Exploring Digital Data: Implications and Applications
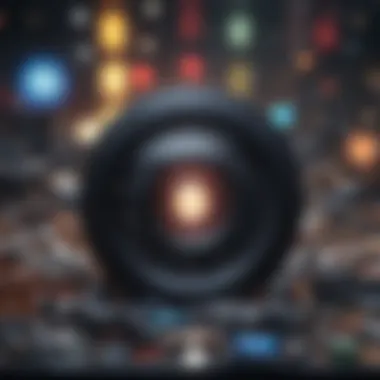
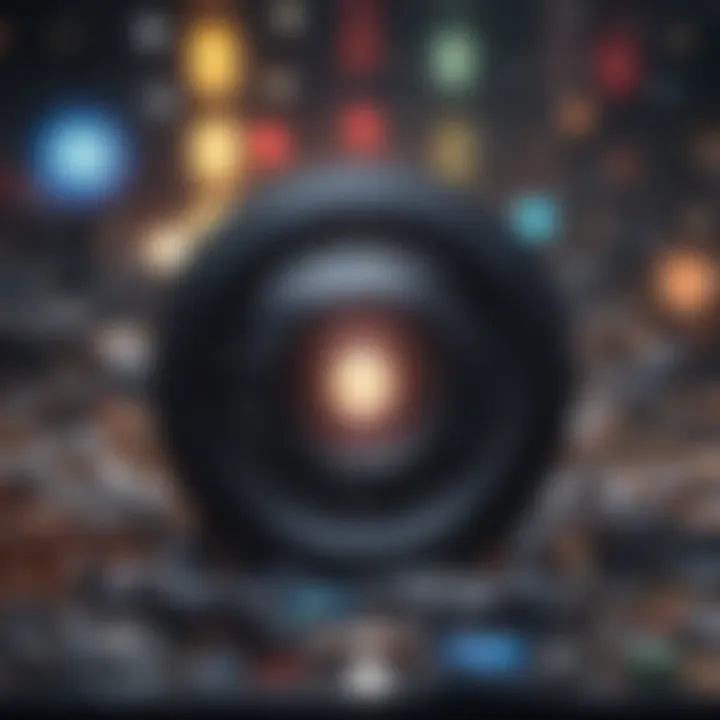
Article Overview
Purpose of the Article
Digital data has become a vital component of modern life. This article seeks to uncover its complexities and implications. The aim is to inform readers about the different types of digital data, its collection methods, and the ethical dilemmas it presents. By scrutinizing these facets, the article emphasizes the relevance of digital data in scientific research and everyday applications.
Relevance to Multiple Disciplines
The implications of digital data stretch far and wide. Its relevance can be seen in various fields such as medicine, social science, engineering, and business analytics. Researchers and professionals must understand these applications to harness the full potential of data in decision-making processes.
Research Background
Historical Context
The evolution of digital data began with early computing in the mid-20th century. Back then, data was largely confined to numerical inputs and simple databases. The rise of the internet and digital technologies in the late 1990s broadened the scope of data creation. Today, data is generated at unprecedented rates, transforming how information is stored and retrieved. This change has significantly impacted various disciplines, facilitating enhanced research capabilities and new methodologies.
Key Concepts and Definitions
To properly engage with digital data, it is crucial to understand some key concepts:
- Digital Data: Information that is encoded in a binary format, enabling it to be processed by computers and digital devices.
- Data Collection: The systematic gathering of information for analysis. This can take various forms, including surveys, sensor readings, or social media interactions.
- Data Privacy: The ethical and legal considerations regarding the management and protection of personal data.
These definitions provide a foundation for diving deeper into the complexities of digital data analysis and its broader impacts on society.
"Data literacy is becoming essential. Individuals who can read and understand data can draw more meaningful insights."
"Data literacy is becoming essential. Individuals who can read and understand data can draw more meaningful insights."
Understanding these core elements encourages a more nuanced exploration of digital data and its implications.
Preamble to Digital Data
The concept of digital data is critical in today’s information-driven society. Understanding its importance forms the foundation of this discussion. Within this article, the exploration of digital data focuses on its definition, characteristic features, and the role it plays in various scientific disciplines.
Definition and Scope
Digital data refers to information that is stored in a digital format. This type of data can encompass a wide range of formats, including text, numbers, images, and videos. The scope of digital data expands as technology evolves and data generation accelerates. Major sources include social media, scientific research databases, IoT devices, and business transactions.
Definition of digital data includes two main categories: structured data, which is easily searchable and organized (like databases), and unstructured data, such as emails and multimedia content. The significance of knowing these categories cannot be overstated, as it underpins many analytical approaches and tools used across fields.
