Exploring Face Ethnicity Recognition in Online Spaces
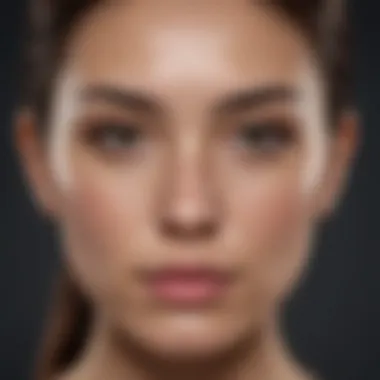
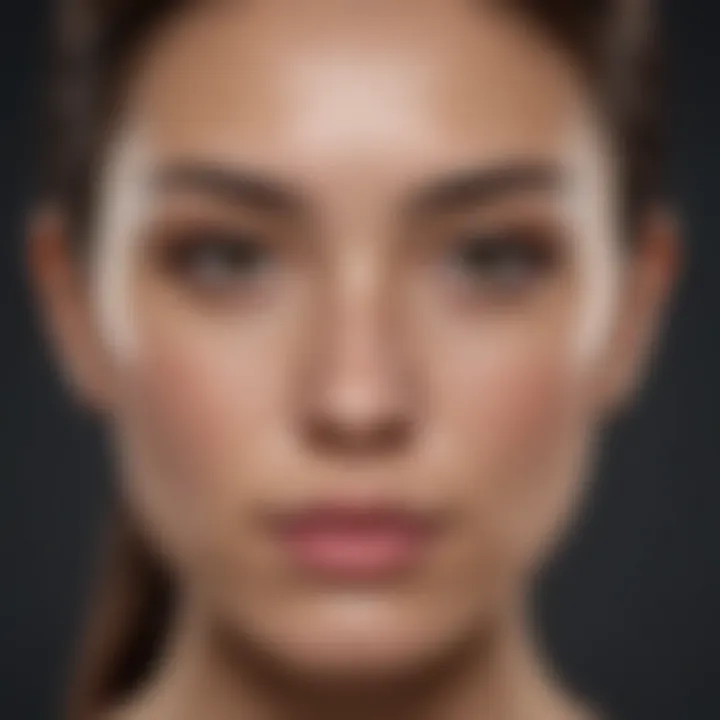
Intro
In today's digital landscape, the exploration of face ethnicity recognition online takes on heightened importance. This technology is not merely a curiosity; it intertwines with questions of identity, ethics, and social justice. As we find ourselves increasingly enmeshed in online environments, how we interpret and react to the diverse features of faces becomes a reflection of wider societal attitudes. The evolution of this technology, along with its implications, shapes both individual and collective experiences.
Article Overview
Purpose of the Article
This article serves to elucidate the various dimensions of face ethnicity recognition via online platforms. The aim is not just to highlight cutting-edge methodologies but to stir a conversation about the ethical ramifications that accompany such technologies. By bridging the gap between innovative technology and societal implications, readers are equipped with an understanding that extends beyond technical details alone.
Relevance to Multiple Disciplines
Face ethnicity recognition doesn't exist in a vacuum; it affects multiple realms.
- Technology: Understanding the algorithms and data influences the advancement of AI technologies.
- Ethics: As we navigate the complexities of social identity, ethical considerations regarding bias and privacy come to the forefront.
- Sociology: The integration of AI into daily lives raises questions about identity and how societies perceive different ethnic backgrounds.
These intersections offer fertile ground for discussion among students, researchers, educators, and professionals.
Research Background
Historical Context
Face recognition technology is not a new buzzword; it has roots tracing back several decades. Initially emerging in the 1960s, early attempts to identify faces relied on rudimentary techniques that often struggled with accuracy. Fast forward to the 21st century, where advancements in machine learning and deep learning have revolutionized this field. With a surge in studies focusing on ethnicity recognition, the dialogue around its utility and consequences has gained momentum.
