Exploring the Spectral Domain: Insights and Implications
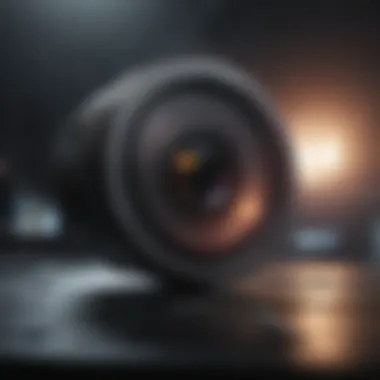
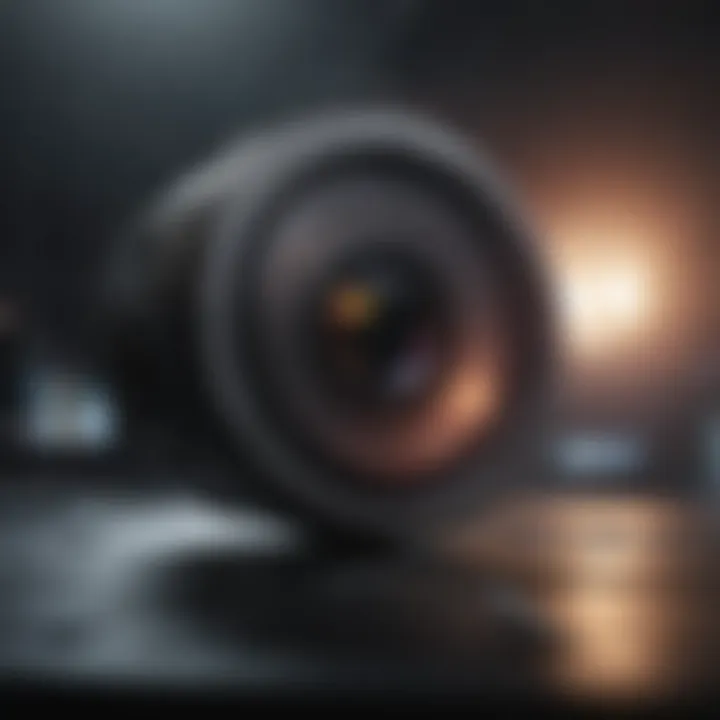
Intro
The spectral domain serves as an important framework in various scientific fields, offering unique analytical perspectives. In this section, we will explore the concept of the spectral domain, its relevance, and how it bridges theoretical and practical applications. The aim is to establish a foundation for understanding how spectral analysis facilitates insights into complex systems.
Understanding the spectral domain begins with defining the foundational terms and concepts. The significance of spectral analysis cannot be understated; it is instrumental in extracting information from signals, spectra, and data sets across multiple disciplines. This article aims to deepen understanding of spectral domain implications, picture how it fits within the broader scientific discourse, and present its current methodologies and future directions.
Intro to the Spectral Domain
The spectral domain is an essential concept that permeates various scientific disciplines. Understanding it is crucial for researchers and professionals who aim to analyze and interpret complex systems accurately. This section serves to familiarize readers with what the spectral domain entails and its significance for analysis across fields such as biology, chemistry, physics, and earth sciences. By laying the groundwork, we can appreciate how spectral methods can illuminate hidden patterns within data.
Definition and Significance
The spectral domain refers to a perspective of data analysis that emphasizes the frequency components of signals. In simple terms, when a signal is transformed into the spectral domain, it's broken down into its constituent frequencies. This transformation allows for a more nuanced examination of the signal, revealing characteristics that may not be apparent in the time domain.
The significance of this approach is substantial. First, it enhances our ability to detect features within signals that may otherwise go unnoticed. For instance, in audio processing, analyzing sound waves in the spectral domain can identify pitches and harmonics that are essential for sound recognition and synthesis. Furthermore, spectral analysis techniques are invaluable in fields such as genomics, where frequency patterns can provide insights into genetic variation.
Among the key benefits of exploring the spectral domain are its applications in noise reduction, feature extraction, and data compression. Signal processing techniques grounded in this domain also facilitate the modeling of complex phenomena in nature and technology. Thus, grasping the spectral domain is not only about mathematical theory; it also translates into practical advantages that drive advancements in research and industry.