Importance in Scientific Research
Scientific research increasingly relies on digital data. Researchers utilize vast amounts of information to draw conclusions and forecasts. From health sciences to social studies, data driven conclusions are essential. This digital shift improves the precision of research and enhances the capability to make evidence-based decisions.
Some reasons digital data is pivotal in scientific research include:
- Accessibility: Data can be shared and accessed on a global scale, fostering collaboration.
- Efficiency: Sources of data can be analyzed rapidly using advanced computational methods.
- Innovation: Emerging technologies can lead to new insights and breakthroughs, pushing boundaries in various scientific fields.
"The true power of data lies in its potential to transcend geographical and disciplinary boundaries."
"The true power of data lies in its potential to transcend geographical and disciplinary boundaries."
Fostering a deeper understanding of digital data ensures researchers can leverage its capabilities, ultimately enhancing the quality of scholarly work. By dissecting each aspect of digital data in this article, we aim to provide a thorough exploration, benefiting those engaged in academic pursuits, industry practices, and technological advancements.
Types of Digital Data
The exploration of digital data is incomplete without understanding its various types. The types of digital data significantly affect how it is collected, analyzed, and applied across different fields. Recognizing these categories can enhance the effectiveness of data-driven decisions and research methods.
Structured vs. Unstructured Data
Structured data is highly organized and easily searchable. It often resides in fixed fields within a record or file, and can be managed in traditional databases like MySQL or Oracle. Examples include spreadsheets and relational databases. The predictability of structured data makes it suitable for routine tasks and automated processes.
In contrast, unstructured data lacks a predefined format or structure. This type comprises a vast array of information, including text, images, and videos, accessible in formats such as emails, social media posts, and multimedia files. Managing unstructured data poses challenges due to its complexity, but it holds rich insights for organizations willing to invest in advanced analytical methods to extract value from it.
Effectively categorizing data as either structured or unstructured allows organizations to choose appropriate tools and techniques for analysis. Thus, understanding these distinctions is crucial for accurate data management and potential applications.
Quantitative and Qualitative Data
Quantitative data provides measurable, numerical values. It is particularly useful for statistical analysis, enabling researchers to formulate hypotheses and examine trends through numerical metrics. Surveys, experiments, and sensor data typically yield quantitative results. The analysis of this data type can guide strategic decisions in various domains such as business and healthcare.
On the other hand, qualitative data encompasses non-numerical information, aiming to understand underlying reasons and motivations. This data type is invaluable for exploring complex phenomena where numbers alone do not suffice. Methods like interviews and open-ended survey responses produce qualitative data, offering deep insights into human behavior and attitudes.
Addressing both quantitative and qualitative data enriches research findings and informs a more nuanced understanding of the subject matter. Their combined insights can lead to a holistic view, supporting informed decision-making.
Big Data and Its Implications
Big Data refers to large volumes of data that cannot be easily processed using traditional methods. It encompasses both structured and unstructured types of data collected from diverse sources like social media, devices, and sensors. As organizations increasingly harness Big Data, they are able to identify patterns and trends that can inform future strategies.
The implications of Big Data are far-reaching. It drives innovation across sectors by enabling organizations to operate efficiently and make data-informed decisions. For instance, predictive analytics in healthcare leverages Big Data to anticipate disease outbreaks, thus enhancing responsiveness. Furthermore, businesses analyze customer feedback and purchasing behavior to refine marketing strategies.
However, managing Big Data requires advanced tools and infrastructure, along with skilled personnel who understand its complexities. As a result, organizations must strike a balance between harnessing Big Data's potential and addressing the inherent challenges it presents. Such consideration is vital for effective data governance and utilization.
"Understanding the different types of digital data is fundamental to optimizing information management and analysis."
"Understanding the different types of digital data is fundamental to optimizing information management and analysis."
In summary, the classifications of digital data shape every aspect of how we approach data challenges and opportunities. Each type has unique characteristics that require tailored strategies for effective use.
Collection Methods of Digital Data
Digital data collection methods are crucial in ensuring that the information gathered is relevant, accurate, and can serve the intended purpose. Various methods exist, each offering distinct advantages and challenges. The choice of a collection method must align with the goals of the research, the nature of the target population, and the type of data needed. Understanding these methods can significantly enhance the quality of research outcomes.