Key Concepts and Definitions
To understand face ethnicity recognition, it's essential to unpack several key terms:
- Face Recognition: The automated identification or verification of individuals by analyzing facial features.
- Ethnicity Recognition: A subfield dealing specifically with the identification of an individual's ethnic background via facial analysis, often clouded by ethical considerations.
- Algorithmic Bias: This refers to the propensity of algorithms to produce skewed results, often reflecting historical societal biases, which is particularly concerning in ethnicity recognition.
Such concepts will provide the groundwork for a deeper exploration into the narrative that follows, setting the stage for an informed discourse on face ethnicity recognition in online environments.
"The question is not whether machines can think, but whether men do." ā B.F. Skinner
"The question is not whether machines can think, but whether men do." ā B.F. Skinner
In summary, the exploration of face ethnicity recognition in online settings encompasses a vast range of topics that cut across technological, ethical, and sociological landscapes. As we journey through this article, the connection between these elements becomes pivotal to understanding the broader implications of this emerging field.
Prolusion to Face Ethnicity Recognition
Face ethnicity recognition stands at the intersection of technology and society, merging complex algorithms with deep social implications. Understanding this phenomenon is not simply an academic exercise; it echoes in real-world applications, from law enforcement to social media platforms. The significance of diving into the aspects of face recognition technology, especially relating to ethnicity, becomes clear when one considers its vast potential and the challenges it introduces in our increasingly digital world.
Understanding Face Recognition Technology
The crux of face ethnicity recognition hinges on the broader landscape of face recognition technology itself. At its core, this technology utilizes algorithms to analyze an individualās facial features, determining identities based on unique characteristics. Systems can then classify these identities into various categories, including ethnicity. Examples like the algorithms used in Google Photos and Facebookās tagging features illustrate just how entrenched this technology is in daily life.
However, this technology doesn't operate in a vacuum. It's equipped with strengths and weaknesses, nuances that professionals and researchers alike must navigate. One of the primary benefits is efficiency; industries can process vast amounts of data quickly. Yet, it raises critical questions around accuracy and bias. A key point of consideration is how various groups may be favored or misrepresented in the training data used by these systems. Misidentification, in this light, becomes more than just a technical failure; it can perpetuate misunderstandings and stereotypes about different ethnicities.
Defining Ethnicity in the Context of Face Recognition
Defining ethnicity isn't just about checking a box; it's a multi-layered concept that can encompass cultural, social, and biological elements. The challenge arises when that definition is integrated into face recognition systems. Ethnic categories are often overly simplisticālimited to broad classifications that do not account for the rich diversity within groups. For example, the label "Asian" can include a vast array of peoples, customs, and histories, from the Japanese to the Fijians.
When integrating these classifications into technology, such distinctions can lead to oversimplified algorithms that fail to recognize individuality. This can manifest in significant ways, particularly through social identity and its perceived roles.
To understand the full scope of face ethnicity recognition, one must consider how ethnicity is defined and the implications of that definition in algorithmic contexts. Each layerāfrom classification to the implications of those classificationsāplays a critical role in shaping the technology's deployment in society.
"Without a careful understanding of how we define the categories of ethnicity in technology, we run the risk of inadvertently feeding bias back into the system."
