The Importance of Low Noise in Scientific Research

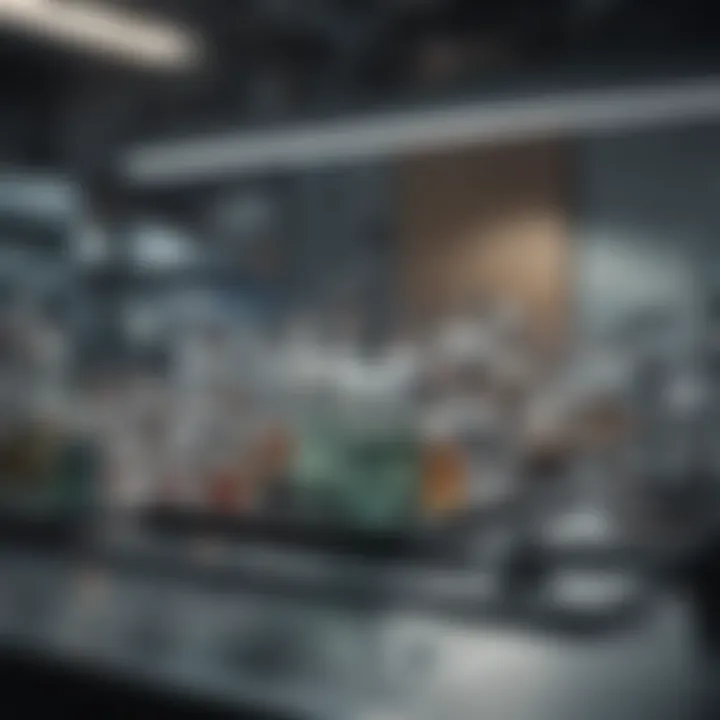
Article Overview
Purpose of the Article
Low noise is a term that encapsulates an essential aspect of scientific research. This article aims to elucidate its significance across different disciplines, helping to understand its definitions, sources, and the profound impact it has on experimental outcomes. The necessity of minimizing noise in scientific environments cannot be overstated; it is vital for ensuring precise measurements, maintaining data integrity, and fostering reliability in scientific discoveries. The intricacies of low noise environments will be examined alongside methodologies to achieve them.
Relevance to Multiple Disciplines
Low noise holds relevance in various fields, from biology and chemistry to physics and earth sciences. Each discipline faces unique challenges posed by noise, yet the consequences can be universally detrimental to the integrity of scientific inquiry. For instance, biologists depend on accurate measurements to assess microscopic changes, while chemists require precise concentrations in reactions. Physicists analyze intricate data that could mislead results if noise is not managed. Understanding noise becomes vital in developing effective solutions to uphold the standard of scientific research.
Research Background
Historical Context
The historical perspective of noise in scientific research provides insight into how it has evolved as a concept. Early experiments often overlooked noise factors, leading to questionable results. Over time, researchers began to identify and address noise sources, paving the way for modern experimental design. This journey highlights the ongoing quest for precision, illustrating a fundamental shift in scientific methodology.
Key Concepts and Definitions
It is crucial to define noise in the context of scientific research. Noise refers to any unwanted disturbances that can interfere with measurements or observations. It can arise from various sources, including:
- Environmental factors: These include temperature fluctuations, electromagnetic interference, and vibrations.
- Instrumental noise: This arises from the equipment used in experiments, such as sensors and measuring devices.
- Human factors: These are errors or biases introduced by the researchers themselves during data collection.
Understanding these definitions enables researchers to identify noise sources critically and consider methods to mitigate their impact.
"Managing noise in scientific research is not merely a technical requirement; it reflects the broader quest for integrity in the discovery process."
"Managing noise in scientific research is not merely a technical requirement; it reflects the broader quest for integrity in the discovery process."
By emphasizing the significance of low noise, this article highlights best practices and methodologies that researchers can apply to enhance accuracy and reliability in their investigations.
Defining Low Noise
Understanding low noise is crucial for scientific inquiry. It serves as a foundation upon which reliable experiments and analyses are built. When noise levels are minimized, researchers can achieve more accurate results and better insights from their work.
Conceptual Framework
Low noise refers to the reduction of unwanted fluctuations that can obscure important signals within scientific measurements. In various fields such as physics and biology, these unwanted variations can manifest in different ways. Therefore, establishing a clear framework allows researchers to identify sources of noise and comprehend its impact on their data.