Historical Context
The historical evolution of the spectral domain is intertwined with the development of key mathematical tools and theories. In the late 18th century, Joseph Fourier introduced the concept of representing functions as sums of sine and cosine functions in his work on heat conduction. This laid the foundation for what would become the Fourier Transform, one of the cornerstones of spectral analysis.
Subsequent developments in the 20th century accelerated the application of spectral techniques across various scientific domains. For example, the rise of digital signal processing in the mid-20th century transformed how signals were analyzed and manipulated, leading to innovations in telecommunications, audio engineering, and image processing. Moreover, the introduction of wavelet transforms in the 1980s provided even more refined analytical capabilities, allowing for the examination of signals across multiple scales.
This historical background emphasizes not only the mathematical advancements but also the evolving needs of science and technology. As new problems and datasets emerged, the tools for analyzing the spectral domain expanded, demonstrating its growing importance across disciplines. By understanding this context, researchers can appreciate modern techniques' roots and their implications in contemporary scientific inquiry.
Theoretical Foundations
The theoretical foundations serve as the bedrock for understanding the spectral domain. From mathematics to signal processing principles, these fundamentals outline essential methodologies that facilitate analysis across various disciplines. Understanding these concepts can significantly enhance one's ability to interpret data accurately and extract meaningful insights. Thus, it is crucial to grasp the theoretical underpinnings to fully appreciate the applications these techniques offer.
Mathematical Framework
Fourier Transform
The Fourier Transform is a powerful tool in the realm of spectral analysis. Its primary role is to decompose a signal into its constituent frequencies. This is essential for many applications, as it allows for the identification and manipulation of signal characteristics over a range of frequency domains. The key characteristic of the Fourier Transform is its ability to convert time-domain signals into the frequency domain. This transition reveals the frequency spectrum, making it easier to analyze signal information.
One beneficial aspect of the Fourier Transform is its computational efficiency, especially with algorithms like the Fast Fourier Transform (FFT). FFT, in particular, has made the analysis of large datasets practical, enabling real-time processing in various applications. However, the Fourier Transform does have limitations, particularly regarding its treatment of discontinuities. Signals exhibiting rapid changes can lead to spectral leakage, which can affect the accuracy of obtained results.
Wavelet Transform
The Wavelet Transform offers a different approach and complements the capabilities of the Fourier Transform. It is particularly useful for analyzing non-stationary signals that have local characteristics. The key feature of the Wavelet Transform is its ability to capture both frequency and temporal information. This dual representation makes it a popular choice for applications requiring time-frequency analysis, such as image processing and biomedical signal analysis.
The unique aspect of the Wavelet Transform is its multi-resolution analysis ability. This allows for varying degrees of resolution across different scales, providing insight into signals at multiple levels of detail. While this technique has significant advantages, it is comparatively more complex than the Fourier Transform and can require more computational resources, which may be a disadvantage in certain scenarios.
Signal Processing Principles
Sampling Theorem
The Sampling Theorem is a fundamental concept in signal processing, establishing the criteria for converting a continuous signal into a discrete one. The theorem asserts that in order to accurately reconstruct a signal, it must be sampled at least twice the highest frequency present in the signal. This is critical for avoiding loss of information during the conversion process. The key characteristic here is the Nyquist rate, which provides guidelines for selecting the appropriate sampling frequency.
In the context of spectral analysis, the Sampling Theorem ensures that no critical signal information is lost. This makes it a vital principle for both current and future applications in the spectral domain. However, if sampling occurs below the Nyquist rate, it can result in distortion, referred to as aliasing. This underscores the importance of adhering to the theorem for accurate measurements.
Aliasing Effects
Aliasing Effects arise when a signal is sampled below the Nyquist rate, leading to incorrect interpretations of frequency information. This can significantly impact the reliability of a signal analysis and can distort the actual content of the signal. The key characteristic of aliasing is that it introduces misleading lower frequencies in the reconstructed signal, misrepresenting the true nature of the data.