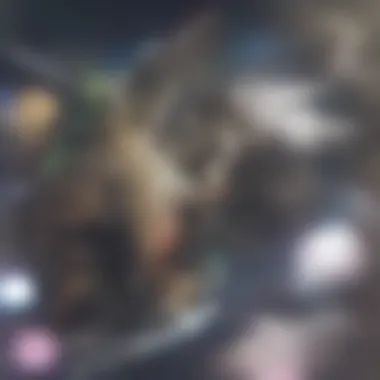
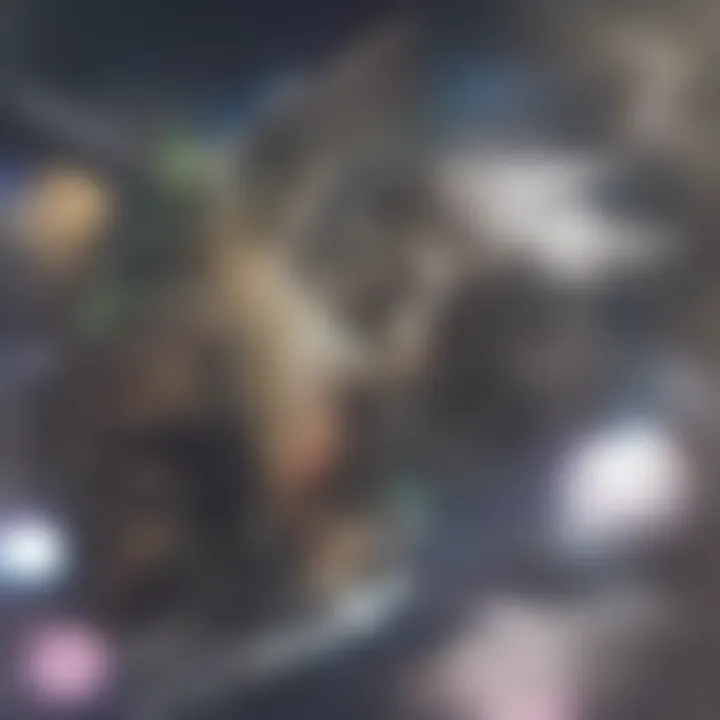
Surveys and Questionnaires
Surveys and questionnaires are popular methods for collecting data. They allow researchers to gain insights from a large number of respondents in a relatively short time. The structured format typically includes closed and open-ended questions that help in gathering quantitative and qualitative data.
Importance
The primary strength of surveys is their ability to discern patterns across a population. They can target specific demographics, making it easier to identify trends. For instance, in healthcare research, surveys can reveal patient satisfaction levels or treatment outcomes.
Considerations
While surveys can be efficient, certain considerations must be addressed:
- Design: Questions must be clear to avoid confusion.
- Sample size: A larger sample size can lead to more reliable results.
- Response bias: Researchers should account for potential biases in responses to ensure authenticity.
Ultimately, surveys and questionnaires serve as invaluable tools for data collection, but careful design is paramount.
Observational Data Collection
Observational data collection involves monitoring subjects in their natural environment without interference. This method is particularly useful in fields like sociology, psychology, and ecology, where researchers seek to understand behavior in context.
Importance
Observation provides real-time data that reflects actual behaviors and interactions. It reduces the risk of response bias because participants are unaware of being studied. For example, a researcher studying classroom dynamics may observe students’ interactions without their knowledge, capturing authentic behavior both socially and academically.
Considerations
Although observational methods can yield rich data, they do have limitations:
- Subjectivity: The observer's biases may influence data interpretation.
- Time-intensive: Gathering data through observation can take considerable time.
- Ethical concerns: Researchers must ensure that their methods comply with ethical standards, particularly regarding consent.
Sensor and IoT Data Acquisition
Sensor and IoT (Internet of Things) data acquisition represents a groundbreaking method for collecting high volumes of data. This technology utilizes various sensors to record information automatically, ranging from environmental conditions to user behaviors.
Importance
The integration of sensors enables continuous data collection, providing insights that are often impossible to capture through traditional methods. For instance, smart meters in homes collect energy usage data, which can help in understanding consumption patterns and informing energy-saving measures.
Considerations
Despite its advantages, this method comes with challenges:
- Data Management: Handling large amounts of data requires robust infrastructure.
- Security: IoT devices are susceptible to breaches, raising concerns about privacy and data integrity.
- Interpretation: The data generated needs proper analysis to yield actionable insights.
To sum up, sensor and IoT data acquisition offers a modern approach to data gathering, yet it necessitates careful planning and consideration of security facets.
Data collection methods form the backbone of effective digital data utilization, influencing how insights are derived and conclusions are drawn.
Data collection methods form the backbone of effective digital data utilization, influencing how insights are derived and conclusions are drawn.