In a broader sense, low noise encompasses a range of practices and technologies. Researchers must be knowledgeable about both the theoretical and practical aspects of noise control. This knowledge enables them to design experiments that can produce meaningful and trustworthy results.
Importance in Research
The significance of low noise in research cannot be overstated. It affects several key areas:
- Data Accuracy: With minimized noise, data collection becomes more reliable. High accuracy leads to robust conclusions, which are essential for validating scientific hypotheses.
- Reproducibility: Scientific findings must be reproducible by independent researchers. Low noise environments are critical to achieving consistent results across experiments and studies. This reliability enhances the credibility of scientific research.
- Enhanced Discovery: Low noise can enable the detection of subtle phenomena that would otherwise go unnoticed. In fields such as molecular biology, the ability to discern minor variations can lead to significant breakthroughs.
Research often hinges on the clarity of the data collected. High noise levels can distort this clarity, leaving researchers with uncertain outcomes and conclusions.
Research often hinges on the clarity of the data collected. High noise levels can distort this clarity, leaving researchers with uncertain outcomes and conclusions.
Overall, understanding and implementing low noise principles is essential for the integrity and advancement of scientific research.
Sources of Noise in Scientific Research
Understanding the sources of noise is paramount in scientific research. Noise can adversely affect the outcomes of experiments and lead to erroneous conclusions. By identifying and addressing these sources, researchers can enhance the reliability of their findings. This section dissects two primary categories of noise: environmental factors and instrumental contributions.
Environmental Factors
Environmental factors encompass a range of influences outside the experimental setup. These may include temperature fluctuations, humidity levels, electromagnetic interference, and vibrations from nearby equipment or foot traffic. Each of these elements can introduce unwanted variability in measurements. For instance, temperature changes can cause thermal expansion in materials, affecting precision in instruments. Similarly, electromagnetic interference can distort signals in electronic devices, complicating data interpretation.
Managing such environmental variables involves careful control and monitoring. Implementing sound insulation and temperature regulation can mitigate some of these impacts. Additionally, isolating experiments from vibrations and ensuring proper grounding of equipment are effective strategies. Conducting experiments in controlled environments, such as dedicated laboratories, further helps maintain the integrity of data.
A well-prepared environment can reduce noise levels significantly, enabling researchers to focus on the actual phenomena being studied.
A well-prepared environment can reduce noise levels significantly, enabling researchers to focus on the actual phenomena being studied.
Instrumental Contributions
Instrumental contributions refer specifically to the noise introduced by the instruments themselves. This can originate from a variety of sources within the equipment used for measurements. For instance, electronic devices may produce thermal noise due to the movement of charge carriers. Additionally, mechanical noise might arise from moving parts in machinery, such as centrifuges or pumps.
A common issue in many experiments is the signal-to-noise ratio. When the noise level exceeds the desired signal, it complicates the task of discerning meaningful data. To combat this, researchers may calibrate and test equipment meticulously before conducting experiments. They often utilize advanced noise reduction techniques, such as using low-noise amplifiers or filters, to minimize the interference caused by instruments.
To navigate these challenges, laboratory protocols should prioritize instrument quality and maintenance. Employing high-quality, well-calibrated instruments reduces their contributions to overall noise. Regular training sessions for researchers on equipment handling can also enhance data integrity.
In summary, awareness of instrumental noise and its sources is crucial in designing reliable experiments. Addressing these issues can significantly improve accuracy and reproducibility in scientific research.
Types of Noise Affecting Experiments
Understanding the types of noise that can impact experiments is crucial for researchers. Noise can obscure signals in data, leading to inaccurate results or failed experiments. By recognizing specific types of noise, scientists can develop strategies to minimize their effects, thus preserving data integrity. The exploration of types of noise, including thermal and quantum noise, allows for greater precision in scientific outcomes.
Thermal Noise
Thermal noise, also known as Johnson-Nyquist noise, is generated by the random motion of charge carriers in a conductor at any temperature above absolute zero. This phenomenon is significant because it sets a fundamental limit on the performance of electronic circuits and measurement devices. In experiments that rely on precise electronic measurements, thermal noise can obscure genuine signals, particularly in low-voltage applications.
