is a pressing challenge. By employing omics approaches, researchers can boost crop yields, improve nutritional content, and enhance resistance to pests and diseases.
The ability to sequence plant genomes enables the identification of genes associated with desirable traits. This wealth of data facilitates selective breeding practices that are more targeted and efficient than traditional methods. Moreover, understanding the metabolomic profiles of crops can lead to the development of varieties that thrive in suboptimal conditions, reducing dependence on chemical inputs. In a world grappling with climate change, these advancements in agriculture are not just beneficial; they are necessary to cultivate a resilient food system capable of withstanding future challenges.
Interdisciplinary Collaboration
Interdisciplinary collaboration in omics data analysis is like weaving a tapestry of knowledge, where each thread contributes to a richer, more comprehensive view of biological phenomena. By bringing together experts from various fields—such as biologists, data scientists, clinicians, and statisticians—this collaboration leads to a more holistic understanding of complex datasets. The convergence of diverse expertise not only enriches the analytical framework but also enhances the accuracy and applicability of research findings.
One of the striking features of this field is how much it benefits from ongoing dialogue among its contributors. Unlike traditional scientific research, which often leans heavily on a single discipline, omics data analysis thrives on shared knowledge. For instance, a biologist may provide insights into a specific cell type, while a data scientist can apply sophisticated modeling techniques that illuminate patterns within that cell's genomic data. Such synergy fosters innovation, driving advancements that no single discipline could achieve alone.
Role of Data Scientists
Data scientists occupy a pivotal position within interdisciplinary collaborations concerning omics. They are the architects of analytical frameworks, utilizing programming languages and computational tools to manage and interpret vast amounts of data. Their expertise in machine learning and statistical analysis transforms raw data into actionable insights, helping researchers identify trends and correlations that might otherwise go unnoticed.
To effectively harness the power of omics data, data scientists must not only be adept at coding and data manipulation but also have a foundational understanding of biological concepts. Communication skills are equally important, as they need to converse comfortably with biologists and clinicians about their findings. Here are some of the core functions data scientists perform in the context of omics:
- Develop algorithms for data processing and visualization.
- Clean and preprocess data to ensure quality and reliability.
- Perform statistical analyses to identify significant patterns.
- Collaborate with wet lab scientists to design experiments driven by preliminary data insights.
By engaging in these activities, data scientists effectively bridge the gap between complex datasets and practical applications in biological research.
Integration with Clinical Practices
The integration of omics data analysis into clinical practices marks a shift towards more personalized approaches to medicine. This collaboration is instrumental for applying genetic and proteomic insights to patient care. Clinicians rely on omics data to make informed decisions about diagnosis, treatment, and patient management. For example, insights gained from a patient's genomic data can guide targeted therapies, improving therapeutic outcomes.
In clinical settings, the successful application of omics requires seamless interaction among multiple players, including clinicians, researchers, and clinical informatics specialists. The journey from raw omics data to patient application involves several critical steps:
- Data Interpretation: Clinicians need data scientists to translate complex analyses into understandable terms applicable in medical contexts.
- Identifying Biomarkers: Interdisciplinary teams work to correlate omics data with disease markers, enabling the discovery of potential diagnostic tools.
- Impact Assessment: Monitoring and analyzing how various patient demographics respond to personalized treatments support ongoing refinements.
With the push towards precision medicine, the demand for integrated teams that can effectively face these challenges is more crucial than ever. The shared goal is common: to improve patient outcomes based on robust, data-driven research.
"Interdisciplinary collaboration is essential in unlocking the full potential of omics data for clinical applications."
"Interdisciplinary collaboration is essential in unlocking the full potential of omics data for clinical applications."
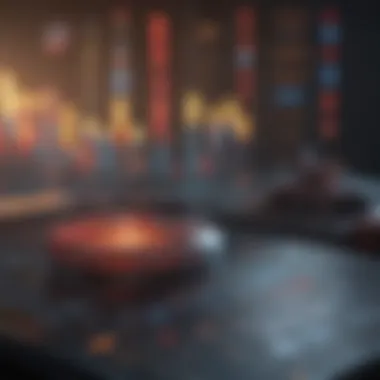
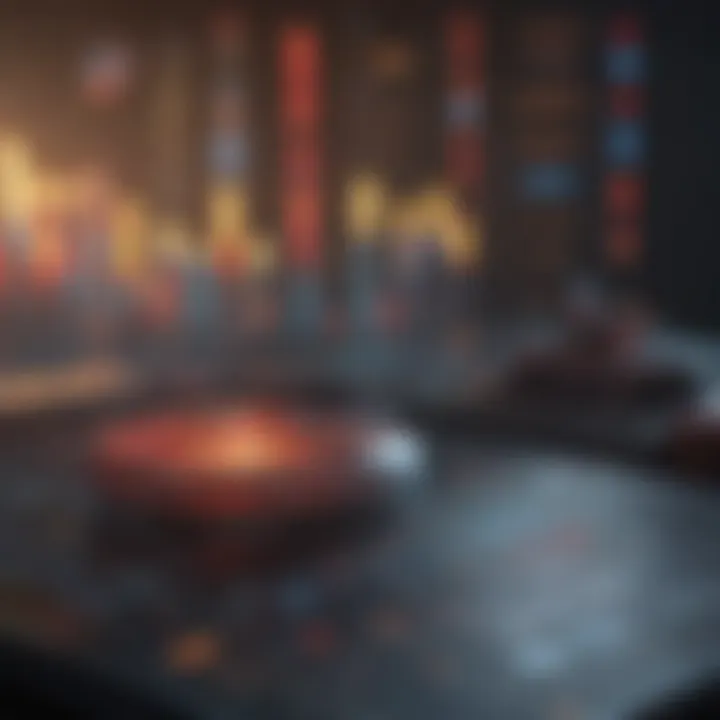
The fusion of disciplines not only enhances current methodologies but also presents fresh avenues for innovation, ensuring the field continues to evolve and adapt to the ever-changing landscape of biological inquiries.