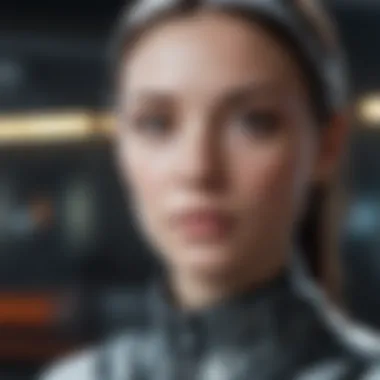
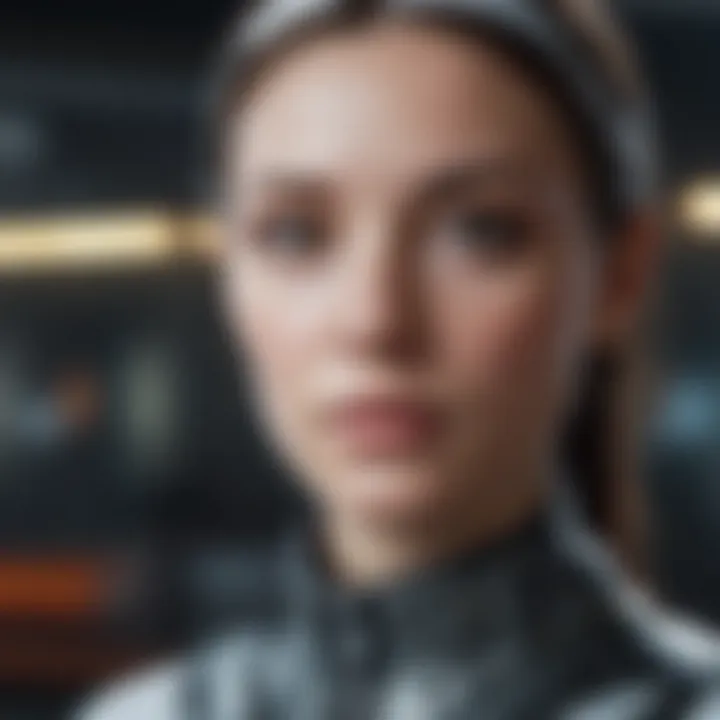
"Without a careful understanding of how we define the categories of ethnicity in technology, we run the risk of inadvertently feeding bias back into the system."
As we delve deeper into this exploration, we'll look into the technological framework that supports these systems, alongside the ethical considerations that arise as bureaucracies utilize them. The exploration is vital in framing future inquiries into face ethnicity recognition and its role in contemporary society.
Technological Framework
The Technological Framework plays a pivotal role in understanding the dynamics behind face ethnicity recognition. It encapsulates the algorithms and computational methods that drive the technology. This framework not only highlights the technological elements at play but also draws attention to the benefits and considerations that come with their application in real-world scenarios. Recognizing faces based on ethnicity requires more than mere identification; it involves nuanced recognition of features influenced by cultural backgrounds and physical characteristics.
With the rise of artificial intelligence, there's a growing reliance on sophisticated techniques that can analyze vast datasets to improve accuracy in ethnicity recognition. But the framework also brings to light critical conversations about trustworthiness and ethics. Decision-makers and developers need to consider how these technologies can be employed responsibly.
Machine Learning Algorithms in Ethnicity Detection
Machine learning algorithms have become the cornerstone of ethnicity detection. These algorithms, which include decision trees, support vector machines, and k-nearest neighbors, work by analyzing patterns found in facial features. They learn from pre-labeled images and build models that can classify new images based on learned traits.
By employing various techniques, machine learning algorithms can take large volumes of data and distill them into insights that can improve recognition accuracy. However, successful implementation hinges on the choice of appropriate datasets for training these algorithms. The right datasets enable more nuanced learning, while poor choices can lead to biases and inaccuracies.
Deep Learning Techniques and Their Applications
Moving beyond traditional methods, deep learning techniques further enhance ethnicity recognition. Utilizing neural networks, especially convolutional neural networks (CNNs), deep learning processes vast amounts of visual data and can extract more intricate features from images than their predecessors.
These techniques are not only beneficial for identifying ethnic traits but also for discerning subtleties like expressions, lighting conditions, and angles which play an essential role in recognition accuracy. As deep learning continues to evolve, its applications keep expanding, leading to more effective solutions in areas like security and user interface personalization.
Data Collection and Dataset Challenges
The backbone of any recognition technology lies in its data collection strategies. It's crucial to acknowledge the challenges surrounding dataset collection that impact the effectiveness of ethnicity recognition technologies.