Reducing thermal noise is essential in sensitive measurements such as those found in physics and engineering research. Strategies to mitigate thermal noise include:
- Using low-resistance components: Selecting resistors with lower resistance can contribute to minimizing thermal noise, as thermal noise power increases with resistance according to the formula:
[ P = 4kT/R ]
Where ( P ) is the noise power, ( k ) is Boltzmann's constant, ( T ) is the absolute temperature, and ( R ) is resistance. - Cooling the components: Reducing the temperature of the components can dramatically decrease the systemic thermal fluctuations. Cryogenic methods are employed in advanced laboratories.
By employing these techniques, researchers can improve the quality of their data. This development is particularly necessary in fields like astrophysics and quantum computing, where even minor errors can cascade into substantial inaccuracies.
Quantum Noise
Quantum noise arises from the inherent uncertainties governed by quantum mechanics. This type of noise is present in measurements that involve quantum states. Quantum noise has a profound effect on processes, especially in fields like quantum optics and superconducting devices.
One of the primary aspects of quantum noise is its relation to the Heisenberg Uncertainty Principle. This principle states that certain pairs of physical properties, like position and momentum or energy and time, cannot be simultaneously known to arbitrary precision. In experiments, this inherent uncertainty can limit the resolution and accuracy of measurements.
To manage quantum noise, researchers can adopt several methods:
- Entanglement: Utilizing quantum entangled states can enhance noise performance. When two particles are entangled, measuring one immediately impacts the other, allowing for more precise measurements.
- Quantum feedback control: Implementing feedback mechanisms can minimize errors caused by quantum noise by adjusting the system in real-time based on measurement outcomes.
As quantum technologies continue to advance, understanding and mitigating quantum noise will be increasingly important. By doing so, the scientific community can unlock new avenues for exploration and enhance the reliability of quantum-based experiments.
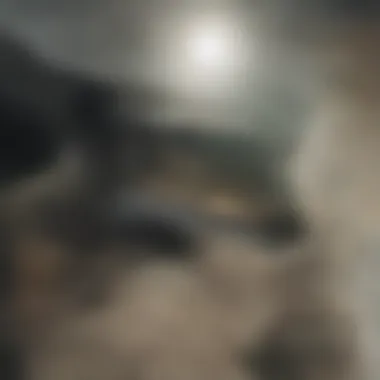
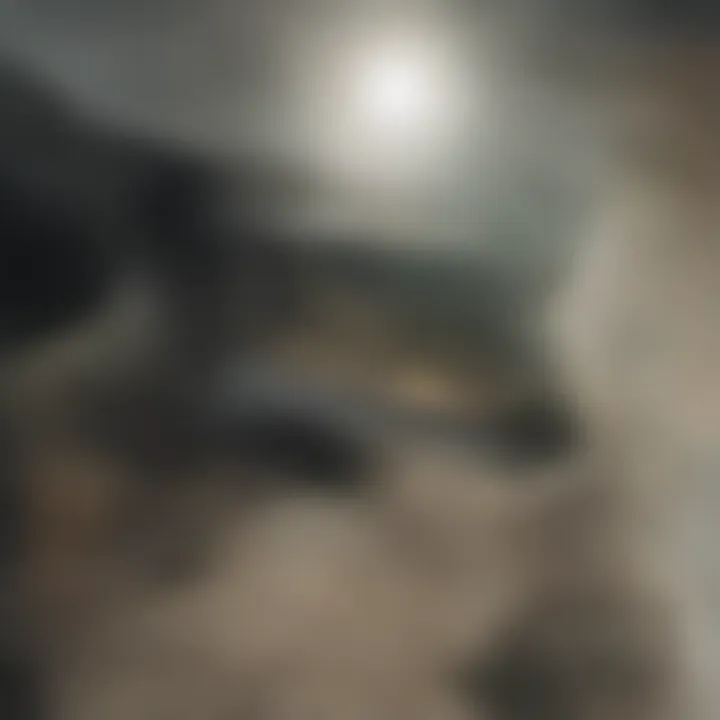
In summary, both thermal and quantum noise present significant challenges in scientific research. Addressing these forms of noise can improve experimental accuracy and reliability, laying the groundwork for future scientific breakthroughs. The pursuit of low noise environments will ultimately lead to enhanced precision and data integrity in various research fields.
Impact of Noise on Data Integrity
Noise in scientific research poses significant challenges to the integrity of data collected during experiments. High levels of noise can obscure underlying signals, resulting in compromised results that question the validity of findings. Thus, understanding the importance of noise reduction is crucial for researchers. Maintaining data integrity is not just about accuracy but also about ensuring that conclusions drawn are reliable and reproducible.