Challenges in Managing Digital Data
Managing digital data presents a complex array of challenges that can significantly affect its utility and reliability. As the volume of data continues to grow, the issues surrounding data privacy, integrity, and ethical considerations become ever more prominent. Addressing these challenges is essential in ensuring that data can be effectively leveraged for research and decision-making.
Data Privacy Concerns
Data privacy concerns are paramount in today's increasingly digital landscape. With an explosion of data being generated daily, often containing sensitive personal information, safeguarding this data is not just a technical requirement but a moral imperative. The implications of mishandled data can be severe, leading to identity theft, unauthorized access to personal information, and a general erosion of public trust in institutions.
To tackle these concerns, organizations must adopt robust data encryption methods and adhere to strict data governance policies. Laws such as the General Data Protection Regulation (GDPR) in Europe serve as frameworks for ensuring data is collected, stored, and handled with respect for individual privacy. Users should have clarity on how their data is utilized, and consent should always be obtained before utilizing personal information. Ultimately, companies that prioritize data privacy can foster trust and encourage greater participation in data sharing initiatives.
Issues of Data Integrity
Issues of data integrity can compromise the validity of research findings and lead to misguided conclusions. Integrity refers not only to the accuracy of the data but also its consistency throughout its lifecycle. Data can become corrupted through various means: software failures, human error, or improper data handling during the collection process.
To ensure data integrity, organizations should implement rigorous validation techniques and establish comprehensive auditing processes. Regular checks can detect anomalies or discrepancies in datasets, allowing for timely rectification. Establishing clear protocols for data entry and processing is crucial. Researchers must be trained to understand these guidelines, which contribute to maintaining high standards of integrity in their work. By prioritizing integrity, the credibility of research outcomes is retained, positively impacting scientific discourse.
Ethical Considerations in Data Use
Ethical considerations in data use are essential for responsible research and data-driven decision-making. The misuse of data can lead to significant implications, like biased algorithms or discriminatory practices. When data are gathered, they must be used in ways that are socially responsible and beneficial.
Researchers and organizations have a duty to evaluate the consequences of their data usage. This includes assessing whether data applications promote equity and do not inadvertently amplify existing biases. Transparency is a key principle in ethical data use. Stakeholders must be aware of how data are collected, processed, and analyzed.
"Ethical data use is not just an obligation but an opportunity for organizations to demonstrate accountability to their stakeholders."
"Ethical data use is not just an obligation but an opportunity for organizations to demonstrate accountability to their stakeholders."
Data Analysis Techniques
In today's digital world, the significance of data analysis techniques cannot be overstated. These methods enable us to extract valuable insights from vast amounts of data. The implications of effective data analysis often extend beyond simple numerics, guiding crucial decisions across various sectors. Techniques such as statistical methods, machine learning algorithms, and natural language processing not only enhance understanding but also improve outcomes in real-world applications. As organizations grapple with increasing data volumes, the need for robust and effective analysis becomes essential.
Statistical Methods for Data Analysis
Statistical methods provide a foundation for data analysis through systematic approaches to interpreting numerical data. They assist in summarizing and understanding trends, identifying relationships among variables, and making predictions. Common statistical methods include regression analysis, hypothesis testing, and variance analysis. Each of these techniques helps in drawing more informed conclusions while considering data variability.
By applying these methods, researchers can better understand sample distributions and variances. For instance, regression analysis not only establishes relationships between dependent and independent variables but also helps in predicting outcomes based on historical data. This approach is particularly useful in fields such as economics and social sciences, where understanding complex interactions is vital. Furthermore, statistical significance testing allows researchers to determine if observed patterns are not mere chance occurrences.
Machine Learning Algorithms
Machine learning algorithms have revolutionized data analysis by introducing computational techniques that learn from and make predictions based on data. These methods, whether supervised or unsupervised, adapt over time, enhancing their ability to provide insights. Common algorithms include decision trees, support vector machines, and neural networks.
The primary advantage of machine learning lies in its ability to process large datasets efficiently, revealing patterns that traditional statistical methods might overlook. For instance, in finance, machine learning can analyze spending patterns to detect fraudulent activities. In health care, it helps predict patient outcomes based on historical data, leading to timely interventions. However, it is important to consider the ethical implications of machine learning, particularly regarding bias in training data.