Public vs. Private Datasets
In exploring Public vs. Private Datasets, one finds that each type contributes uniquely to the overall effectiveness of ethnicity detection systems. Public datasets are often lauded for their accessibility and the breadth of diversity they encompass. They typically consist of thousands of images available for research and development purposes.
On the flip side, private datasets may provide more controlled environments and targeted demographics, but often come with restrictions that can stifle comprehensive analysis. A key characteristic of public datasets is their availability, which allows researchers and developers to circumvent some financial barriers, thus promoting more inclusive technological advancements.
Bias in Dataset Composition
The challenge of Bias in Dataset Composition looms large in the domain of ethnicity recognition. This aspect illustrates how the datasets used can radically influence the effectiveness of recognition algorithms. Datasets that lack diversity can lead to biased outcomes, where certain ethnic groups may be overrepresented while others are entirely neglected.
An important factor to note here is how bias can creep into both the composition of datasets and the resultant algorithms. These biases not only skew results but can also reinforce racial stereotypes, raising serious ethical concerns about the impact of such technologies in society. The implications of using biased datasets stretch far beyond technical flaws; they penetrate social dynamics, affecting public perception and trust in such systems.
Ultimately, addressing these issues invites a deeper exploration of our ethical compass in technology deployment and acceptance.
Ethical Considerations
The rise of face ethnicity recognition technology brings forth a myriad of ethical challenges that require careful scrutiny. These considerations are paramount not only in technology development but also in its application. The importance of ethical considerations in this domain hinges on the need to protect individual rights and promote fairness while leveraging technological advancements. This section aims to dissect key elements of ethical implications, focusing on bias, social identity, and privacy.
Addressing Bias and Inaccuracy
Face ethnicity recognition systems are often criticized for their inherent biases. Thereās a growing body of research indicating that these systems can misidentify individuals based on their ethnic background. Often, these biases stem from the datasets used to train recognition algorithms. If these datasets lack diversity, it results in algorithms that perform poorly on underrepresented groups. This failure is not merely a technical shortcoming; it can lead to serious consequences in real-world situations, such as wrongful arrests or exclusion from opportunities.
To combat these biases, developers must emphasize the necessity of more inclusive datasets. An ethically responsible approach requires teams to actively seek data that represent diverse populations. Furthermore, ongoing audits of algorithm performance on various ethnicities should be standard practice. The implications of ignoring this can ripple across societal structures, exacerbating existing inequalities and perpetuating stereotypes.
"The effectiveness of face ethnicity recognition technology hinges on its commitment to fairness and accuracy. Without these, the potential benefits become overshadowed by risks and inaccuracies."
"The effectiveness of face ethnicity recognition technology hinges on its commitment to fairness and accuracy. Without these, the potential benefits become overshadowed by risks and inaccuracies."
Impact on Social Identity and Stereotyping
Social identity plays a crucial role in shaping individual experiences and perceptions. The ability of technology to categorize individuals based on perceived ethnicity has far-reaching implications for social dynamics. When algorithms pigeonhole users into ethnic categories, it risks reinforcing harmful stereotypes that can affect how people are treated in society.