Data Accuracy
Data accuracy refers to the closeness of measurements to the true value. When noise levels are high, the fidelity of data can be distorted. For example, in a biological experiment measuring enzyme activity, random fluctuations may steer researchers toward incorrect conclusions regarding enzyme behavior.
To enhance data accuracy, several strategies can be implemented:
- Calibration of Instruments: Regularly calibrating instruments can help minimize systematic errors due to noise.
- Controlled Environments: Conducting experiments in environments with controlled temperature and humidity can reduce the influences of external noise.
- Advanced Signal Processing: Utilizing algorithms designed to filter out noise can significantly improve data accuracy.
By focusing on the mechanisms that drive accuracy, researchers can safeguard the integrity of their findings, thus enhancing the confidence in published results.
Reproducibility Challenges
Reproducibility is a cornerstone of scientific research. Without reproducibility, results lose their significance. When noise is present, it often leads to differing outcomes in repeated studies even when conducted under ostensibly similar conditions. This variability raises serious concerns about the reliability of scientific claims.
Factors contributing to reproducibility challenges include:
- Variations in Noise Levels: Different labs may experience varying noise levels influenced by equipment and location.
- Subjectivity in Data Interpretation: High noise levels can lead scientists to interpret data differently, resulting in inconsistent conclusions.
- Inconsistent Methodologies: When protocols are not standardized across laboratories, noise can play a decisive role in the variability of results.
To mitigate the impact of noise on reproducibility, researchers should adopt rigorous methodological standards. This includes:
- Standardized Protocols: Establishing clear, consistent operating procedures for experiments.
- Shared Data Repositories: Encouraging the use of common platforms for data storage can help verify and validate results across different teams.
Ensuring data integrity through noise management strengthens the foundation upon which scientific knowledge rests.
Ensuring data integrity through noise management strengthens the foundation upon which scientific knowledge rests.
By addressing data accuracy and reproducibility challenges, the entire scientific community benefits, allowing for more trustworthy advancements and innovations. Understanding noise's implications is not just a matter of improved measurements; it is about upholding the core values of scientific inquiry.
Strategies for Minimizing Noise
In scientific research, implementing effective strategies to minimize noise is essential for enhancing the quality and reliability of experimental data. Noise can obscure true signals and lead to erroneous conclusions, making it imperative to consider noise reduction from the outset of research design. This section discusses the key elements associated with minimizing noise, including careful planning, thoughtful design, and practical techniques that researchers can employ to create optimal environments for their experiments.
Experimental Design Considerations
Designing experiments with low noise in mind requires a comprehensive approach that considers various factors. Researchers must prioritize several design elements:
- Site Selection: Choosing the right location for experiments is crucial. Laboratories should be located in areas with minimal external disturbances such as traffic noise or electromagnetic interference.
- Controlled Environment: Maintaining constant temperature and humidity levels is vital, particularly in sensitive experiments. Variations in environmental conditions can introduce unwanted noise into measurements.
- Instrument Calibration: Regular calibration of equipment helps ensure that instruments maintain a high level of accuracy. This reduces the chances of noise originating from malfunctioning or miscalibrated instruments.
When researchers integrate these considerations into their experimental design, they create a foundation for acquiring cleaner data. This, in turn, increases the accuracy and reliability of their results.
Noise Reduction Techniques
Several specific techniques exist for reducing noise during experiments. Implementing these methods can significantly enhance data integrity:
- Shielding: One effective approach involves shielding sensitive equipment from electromagnetic noise. Using Faraday cages or other shielding methods can create low-noise environments.
- Isolation: Utilizing vibration isolation tables helps minimize mechanical noise. This is important in studies that involve precise measurements, such as microscopy.
- Signal Processing: Techniques such as averaging multiple measurements can help in distinguishing true signals from noise. Digital filters can also aid in isolating relevant data from unwanted interference.
Researchers should continuously evaluate and employ a combination of these techniques depending on the specific context of their work. The specific solutions may vary according to the challenges faced in noise management across different research scenarios. Low noise strategies must be adaptable to the needs and constraints of particular experiments, ensuring ongoing improvements in data quality.
In summary, the significance of minimizing noise cannot be overstated in scientific research. By focusing on experimental design considerations and employing effective noise reduction techniques, researchers can foster environments that enhance data integrity and the overall quality of scientific inquiry.