Challenges in Omics Data Analysis
Omics data analysis presents numerous challenges that researchers and practitioners must navigate carefully. Understanding these challenges is crucial not just for the accuracy of conclusions drawn from the data, but also for the broader implications on scientific research, therapeutic development, and policy-making. As the fields of genomics, proteomics, transcriptomics, and metabolomics evolve, the complexity of data grows exponentially. Researchers must confront various data quality issues, interpretational difficulties, and ethical dilemmas that can profoundly affect the outcomes of their analyses.
Data Quality Concerns
Data quality is a cornerstone in the reliability of omics analyses. High-quality data is essential; otherwise, conclusions made from poor data could lead to misguided pathways in research. Issues such as sample contamination, improper handling, and inaccurate measurements can greatly skew results. However, ensuring data quality goes beyond mere technical procedures. Researchers tend to face challenges like:
- Batch Effects: Variability introduced when different groups of samples are processed at different times, which can distort the results.
- Missing Data: Often, certain observations cannot be collected, leading to incomplete datasets that can complicate analyses.
- Inconsistency: Variability that arises from differences in measurement techniques across labs or instruments.
To tackle these quality concerns, implementing robust protocols for sample collection, processing, and analysis is key. It requires diligence and attention to detail, as even the smallest oversight can lead to significant discrepancies.
Interpretation Hurdles
Once the data is collected and cleaned, interpreting the results poses its own set of challenges. The sheer volume of data generated from omics studies can be overwhelming, making it difficult to distill meaningful insights. Common hurdles in interpretation include:
- Complexity of Biological Systems: Biological processes are inherently intricate. Understanding how various omics layers interact without oversimplifying is demanding.
- Statistical Challenges: The need for advanced statistical methods to accurately analyze high-dimensional data often brings its own complications. Selecting appropriate models and avoiding overfitting are critical considerations.
- Contextual Misinterpretation: Data can often be accurate but still misunderstood. For instance, a biomarker identified in one condition may not apply to another context, leading to potentially erroneous conclusions.
To mitigate these interpretation challenges, collaboration across disciplines—bringing together biologists, statisticians, and data scientists—can foster a more comprehensive understanding of the data's implications.
Ethical Considerations
Ethics is another crucial realm that cannot be ignored in omics data analysis. As researchers delve deeper into personal genomic data, the stakes for ethical responsibility rise. Key ethical concerns include:
- Privacy Issues: Safeguarding sensitive genetic information is paramount. Researchers must ensure that personal data is de-identified to protect individuals’ privacy.
- Informed Consent: Participants in omics studies must be fully informed about how their data will be used, which can be complex given the multidisciplinary nature of research.
- Potential Misuse: There’s the risk that data may be utilized for nefarious means, such as discrimination based on genetic information.
"Navigating the ethics of omics data is not just a matter of policy, but one of ensuring trust between researchers and participants."
"Navigating the ethics of omics data is not just a matter of policy, but one of ensuring trust between researchers and participants."
A transparent approach to data collection and analysis, reinforced by ethical oversight, can help establish a framework in which participants feel respected and valued.
Future Directions in Omics
Understanding the future directions in omics is essential because it paves the pathway for advancements in biological and medical research. As we stand on the brink of new discoveries, the innovations on the horizon promise to transform our understanding of complex biological systems. With numerous fields continually evolving, it’s crucial to explore how these advancements can be integrated into current research practices. The relationship between improvements in methodologies and computational biology enhances our ability to analyze and interpret enormous datasets.
Innovations in Methodologies
In recent years, numerous innovative methodologies have emerged in the field of omics, signaling a shift toward more efficient and precise approaches. One such methodology is single-cell sequencing, which allows researchers to analyze the genome or transcriptome of individual cells. Unlike traditional techniques that consider bulk populations, this can uncover heterogeneity, providing insights into cellular functions and differentiation processes at a granular level. This is particularly relevant for cancer research, where tumor cells may present vastly different characteristics.