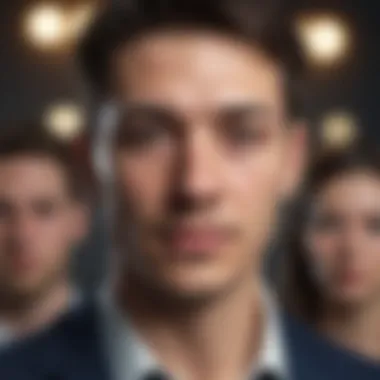
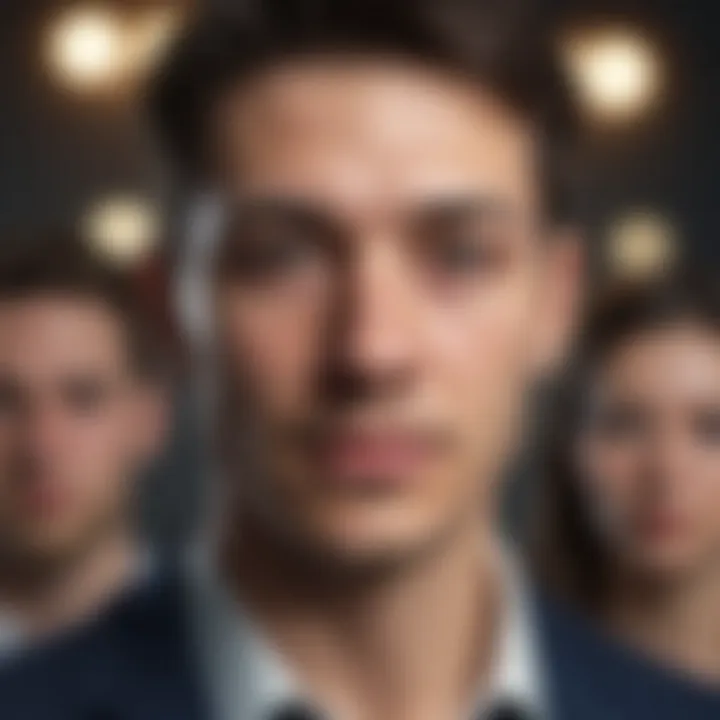
Moreover, the implications of such categorization extend to identity itself. People may feel confined by the identities technology assigns to them, leading to questions about self-perception and representation. For communities that have historically faced oppression, reliance on automated recognition systems can deepen feelings of marginalization. Thus, it is vital to recognize how these tools can unintentionally reinforce social divides rather than promote understanding.
Privacy Implications of Recognition Technologies
In an age where personal data is increasingly commodified, privacy concerns regarding face ethnicity recognition technologies cannot be overstated. The deployment of these systems often occurs without comprehensive consent from individuals being identified. This lack of transparency about who is using the technology, and for what purposes, leads to significant trust issues between communities and organizations.
Furthermore, the risk of surveillance looms large. When face recognition technology is paired with mass data collection, it puts at risk not only personal freedom but also the integrity of communities. Thereās a need to establish regulations that ensure privacy rights are upheld. Itās essential to foster public discourse on these matters, making it clear that individuals' rights should not be sacrificed at the altar of innovation.
Applications and Use Cases
The realm of face ethnicity recognition finds a host of applications and use cases that extend into various sectors of society. This technology is not just a futuristic gimmick; it is shaping security measures, social connectivity, and even marketing strategies today. Understanding these applications helps elucidate the impact of ethnicity recognition on our lives, guiding discussions on its ethical use and societal implications.
Surveillance and Law Enforcement
In the domain of law enforcement, face ethnicity recognition has emerged as a vital tool. Police departments across the globe are increasingly leveraging this technology to enhance safety and reduce crime rates. For instance, facial recognition can help identify suspects in real-time, aided by bodies of software that sift through databases of known criminals. This capability is particularly beneficial in urban areas where the density of people complicates traditional surveillance methods.
However, while the advantages seem compelling, concerns about bias bloom like wildflowers in spring. Studies have shown that these recognition systems may struggle with accuracy across diverse ethnic groups, leading to disproportionate targeting of certain minorities. Is it a coin flip between effective policing and social justice? The implications on community dynamics are profound, raising an urgent need for scrutiny and oversight in deploying such technologies.
Social Media and Online Platforms
The intersection of social media and face ethnicity recognition stands as a double-edged sword. Platforms like Facebook and Instagram utilize facial recognition to improve user experiences, such as tagging and enhancing connectivity. On one hand, this feature broadens user engagement and fosters a sense of belonging; on the other, it raises questions about consent and privacy. People may not fully realize how their data is used or shared, prompting a need for clear communication from tech companies about their practices.