In many cases, avoiding aliasing can be addressed through proper filtering techniques before sampling. These techniques help to retain the necessary signal information while preventing aliased components from distorting the results. While careful consideration of sampling can mitigate these effects, researchers must remain alert to the potential pitfalls of inadequate sampling strategies.
Understanding the theoretical foundations is essential for effectively applying spectral analysis techniques. Each component's insights contribute to a deeper understanding of complex systems across various scientific fields.
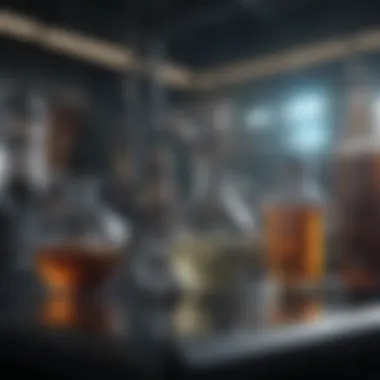
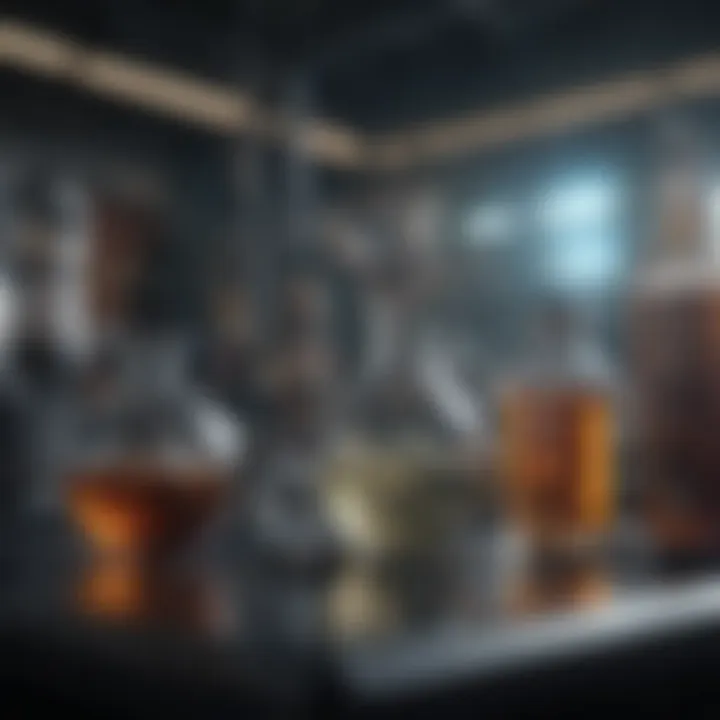
Understanding the theoretical foundations is essential for effectively applying spectral analysis techniques. Each component's insights contribute to a deeper understanding of complex systems across various scientific fields.
Spectral Analysis Techniques
Spectral analysis techniques are fundamental to understanding the spectral domain. They allow researchers to decompose signals into their frequency components. This decomposition is crucial in various fields, enabling insights into complex interactions within the data. These techniques help to simplify analysis, making them indispensable in modern science.
Basic Techniques
Fourier Analysis
Fourier analysis is a powerful mathematical tool used extensively in the spectral domain. Its primary contribution lies in transforming a time-domain signal into its frequency-domain representation. This approach allows researchers to identify dominant frequencies in the signal. One key characteristic of Fourier analysis is its efficiency in processin data. This efficiency makes it a popular choice, particularly in signal processing tasks.
A unique feature of Fourier analysis is its ability to represent periodic signals with sine and cosine functions. This representation provides flexibility when analyzing different kinds of signals. However, while Fourier analysis is beneficial, it does have limitations. For instance, it assumes that signals are stationary, which may not be valid in all real-world situations. Thus, careful consideration is essential when applying this technique.
Spectrograms
Spectrograms serve as a visual representation of the frequency spectrum of signals over time. They contribute significantly to analyzing data by providing a three-dimensional perspective: time, frequency, and amplitude. This visualization is especially useful for observing transient phenomena in signals. The key characteristic of spectrograms is their ability to display how the frequency content of a signal evolves over time. This feature makes them a valuable tool in many fields, such as audio analysis and biomedical signal processing.