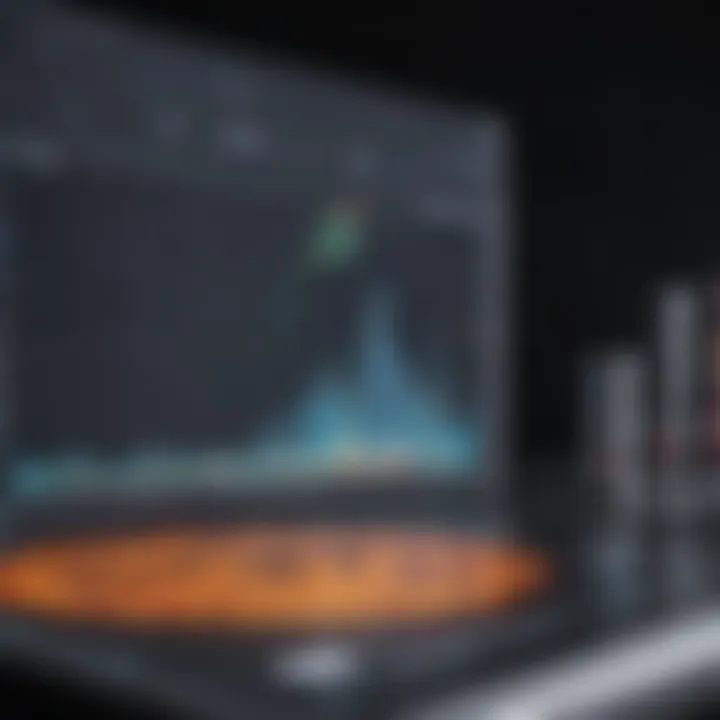
Natural Language Processing Applications
Natural Language Processing (NLP) applies algorithms and computational techniques to understand and generate human language. This field enables automated systems to process and analyze vast amounts of text data, providing significant insights in areas like sentiment analysis, topic modeling, and information retrieval.
The application of NLP is extensive. For example, businesses use sentiment analysis to gauge consumer opinions about products and services by examining social media conversations. Academic researchers utilize topic modeling to identify themes across large corpuses of literature. Moreover, NLP transforms unstructured data into actionable insights, thereby enhancing decision-making processes. As language continues to evolve, staying updated with NLP advancements is crucial for leveraging its full potential in data analysis.
"As we continue to generate data at an increasing pace, understanding how to analyze this data effectively is critical to leveraging its full value."
"As we continue to generate data at an increasing pace, understanding how to analyze this data effectively is critical to leveraging its full value."
The integration of these data analysis techniques provides a comprehensive toolkit for researchers, educators, and professionals alike. The advancements in statistical methods, machine learning algorithms, and natural language processing applications ensure that digital data can be transformed into meaningful insights, driving innovation and enhancing decision-making across numerous fields.
Data Visualization Techniques
Data visualization techniques are essential in translating complex data into understandable and actionable insights. Through visual representation, such as charts, graphs, and maps, data becomes accessible. This section will delve into various aspects of data visualization, highlighting its importance, best practices, and the tools available to aid in effective visualization.
Graphical Representation of Data
Graphical representation of data employs visual elements to convey information rapidly. Important aspects include clarity, precision, and engagement. Well-designed graphs can illustrate trends, highlight correlations, and reveal anomalies. Choosing the right type of graph is crucial. For instance, line graphs are effective for showing changes over time, while bar charts allow for easy comparison between categories. A pie chart might depict part-to-whole relationships succinctly.
Key benefits of graphical representation include:
- Improved Comprehension: Visual aids enhance retention, especially for audiences unfamiliar with the subject matter.
- Quick Insights: Graphs can summarize large datasets, allowing for faster decision-making.
- Identification of Patterns: Visual representation often uncovers insights that raw data fails to reveal.
Dashboards for Data Interpretation
Dashboards serve as consolidated displays of key performance indicators and metrics. They facilitate real-time monitoring and analysis, allowing stakeholders to grasp complex information quickly. A well-structured dashboard displays various forms of data at a glance, making it easier to interpret and act upon.
Key considerations in dashboard creation include:
- User-Friendliness: A clean, organized layout aids user engagement.
- Interactivity: Features that allow users to filter data can enhance the understanding of specific metrics.
- Regular Updates: Dashboards must be current to provide relevant insights.
Dashboards are indispensable tools for businesses seeking to integrate data into their strategies.
Tools and Software for Visualization
Numerous tools exist for data visualization. The choice of tools can impact the efficiency of turning data into meaningful visuals. Notable software includes Tableau, Microsoft Power BI, and Google Data Studio. Each tool comes with unique features catering to different visualization needs.
Important factors to consider when selecting a visualization tool include:
- Ease of Use: A straightforward interface encourages users to explore data independently.