Moreover, the role of ethnicity recognition in mapping social connections warrants careful consideration. Users might find themselves categorized based on their appearances, sometimes exacerbating existing prejudices rather than alleviating them. Moving forward, platforms must strike a balance: maximizing user engagement while safeguarding ethical standards.
Marketing and User Experience Enhancement
In marketing, face ethnicity recognition is transforming the strategies companies utilize to connect with their audiences. Brands are now able to tailor content based on ethnic backgrounds, allowing for personalized advertisements that resonate more deeply with specific demographics. For example, a campaign featuring culturally relevant products is likely to hit home with consumers who see themselves reflected in the messaging.
However, there's a thin line here; while targeted marketing can enhance user experience, it also risks stereotyping. When brands paint broad strokes based on ethnicity, they can inadvertently alienate potential customers who don't feel represented. Brands must tread carefully, ensuring their marketing strategies embrace diversity without falling into the pit of oversimplification.
For instance, instead of just relying on visual cues, companies can use a more holistic approach to understand their users' preferences, leading to better engagement and customer loyalty.
"Technology is a tool, but its true power lies in the ethical framework guiding its use."
"Technology is a tool, but its true power lies in the ethical framework guiding its use."
In summary, the application of face ethnicity recognition spans several significant areas, each laden with its own set of benefits and challenges. As we venture into a future increasingly intertwined with this technology, it is essential to actively engage in discussions about how we can use these tools for the greater goodāensuring they enhance rather than hinder social values.
Technical Limitations and Challenges
The realm of face ethnicity recognition online is not without its hurdles. While the technology boasts impressive advancements, a number of technical limitations can hinder its efficacy and reliability. Understanding these limitations is critical, as they inform both the capabilities and shortcomings of various systems in use today. Exploring the topic of technical limitations helps clarify the pathway forward in this evolving field, highlighting the essential considerations that must be addressed moving forward.
Variability in Facial Features Across Ethnicities
Face recognition technology hinges heavily on the distinct features that define various ethnic groups. However, the facial features of individuals can be profoundly variable, even within the same ethnic group. Factors such as age, gender, and regional background amplify this variability.
Some common challenges include:
- Diversity in Facial Structures: Bone structure, skin tone, and facial hair can vary so much that algorithms struggle to accurately identify individuals.
- Lighting Conditions: The ability of systems to perform well under different lighting conditions is crucial, but many fail when faced with shadows or glare.
- Cultural Practices: Different ethnicities may have varying grooming patterns, like the use of headscarves or facial tattoos, which complicate the recognition process.
The intricacies of the human face make it paramount for developers to consider a diverse training set that accurately reflects these differences, otherwise, any system will likely miss the mark.
Real-time Processing Constraints
Real-time processing has been a buzzword in technology circles, alluring consumers and businesses alike with the promise of instantaneous feedback. However, achieving this in the context of facial recognition comes with its own set of challenges.