While spectrograms offer substantial advantages, they also come with challenges. For example, determining the right parameters like window size can affect the resulting spectrogram's clarity. Additionally, they might not capture all nuances in the signal, particularly those at very low or high frequencies. Therefore, users must interpret spectrogram data cautiously, ensuring a holistic understanding of the underlying signals.
Advanced Techniques
Principal Component Analysis
Principal Component Analysis (PCA) is a statistical method used to reduce the dimensionality of data while preserving as much variance as possible. It contributes significantly to the spectral domain by enabling the identification of key patterns within complex datasets. The ability of PCA to simplify data makes it a widely appreciated technique in fields such as genomics and finance.
One of the most notable features of PCA is its eigenvalue decomposition, which helps in determining the principal components. By focusing on these components, researchers can effectively reduce noise and enhance the analysis. However, PCA has its disadvantages; for example, it can overlook information that is not aligned with the identified principal components. This shortcoming can lead to missing critical insights if the underlying data structure is not considered.
Independent Component Analysis
Independent Component Analysis (ICA) is another advanced technique that aims to decompose complex signals into statistically independent components. This method plays a crucial role in applications where signals are mixed, such as in biomedical engineering. The key characteristic of ICA is its focus on statistical independence, differentiating it from methods like PCA that prioritize variance.
A unique feature of ICA is that it does not assume that the observed signals are Gaussian distributed, which gives it an edge in identifying hidden factors. Moreover, ICA can reveal information that other techniques might miss, such as specific brain activities in EEG data. However, ICA also has challenges, such as the need for a large amount of data to yield reliable results. Therefore, when using ICA, researchers must ensure that their datasets are adequate to extract meaningful insights.
Understanding these spectral analysis techniques enhances our ability to explore and interpret complex phenomena across various scientific fields.
Understanding these spectral analysis techniques enhances our ability to explore and interpret complex phenomena across various scientific fields.
By comprehending these techniques, researchers can effectively utilize them in their studies. Each technique offers unique strengths and weaknesses, requiring careful selection based on the specific analysis goals.
Applications of Spectral Domain Analysis
The applications of spectral domain analysis span numerous scientific fields, revealing insights that enhance understanding of complex systems. By employing spectral analysis, researchers can dissect signals and data to uncover features that may not be immediately evident in the time domain. This approach leads to improved interpretations in biology, chemistry, physics, and earth sciences. Spectral domain analysis provides advantages such as increased resolution and the capacity to identify periodic trends, facilitating advanced research and development.
Biological Studies
Genomic Data Analysis
Genomic data analysis plays a crucial role in modern biological studies. It allows researchers to examine sequences of DNA and understand variations among genomes. A key characteristic of genomic data analysis is its ability to handle vast datasets, which is essential given the complexity of genomic information. This technique is a beneficial choice for analyzing genetic patterns and identifying mutations related to diseases.
One unique feature is the use of Fourier Transform methods in analyzing gene expression data. This method can reveal periodicities in gene activities, thus identifying biological rhythms that correlate with health conditions. However, genomic data analysis also has its challenges. Errors in sequencing or data processing can lead to misinterpretations. Despite this, its advantages for understanding hereditary traits and genetic disorders make it indispensable in genomics.
Neuroimaging Techniques
Neuroimaging techniques significantly contribute to our understanding of brain activities. They map brain functionalities and structures through various methods, such as fMRI and EEG. These techniques are beneficial for diagnosing neurological disorders and studying brain networks. A key characteristic is the ability to visualize activity in real time, which aids in deciphering complex cognitive processes.
One unique feature of neuroimaging is the use of spectral analysis on EEG data, which can isolate specific brainwave patterns linked to different mental states. This application highlights its advantages, especially in research related to cognitive functions and psychological conditions. Nevertheless, neuroimaging techniques can produce results that require careful interpretation due to factors such as noise in the data or individual variability in brain function.