Moreover, techniques like CRISPR-Cas9 have revolutionized genomic editing, enabling researchers to modify DNA at specific locations. This technology enhances the reliability of genomic studies and fosters the development of tailored treatments. It’s also worth mentioning advances in high-throughput proteomics that enable the simultaneous identification and quantification of thousands of proteins, further enriching the depth of omics analysis.
As methodologies evolve, the integration of multi-omics approaches is gaining traction. People are now capable of combining genomics, proteomics, and metabolomics data to provide a more comprehensive view of biological processes. This holistic perspective can greatly improve how diseases are understood and treated, considering both genetic and environmental factors.
"The future is not something we enter. The future is something we create."
– Leonard I. Sweet
"The future is not something we enter. The future is something we create."
– Leonard I. Sweet
Trends in Computational Biology
The rise of computational biology is profoundly shaping the landscape of omics research. Machine learning and artificial intelligence are becoming vital partners in data analysis, enabling researchers to glean insights from large datasets that were previously impossible to interpret. For instance, algorithms can predict protein structures with remarkable accuracy, as seen in projects like AlphaFold. This allows for better understanding of how proteins interact and function, which is essential for drug development.
Additionally, cloud computing and advanced data storage solutions are facilitating access to large datasets from anywhere in the world. Interoperability between different tools and databases improves collaboration among scientists from diverse fields. The availability of resources such as open-access databases is fostering a culture of shared knowledge that accelerates innovation.
Furthermore, data visualization tools play an important role in making complex data more accessible. Sophisticated software can transform intricate datasets into understandable, interactive graphics, helping researchers and clinicians alike grasp the implications of their findings swiftly.
Overall, the intertwining of innovative methodologies and cutting-edge computational tools is setting the stage for a transformative era in omics. Researchers are no longer merely analyzing data; they're creating a narrative that enhances our understanding of life at its very core.
Closure
In the realm of omics data analysis, the conclusion serves as a pivotal juncture where all threads of inquiry converge. It encapsulates not only the breadth of knowledge shared but also emphasizes the essential insights gleaned from the exploration of various omic fields. This article has charted the landscape of genomics, proteomics, transcriptomics, and metabolomics, showcasing the methodologies utilized and the relevance of interdisciplinary collaboration.
Understanding the intricate tapestry woven by these studies is crucial. First, the conclusion reiterates the significance of maintaining a holistic perspective when analyzing omics data. The intersectionality of these fields enhances the depth of understanding in biological complexities and clinical implications. It offers a veritable toolkit for researchers, pushing them to employ a mélange of techniques, from statistical approaches to bioinformatics tools, thereby addressing potential challenges.
Moreover, it shines a light on the importance of addressing data quality concerns and ethical considerations. Acknowledging these aspects substantiates the commitment to scientific integrity and the earnest pursuit of innovation. Therefore, the conclusion not only reviews findings but also advocates for responsible practices in the handling and interpretation of omics data.
Summary of Findings
The exploration of omics data has revealed several crucial findings that shape our understanding of biological systems:
- Diverse Applications: From disease research to agricultural advancements, the utility of omics is extensive. Each type of omic data brings unique insights that can dramatically alter research trajectories.
- Interdisciplinary Approach: Collaboration across disciplines is paramount. Data scientists, clinicians, and biologists must work hand in hand to interpret complex data accurately.
- Analytical Techniques: The diversity of analytical methods—including high-throughput sequencing and mass spectrometry—highlights that there is no one-size-fits-all in omics research. The choice of tools must align with specific research goals and the nature of the data.
- Future Directions: Innovations in methodology and trends in computational biology point towards a future that promises even greater integration and application of omics data in personalized medicine.
These findings underscore the vital role of omics in scientific advancement today. Gathering this knowledge enables researchers and practitioners to reimagine the possibilities within their respective fields.
Implications for Future Research
Looking ahead, it’s clear that the implications for future research in omics data analysis are profound. The continuation of this exploration holds potential for:
- Enhanced Personalization in Medicine: As researchers delve deeper into genomic data, the ability to tailor medical treatment to individual patients will only improve, leading to better outcomes and patient satisfaction.
- Integration of Technologies: The ongoing development of technologies such as artificial intelligence and machine learning can vastly improve data interpretation. By harnessing these advancements, researchers can analyze large-scale datasets more effectively and efficiently.
- Collaborative Framework: The establishment of collaborative research networks can encourage knowledge sharing and standards across disciplines, ensuring that diverse insights contribute to a more comprehensive understanding of biological phenomena.
- Ethical Frameworks: As omics research progresses, discussions surrounding ethical considerations must also evolve. It is crucial to establish ethical guidelines that ensure responsible usage of genetic and biological information.