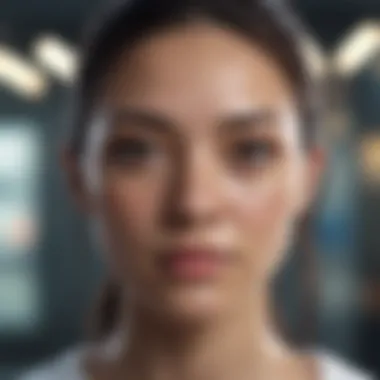
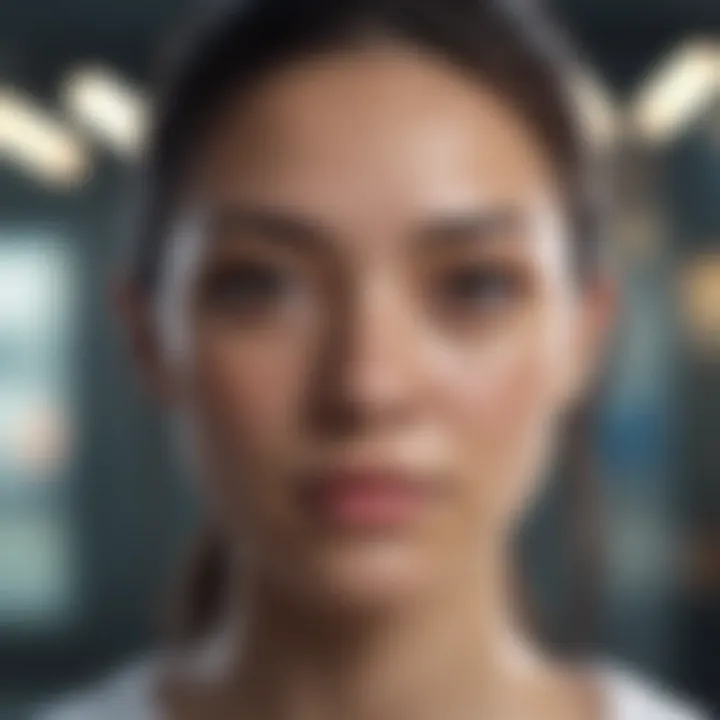
Some of the key issues faced include:
- Computational Limits: Advanced algorithms require significant processing power, which is often not available in low-end devices. Without the right hardware, users may experience lags or failures.
- Network Latency: For many online applications, a delay in data transmission can lead to inconvenience, giving a poor user experience. In time-sensitive situations, real-time recognition may become impractical.
- Algorithm Complexity: More advanced algorithms often mean a more complex implementation, which can detract from both speed and efficiency.
In summary, while the dream of real-time face ethnicity recognition is tantalizing, it casts a spotlight on the pressing need for innovative solutions that can effectively bridge the gap between great promise and substantial limitations.
">Recognizing the limitations of technology is essential for progress. Effective solutions arise not from ignoring challenges, but by addressing them head-on."
">Recognizing the limitations of technology is essential for progress. Effective solutions arise not from ignoring challenges, but by addressing them head-on."
By thoroughly inspecting these technical limitations, we can prepare better for future developments that may enhance the capabilities of face ethnicity recognition systems and ensure they are equipped for real-world application.
Future Directions
The future direction of face ethnicity recognition technology stands as a pivotal element in the ongoing discussion of its implications in online spaces. With rapid advancements in artificial intelligence, the pathways we traverse not only shape the functionalities of technologies but also their societal impact. As we look ahead, it's crucial to analyze how these technologies evolve, the regulatory landscapes that shape them, and the perceptions of the public about their use. This underscores the notion that technological advancements must be matched with ethical considerations, ensuring a responsible approach to implementation and application.
Advancements in AI and Facial Recognition
Recent strides in artificial intelligence have significantly influenced the capabilities of facial recognition systems. From improved image processing through convolutional neural networks to enhanced accuracy in image classification, the developments are noteworthy. Take, for example, how systems now rely on extensive datasets with diverse images, enabling better recognition across various ethnic backgrounds, reducing the risk of bias, and improving overall performance.
These advancements are not just a product of technical innovation; they also aim to address the growing concerns surrounding bias and fairness in AI systems. Moreover, the integration of machine learning techniques enables systems to adapt and learn from their mistakes over time, thus refining their accuracy. This becomes particularly important in the context of ethnicity recognition, where misidentification can lead to serious social and ethical repercussions.
Evolving Regulations and Standards
As facial recognition technology becomes more integrated into our everyday lives, the regulatory standards governing its use are evolving as well. Countries and states are grappling with how best to legislate these technologies, balancing the need for security against individual privacy rights. For instance, regions like the European Union have begun developing comprehensive regulations that govern the use of AI, particularly focusing on applications that could infringe upon personal freedoms or perpetuate discrimination.