Chemical Analysis
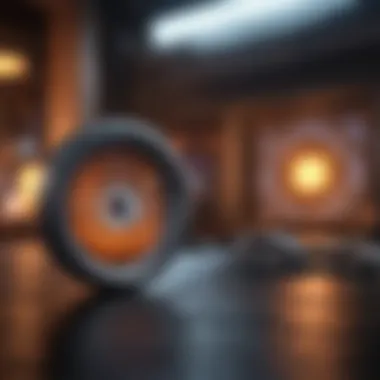
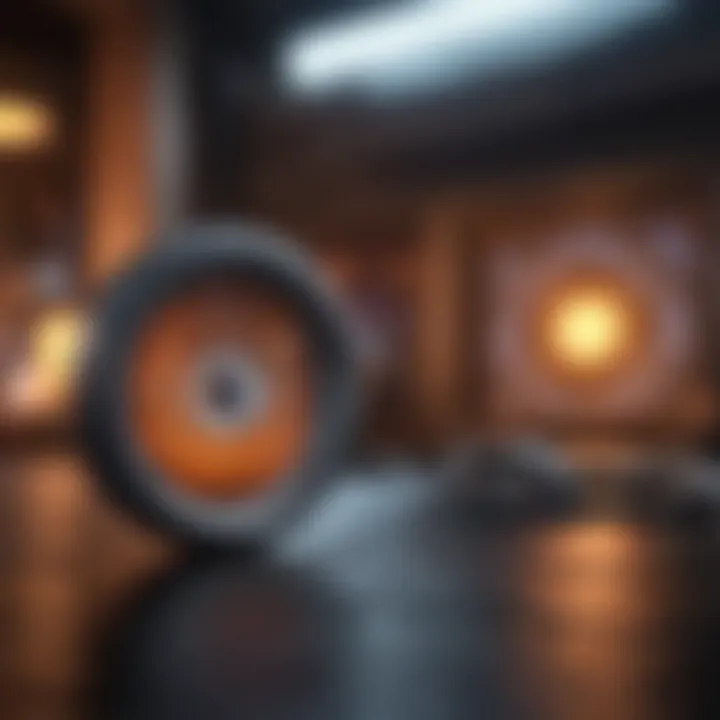
Spectroscopy Techniques
Spectroscopy techniques are vital for chemical analysis, allowing researchers to identify materials and their properties through light interaction. The primary benefit of these techniques is that they provide detailed information about chemical composition and molecular structures. A significant aspect of spectroscopy is its capability to analyze samples without altering their state, thus maintaining sample integrity.
A unique feature of spectroscopy is its ability to distinguish between different molecular entities based on their spectral signatures. This capacity makes it a favored choice for many applications, including identifying unknown substances in forensic science. However, these techniques necessitate rigorous calibration and maintenance. Errors in such processes can lead to misleading results, yet the advantages make spectroscopy fundamental in chemistry.
Mass Spectrometry
Mass spectrometry serves as a powerful tool for identifying chemical compounds by measuring the mass-to-charge ratio of ions. Its contribution to chemical analysis is substantial, especially in determining molecular weights and structures of unknown compounds. A key characteristic is its high sensitivity and specificity, allowing researchers to detect trace levels of substances.
An important feature of mass spectrometry is its application in proteomics, where it identifies and quantifies proteins. This application presents advantages for advancing drug discovery and understanding metabolic pathways. However, mass spectrometry can be complex and requires specialized knowledge for proper data interpretation. Despite these challenges, its efficiency and accuracy make it a favored method in analytical chemistry.
Physical Applications
Quantum Mechanics
Quantum mechanics provides a theoretical framework to understand the behavior of matter on a microscopic scale. This field is pivotal for developing new technologies in computing and materials science. A key characteristic is its ability to describe phenomena that classical mechanics cannot, offering profound insight into atomic and subatomic processes.
One unique aspect of quantum mechanics is the use of quantum Fourier transforms, which facilitate advancements in quantum computing algorithms. This innovation is crucial as it enhances computational speeds exponentially, showcasing its benefits in the realm of physics. However, the inherent complexity and abstract nature of quantum mechanics can pose challenges in practical applications.