- Integration Capabilities: The ability to pull in data from various sources enhances functionality.
- Customization: Tools offering advanced customization enable brands to maintain consistency with their visual identity.
In summary, data visualization techniques serve as powerful allies for researchers, educators, and professionals. By ensuring effective communication of data, these techniques play a vital role in making informed decisions and strategic planning.
Emerging Technologies and Data
Understanding the intersection of emerging technologies and data is essential for grasping contemporary trends in digital information management. Technologies such as Artificial Intelligence, Blockchain, and Cloud Computing significantly influence how data is utilized, secured, and stored. These advancements offer notable benefits, but they also come with their own set of considerations that must be understood by stakeholders in various fields.
Artificial Intelligence and Data Utilization
Artificial Intelligence (AI) transforms the way data is processed and analyzed. Through algorithms and machine learning techniques, AI can discover patterns within large datasets that human analysts may overlook. This capability plays a crucial role across many sectors, including healthcare, finance, and marketing.
Key benefits include:
- Enhanced Decision-Making: AI can analyze vast amounts of data rapidly, providing insights that improve decision-making processes.
- Automation of Routine Tasks: Repetitive data tasks can be automated, allowing human resources to focus on more strategic initiatives.
- Predictive Analysis: It helps in forecasting trends and behaviors, which is particularly valuable for businesses aiming to tailor their services.
However, the use of AI also raises questions about data governance and ethical implications, such as biases in data interpretation or decision-making processes.
Blockchain for Data Security
Blockchain technology presents a revolutionary approach to ensuring data integrity and security. Its decentralized nature means that data is stored across a network of computers, making it difficult to alter or hack. This feature is particularly useful in sectors where data security is critical, such as finance and healthcare.
Benefits of blockchain include:
- Transparency: Changes to the data are recorded chronologically, providing an immutable audit trail.
- Enhanced Security: Decentralization minimizes the risk of data breaches, as there is no central point that can be attacked.
- Smart Contracts: These automatically execute transactions based on pre-defined conditions, reducing the need for intermediaries and enhancing efficiency.
While promising, blockchain also faces challenges, such as scalability and regulatory acceptance, which must be navigated for broader adoption.
Cloud Computing and Data Storage
Cloud computing has changed the landscape of data storage and management. It allows organizations to store vast amounts of data remotely. This capability not only reduces infrastructure costs but also enhances accessibility.
Key advantages include:
- Scalability: Organizations can easily adjust their storage and processing powers as their data needs change.
- Cost-Effectiveness: Moving to the cloud often reduces the need for costly physical hardware and maintenance.
- Collaboration: Cloud services enhance collaboration among teams by allowing access to shared data resources from various locations.
However, companies must also consider issues related to data sovereignty and privacy, especially under different regulations across jurisdictions.
"Emerging technologies serve as a catalyst for change, revolutionizing how we manage, secure, and utilize data in an interconnected world."
"Emerging technologies serve as a catalyst for change, revolutionizing how we manage, secure, and utilize data in an interconnected world."
The Role of Data Literacy
Data literacy is essential in today's world where digital data prevails. With the exponential increase in information flow, being data literate is no longer optional. It shapes how individuals engage with data in various contexts, including research, business, and everyday decision-making. This section explores the fundamental aspects of data literacy, its benefits, and its implications in a digital-centric society.
Understanding Data in Context
Grasping data's context is a critical component of data literacy. Understanding where data originates, its purpose, and its relevance ensures individuals can interpret it accurately. Context provides a framework that aids in comprehending not just the numbers or words on a page, but also the underlying story they tell. This understanding can influence decisions across fields like healthcare, finance, and education.
Data presented without context can lead to misinterpretation. For example, a researcher seeing a spike in a certain health metric must understand demographic variables to draw informed conclusions. Data without context becomes ambiguous, and the significance gets distorted.