These evolving regulations are gaining notice from industry leaders who are advocating for clearer guidelines that ensure safe and ethical uses of these technologies. A unified approach to regulations can set a foundation for responsible usage and foster public trust, which is vital for the longevity of these systems. The involvement of various stakeholders, including policymakers, rights groups, and tech companies, is essential in creating standards that reflect societal values while addressing potential risks.
Public Perception and Acceptance
Public perception plays a critical role in the deployment and effectiveness of face ethnicity recognition technologies. As society becomes more aware of the potential pitfallsāsuch as privacy concerns, data breaches, and algorithmic biasāthe acceptance of these technologies often hinges on transparency and accountability from tech companies. Trust can be a fragile thing; one breach can shatter public confidence.
Education around how these systems work and their potential benefits could help shift public opinion in a positive direction. Engaging in open dialogue, perhaps through forums like Reddit or community meetings, may provide space for people to express their concerns and learn about the technology's improvements over time.
Furthermore, media representation and narratives surrounding these technologies can shape public understanding and acceptance. Misinformation and sensationalism can lead to heightened anxiety or backlash, whereas factual reporting can foster an informed public that is more receptive to continued innovations in the field.
"The way people perceive technology often dictates its adoption. Thus, clearing the fog of misunderstanding is crucial for advancing meaningful dialogue and acceptance."
"The way people perceive technology often dictates its adoption. Thus, clearing the fog of misunderstanding is crucial for advancing meaningful dialogue and acceptance."
In summary, as we explore future directions in face ethnicity recognition, it becomes apparent that advancements in technology must go hand-in-hand with a robust regulatory framework and mindful public engagement. As the landscape continues to evolve, fostering an environment of trust and accountability could prove beneficial for everyone involved.
The End
As we draw our exploration to a close, itās vital to reflect on the significance of face ethnicity recognition technology. This area not only melds advanced computational methodologies with societal needs but also raises critical questions about fairness, accuracy, and ethics. Technologies that recognize facial features linked to ethnicity have profound implications for various sectors, such as law enforcement, marketing, and social media. However, the potential for misuse and the ethical dilemmas it introduces cannot be overlooked.
Summarizing Key Findings
Throughout this article, we've traversed the complex landscape of face ethnicity recognition. The key findings can be summarized as follows:
- Technological Developments: Machine learning and deep learning have tailored algorithms that can identify features across different ethnicities, showcasing remarkable advancements but still facing challenges related to bias and accuracy.
- Ethics and Bias: The ethical ramifications of deploying these technologies urge stakeholders to address inherent biases. Discriminatory outcomes can emerge from flawed datasets or algorithms, emphasizing the need for ethical frameworks.
- Applications and Concerns: While there are significant applications in fields like surveillance and marketing, thereās an ongoing conversation about privacy rights and societal implications. Understanding these dynamics is crucial for rebuilding trust in technology.
The Path Forward for Ethnicity Recognition Technology
Looking ahead, the future of face ethnicity recognition technology is fraught with both promise and caution. Here are some strategic considerations for its evolution:
- Advancing Algorithms: There's a pressing need for developing more nuanced algorithms that not only focus on accuracy but also incorporate ethical considerations. Researchers must address biases in datasets more explicitly.
- Regulatory Frameworks: Evolving regulations around data privacy and ethical usage will be essential. Policymakers must step up to ensure that technologies are implemented transparently and responsibly.
- Public Discourse: Engaging the public in conversations about these technologies is vital. Awareness and education can foster a better understanding of the benefits and risks, ultimately paving the way for greater acceptance of advanced technology.
It is crucial that as we venture into a future populated by intelligently driven technologies, we remain vigilant, responsible, and engaged.
It is crucial that as we venture into a future populated by intelligently driven technologies, we remain vigilant, responsible, and engaged.
In summation, the trajectory for face ethnicity recognition technology is intertwined with its capacity to adapt to the ethical landscape while providing meaningful contributions to society. As we navigate this complex interplay of technology and humanity, ongoing dialogue and conscious implementation will shape a more equitable future.