Material Characterization
Material characterization refers to the methods used to identify and understand the physical properties of materials. It plays a vital role in fields such as engineering and nanotechnology. A significant characteristic is the diverse range of techniques employed, including X-ray diffraction and electron microscopy, making it flexible for varying research needs.
A unique feature is how spectral analysis can unveil microstructural properties within materials. For instance, using spectroscopic techniques allows researchers to detect defects or impurities. This insight leads to more informed material selection for engineering applications. Despite the precision of these techniques, they can be resource-intensive, requiring careful planning and execution to maximize the potential of findings.
Earth Sciences
Remote Sensing
Remote sensing involves acquiring data about Earth's surface without direct contact. This application is crucial in environmental monitoring and urban planning. A key characteristic is the ability to monitor vast areas in real time, providing essential data for assessing changes in land use or climate.
One unique feature of remote sensing is its integration with spectral analysis, which helps identify materials based on their reflectance properties. This integration is beneficial for mapping natural resources and monitoring environmental changes. However, factors such as atmospheric interference can affect data quality, indicating the need for careful calibration and data processing.
Seismology
Seismology focuses on studying earthquakes and seismic waves to understand Earth's internal structure. Its contribution is critical for disaster preparedness and mitigation strategies. A key characteristic of seismology is its reliance on spectral analysis to interpret seismic data, offering insights into earthquake origins and magnitudes.
A unique aspect is how seismologists use power spectral density to analyze frequency content in seismic signals. This feature is advantageous for distinguishing between different seismic events. Nonetheless, challenges exist, including the interpretation of complex seismic signals that can vary significantly based on geological conditions. The knowledge gained through seismology remains vital for enhancing safety measures in earthquake-prone regions.
Case Studies in Spectral Domain Analysis
The exploration of the spectral domain has yielded various case studies that illustrate its profound impact across different fields. These case studies provide real-world applications and outcomes resulting from spectral analysis, demonstrating both its utility and relevance. They serve as a bridge between theory and practical implementation, showcasing how theoretical concepts can inform and enhance empirical research. Additionally, these cases elucidate the methodologies employed and their implications for future exploration in spectral analysis.
Notable Research Findings
Numerous studies have emerged that utilize spectral domain analysis to yield significant insights. For instance, research in neuroimaging often employs techniques like functional Magnetic Resonance Imaging (fMRI) and Electrocorticography (ECoG) to analyze brain activity patterns. Notable findings have demonstrated how different brain regions engage during complex cognitive tasks, providing a deeper understanding of neurophysiology. These findings not only contribute to the field of neuroscience but also have implications in clinical practices for diagnosing and treating neurological disorders.
Another example can be found in genomics. Spectral analysis helps researchers identify variations in DNA sequences. Through techniques such as Fourier Transform in analyzing genomic data, scientists have been able to pinpoint genetic mutations associated with diseases such as cancer. This illustrates the potency of spectral analysis in making biological discoveries that can affect healthcare outcomes.
"The application of spectral analysis in genomics and neuroimaging underscores its versatility and transformative impact on scientific research."
"The application of spectral analysis in genomics and neuroimaging underscores its versatility and transformative impact on scientific research."
Innovative Applications
Beyond research findings, the applications of spectral domain analysis have reached innovative levels in technology and industry. In the field of material science, techniques such as Raman Spectroscopy exploit the spectral domain to understand material properties at a molecular level. This analysis aids in quality control and the development of new materials in industries ranging from pharmaceuticals to nanotechnology.
In environmental science, spectral domain techniques are increasingly used in remote sensing. Satellite images analyzed through spectral transformation can reveal changes in land use, vegetation health, and pollution levels. This application facilitates the monitoring of environmental changes, guiding policymakers in effective decision-making.