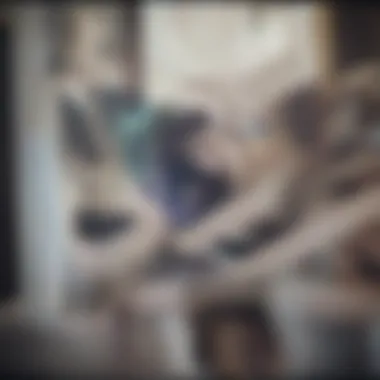
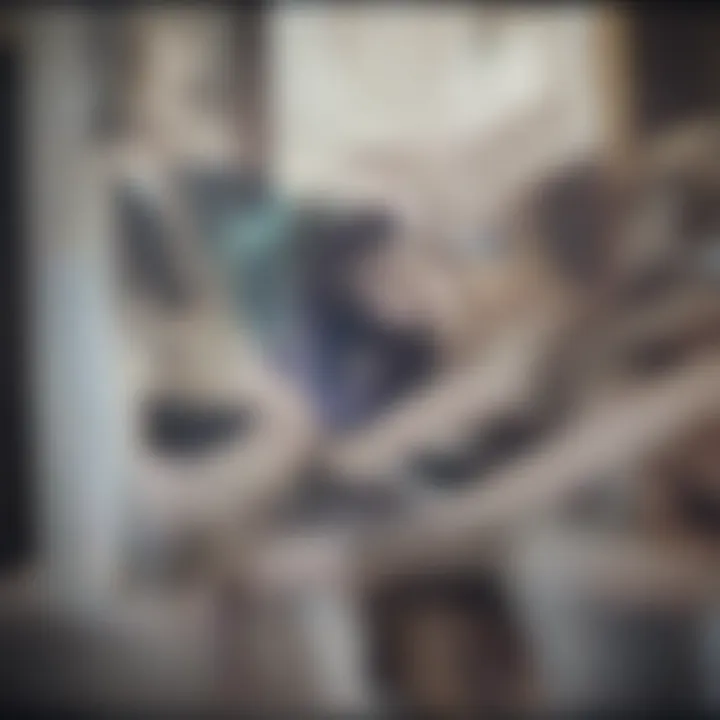
Critical Thinking and Data Interpretation
Critical thinking plays a vital role when interpreting data. It allows for a balanced assessment of information. Instead of taking data at face value, critical thinkers weigh the sources, the quality of the data, and its potential biases. This process fosters a more nuanced understanding and encourages questioning assumptions.
When analyzing data, the following questions can enhance interpretation:
- Who collected this data?
- What methodologies were used?
- Are there any conflicts of interest?
A rigorous approach promotes a culture where data is used responsibly and ethically, allowing people to make decisions grounded in reality, not mere speculation.
Educating Future Generations on Data Usage
Education systems must evolve to prioritize data literacy for future generations. This begins with curriculums inclusive of data skills from an early age. As children learn math and science, they should also learn how to understand and use data. Integrating real-world data projects can provide practical experience.
For example, initiatives like coding programs or data science clubs in schools can foster enthusiasm and competence. When students understand data's power, they innovate in ways that benefit society.
Incorporating data literacy into education helps bridge the gap between theoretical knowledge and practical application. As digital data becomes ubiquitous, ensuring that upcoming generations are prepared becomes imperative.
"Data literacy is the new literacy in a data-driven world. It is crucial for individuals to make informed decisions based on factual analysis."
"Data literacy is the new literacy in a data-driven world. It is crucial for individuals to make informed decisions based on factual analysis."
The focus on data literacy not only enhances personal skills but also contributes to informed citizenship. Being data literate equips people to navigate the complexities of modern life. They become better advocates for themselves and their communities, fostering a culture where data-driven decision-making prevails.
Case Studies of Digital Data Applications
The exploration of digital data applications through case studies is crucial for understanding its transformative impact across various sectors. These studies illustrate real-world applications, enabling researchers and professionals to glean insights and draw lessons that influence strategy and decision-making. They highlight the organic integration of digital data into operational frameworks and showcase the tangible benefits that arise from harnessing this technology. Furthermore, case studies reveal the challenges and ethical considerations that come with data usage, ensuring a holistic understanding of the implications involved.
Healthcare Data Management
Healthcare data management stands as a prime example of how digital data applications can revolutionize an industry. Hospitals and healthcare providers collect vast amounts of patient data. This data includes electronic health records (EHR), imaging studies, and patient demographics. Managing this data effectively results in improved patient outcomes and operational efficiencies.
Benefits include:
- Enhanced accuracy in diagnosis through data analytics predictive modeling, enables healthcare professionals to anticipate health issues before they become critical.
- Streamlined patient care workflows can save time and resources, allowing medical personnel to focus on direct patient interactions.
- Effective use of healthcare data facilitates targeted research, leading to breakthroughs in medical treatments and therapies.
However, several challenges persist. Data privacy is paramount, with regulations like HIPAA in the U.S. safeguarding patient information. The integration of data from various sources presents issues of interoperability, requiring collaborative efforts to standardize data formats and protocols.
Environmental Research and Data Collection
Data collection in environmental research illustrates another compelling application of digital data. By utilizing sensors and IoT devices, researchers monitor variables such as air quality, water levels, and wildlife movements. This real-time data plays critical roles in climate studies, biodiversity assessments, and pollution control efforts.