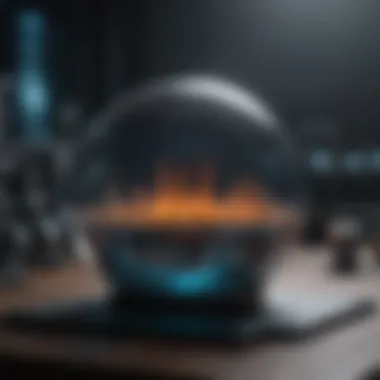
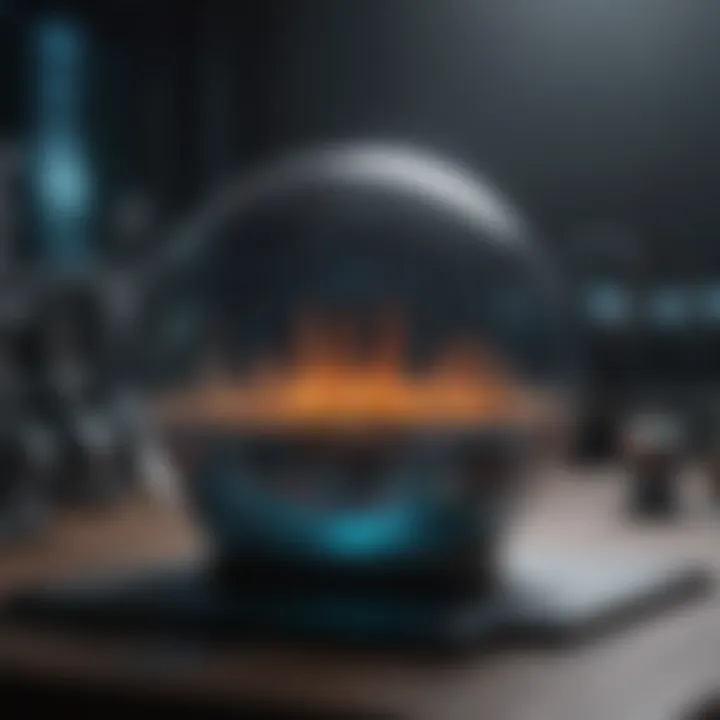
Additionally, in audio processing, techniques such as the Short-Time Fourier Transform allow for the analysis of sound signals. This has implications in music technology and acoustics, enriching our understanding of auditory perceptions.
Challenges and Limitations
The study of the spectral domain encounters various challenges and limitations that can hinder accurate analysis and application. Understanding these challenges is vital for researchers and professionals involved in spectral analysis. Awareness of issues such as data acquisition and result interpretation is fundamental to successfully utilizing spectral analysis tools and technologies. Addressing these challenges helps to improve the reliability of results and the effectiveness of applications in different fields.
Data Acquisition Issues
Data acquisition is a critical aspect of spectral analysis. High-quality data is essential for reliable results, but various factors can complicate this process. One common issue is noise interference, which can distort the spectral signals being analyzed. Sources of noise can range from electronic devices to environmental factors. Noise can mask relevant data, making it difficult to extract meaningful insights.
Moreover, sensor limitations can affect data accuracy. For instance, sensors may not capture all frequencies, leading to gaps in the spectral information. This is particularly significant in applications such as neuroimaging and spectroscopy where precision is crucial.
In addition, the need for large datasets can present a logistical challenge. Collecting sufficient data requires time and resources, which might not always be feasible in every research environment. This can limit the progress in exploring the spectral domain comprehensively.
Interpretation of Results
Interpreting spectral analysis results is another significant challenge. Even with accurate data acquisition, results may not always be straightforward to interpret. The process often involves complex mathematical models and assumptions, which can lead to misinterpretations if not handled correctly.
Another important factor is the subjective nature of some interpretative methods. Different researchers may derive varying conclusions from the same dataset, influenced by their background, expertise, and perspective. This variability can create a lack of consensus in certain findings, complicating the advancement of knowledge in the spectral domain.
To mitigate these challenges, it is essential for researchers to adopt robust statistical methods and ensure rigorous validation of their results. Implementing standardized protocols for data collection and analysis can also enhance the consistency and reliability of interpretations across different studies.
"Recognizing the limitations of spectral analysis is as crucial as understanding its capabilities. It governs the way researchers can apply these methods to their specific fields and influences future developments in the area."
"Recognizing the limitations of spectral analysis is as crucial as understanding its capabilities. It governs the way researchers can apply these methods to their specific fields and influences future developments in the area."
Understanding and addressing these challenges can pave the way for more effective applications and innovations in the spectral domain.
Future Directions in Spectral Analysis
The future of spectral analysis holds significant promise across various scientific fields. By integrating advanced technologies and fostering interdisciplinary collaboration, researchers can deepen their understanding of complex systems and phenomena. This section explores key aspects that may shape the trajectory of spectral domain analyses, providing insights that underscore their potential benefits and the considerations involved.
Emerging Technologies
Emerging technologies are reshaping how spectral analysis is performed. The development of faster computing capabilities is enabling the analysis of larger datasets with increased precision. For instance, machine learning algorithms are being leveraged to better interpret spectral data. These algorithms can identify patterns and anomalies that traditional methods might miss. As computational power continues to enhance, the ability to perform real-time spectral analysis becomes more feasible. This may lead to quicker decision-making in various fields.
Moreover, advancements in sensor technology are revolutionizing data acquisition. High-resolution spectrometers and imaging systems are becoming more accessible. Such instruments provide finer detail in spectral data, which can lead to more accurate analysis. The integration of technologies such as the Internet of Things (IoT) allows for continuous monitoring of environmental changes using spectral techniques. This offers myriad applications in agriculture, environmental science, and health monitoring.
Interdisciplinary Research
Interdisciplinary research is crucial for propelling spectral analysis forward. By crossing traditional discipline boundaries, researchers can combine methodologies from fields such as biology, chemistry, physics, and engineering. This collaborative approach encourages innovative solutions to complex problems. For example, bioinformatics, which melds biology and data analysis, relies heavily on spectral methods to interpret genomic and proteomic data.
Additionally, partnerships between academia and industry foster the real-world application of spectral analysis. Industries like pharmaceuticals utilize spectral techniques for drug development. Collaborations with academic institutions facilitate access to cutting-edge research and mutual benefits from shared findings. This synergy not only enhances empirical data but also drives innovation in spectral domain applications.
"By fostering interdisciplinary research, we can unlock new potential within the spectral domain, paving the way for major scientific breakthroughs."
"By fostering interdisciplinary research, we can unlock new potential within the spectral domain, paving the way for major scientific breakthroughs."
The importance of these elements cannot be overstated. As spectral analysis continues to evolve, a focus on emerging technologies and interdisciplinary research promises to yield insights that were previously unattainable. The exploration of these avenues will undoubtedly enrich the scientific community, offering a comprehensive understanding and new applications of spectral analysis in the years to come.
Culmination
The conclusion serves as a pivotal component of this article, encapsulating the essence of the spectral domain and its multifaceted relevance across diverse scientific fields. Summarizing key insights provides a concise recall of important findings, while simultaneously highlighting the broader implications of these insights for forthcoming research.
Summary of Key Insights
In examining the spectral domain, several critical themes emerge:
- Interdisciplinary Nature: The spectral domain crosses multiple fields including biology, chemistry, physics, and earth sciences, showcasing its versatility in addressing complex scientific questions.
- Fundamental Principles: Techniques such as Fourier and wavelet transforms are core to spectral analysis, allowing for a refined understanding of signals and their frequency components.
- Application Diversity: From genomic data analysis in biological studies to mass spectrometry in chemical analysis, the applications underscore the method's utility in extracting meaningful information from various data types.
- Challenges Identified: Issues in data acquisition and interpretation raise awareness about limitations, prompting further developments in methodologies.
These insights illustrate how engaging in spectral domain analysis can contribute to advancements in different scientific spheres.
Implications for Future Research
The exploration of the spectral domain opens paths for numerous future research directions:
- Technological Integrations: Emerging technologies such as machine learning and artificial intelligence are anticipated to enhance the capabilities of spectral analysis further. Their inclusion may automate processes and increase data processing efficiency, leading to deeper insights.
- Enhanced Collaboration: Interdisciplinary approaches are expected to foster collaboration among researchers from various fields. The synergy created can produce innovative applications and methodologies, enhancing the study of complex systems.
- Long-term Impacts: As quantum computing evolves, its potential implications on spectral analysis will warrant investigation. This transition could redefine current practices and offer new perspectives in data interpretation.