Key considerations include:
- The ability to gather comprehensive datasets across various environmental parameters strengthens the reliability of research findings.
- Utilizing predictive analytics helps forecast environmental changes, enabling proactive measures for conservation efforts.
- Collaborations between agencies can lead to more robust data sharing, providing a more unified understanding of environmental issues.
However, challenges arise, such as ensuring data collection methods are accurate and representative of real-world conditions.
Business Intelligence and Analytics
In the realm of business, digital data applications are vital for driving intelligence and informed decision-making. Companies leverage analytics to analyze consumer behavior, sales trends, and market dynamics. Tools like Tableau, Power BI, and Google Analytics have become invaluable in deciphering large datasets.
Some clear benefits are:
- Improved customer insights result from extensive data analysis, allowing businesses to tailor products and services to consumer preferences.
- Operational efficiencies can be realized by identifying trends that inform strategic planning and resource allocation.
- Enhanced competitiveness occurs by staying informed about market changes and emerging opportunities.
Nonetheless, there is an ongoing need for businesses to prioritize data quality and security. The risks associated with data breaches can undermine trust and lead to severe repercussions.
"Understanding the real-world implications of digital data applications enhances not just theoretical knowledge but also practical capabilities across sectors."
"Understanding the real-world implications of digital data applications enhances not just theoretical knowledge but also practical capabilities across sectors."
The examination of case studies in digital data applications showcases the significant value this data holds across various domains. By understanding healthcare management, environmental research, and business analytics, stakeholders can better navigate the complexities of data usage and its implications.
Future Trends in Digital Data
The exploration of future trends in digital data is critical for understanding how organizations and societies will interact with data in the years to come. As digital data continues to proliferate, its implications for usage, management, and regulation are profound. An understanding of these trends allows stakeholders to design strategies that leverage data effectively while also navigating the complexities that come with increased volume and variety.
The Evolution of Data Roles in Organizations
In organizations, roles related to data are evolving rapidly. Traditionally, data management was often the purview of IT departments, but that is no longer sufficient. As the reliance on data grows, there is a pressing need for specialized roles such as Data Analysts, Data Scientists, and Chief Data Officers. These professionals not only handle data but also interpret it for decision-making. Their work requires strong analytical skills and a deep understanding of both business needs and advanced data techniques.
- The evolution of these roles is closely tied to how businesses operate.
- Companies are transitioning from legacy systems to integrated data solutions that allow for real-time analytics.
- This shift demands a more diverse skill set among employees, emphasizing continuous learning and adaptability in the workforce.
The implications of these changes are multifold. Organizations see improved efficiency and better strategic alignment. They harness data to drive innovation and maintain a competitive edge.
The Impact of Policy Changes on Data Usage
Policy changes regarding data usage will have significant ramifications. As governments and institutions implement stricter regulations, companies must navigate a landscape of compliance and ethical considerations. Regulations like the General Data Protection Regulation (GDPR) in Europe have set benchmarks for how personal data is collected, stored, and utilized.
Key points to consider are:
- Increases in transparency requirements will necessitate robust data management strategies.
- Non-compliance could result in heavy fines, pushing organizations to invest in compliance training and systems.
- Policy changes could influence public trust; when organizations adhere to ethical standards, they enhance consumer confidence.
These evolving policies will shape not only the operational frameworks of organizations but also public perceptions of data handling and privacy.
Anticipating Technological Advances in Data Science
As we look ahead, technological advances in data science promise to reshape how organizations leverage digital data. Emerging technologies such as Machine Learning, Artificial Intelligence, and the Internet of Things are at the forefront. These technologies enable sophisticated data processing and predictive analytics, allowing for insights that were previously unattainable.
- The rise of automation in data analysis will reduce the manual workload, freeing up professionals to focus on interpretation and strategy.
- Increased processing capabilities will allow even small organizations to harness big data effectively.
- Collaboration between data scientists and subject-matter experts will become increasingly vital as domain-specific insights drive better outcomes.
One important aspect to highlight is the integration of ethical considerations into these technological evolution. Organizations must ensure that their use of technology aligns with ethical best practices, mitigating biases and safeguarding privacy.
"The future of digital data is not just about more data, but about smarter data management, informed usage, and ethical responsibility."
"The future of digital data is not just about more data, but about smarter data management, informed usage, and ethical responsibility."
In summary, grasping the future trends in digital data—through the lens of evolving roles, shifting policies, and technological advancements—equips organizations and individuals to navigate the complexities of this landscape effectively.