Low Noise Environments in Various Disciplines
Low noise environments are crucial across a variety of scientific fields. By minimizing unwanted signals and disturbances, researchers can obtain data that is more reliable and precise. This section will focus on the specific elements and benefits of low noise conditions in biological research and physical sciences, illustrating how they contribute to advanced discoveries and technological developments.
Biological Research Applications
In biological research, maintaining a low noise environment is essential for several reasons. First, many biological processes operate on very fine scales. For instance, cellular responses and molecular interactions can occur in nanoseconds. Noise in these environments can easily obscure genuine reactions, leading to misinterpretations of results.
Moreover, various techniques, such as fluorescence microscopy or single-cell sequencing, rely heavily on low noise conditions. High-quality imaging is necessary to observe cellular behaviors accurately. If noise levels are too high, it could result in blurred images, thus complicating the analysis of cell features and behaviors.
Some notable applications in biology where low noise plays a vital role are:
- Genetic Studies: Noise reduction is vital in sequencing technologies, ensuring that the reads generated are precise.
- Neuroscience: In brain imaging, even minor noise can lead to significant errors in interpreting brain activity, thus emphasizing the importance of low noise setups.
By focusing on noise reduction in biological research, scientists can ensure they capture the most accurate, representative data possible, which is essential for breakthroughs in medical and pharmaceutical domains.
Physical Sciences and Low Noise Technology
The physical sciences also greatly benefit from low noise technologies. Experiments in fields like quantum mechanics, particle physics, and engineering frequently involve very delicate measurements. For instance, in quantum computing, maintaining low noise conditions is critical to prevent decoherence, which can disrupt quantum states.
Additionally, advanced experiments often employ technologies like cryogenic cooling and vacuum systems to achieve low noise environments. Such setups minimize thermal fluctuations and external interference, allowing scientists to obtain data that reflects more precise phenomena. Here are some areas in physical sciences where noise control matters:
- Spectroscopy: Accurate readings are crucial for understanding molecular structures. High noise levels could lead to incorrect spectra interpretation.
- Astrophysics: Low noise detectors are essential to observe faint celestial bodies. Unwanted signals can mask these observations, leading to potential misreadings.
Case Studies Demonstrating Low Noise Importance
The examination of low noise in scientific research has clear implications that are best understood through real-world applications. Case studies serve as an essential mechanism to illustrate the benefits of low noise environments in various fields. These studies provide concrete examples of how reducing noise can lead to significant advancements in technology, accuracy in data, and improved outcomes in research. They reinforce the theoretical concepts discussed previously, making a strong case for the necessity of low noise considerations in experimental design.
Biotechnology Innovations
In biotechnology, the need for low noise is critical due to the sensitive nature of biological systems. For instance, in the development of PCR (Polymerase Chain Reaction) techniques, researchers have demonstrated that low noise levels enhance the sensitivity of detection methods. A specific study showed that by utilizing low-noise amplification devices, researchers could detect even the slightest trace of genetic material—this has significant implications in fields like diagnostics and therapeutics.
The benefits of low noise in biotechnology extend beyond mere detection. In cell imaging, noise can obscure important details necessary for analysis. Technologies aimed at reducing background noise have lead to higher resolution images, enabling clearer observation of cellular processes. This advancement not only refines the accuracy of results but also accelerates the pace of innovation within the field—which is vital for ongoing research into diseases like cancer and genetic disorders.
Precision Measurement in Physics
Similarly, in the realm of physics, precision is paramount, especially in experimental settings that require highly accurate measurements. A notable example can be found in the field of quantum mechanics. Experiments involving quantum entanglement require extremely low noise levels to accurately measure quantum states. High noise levels can introduce significant errors, thus obscuring the results.
In one instance, a team of physicists working on a quantum sensor reported that by utilizing low noise technology, they achieved unprecedented accuracy in measuring gravitational waves. This was possible because the instruments could isolate more significant signals from random fluctuations, leading to discoveries that could enhance our understanding of the universe.
Through both biotechnology innovations and precision measurements in physics, it is evident that low noise is not merely a technical requirement—it is fundamental for achieving breakthroughs. Studies continually show that a focus on noise reduction policies results in clearer outcomes, improved methodologies, and ultimately, more reliable scientific knowledge.
Overall, examining such case studies highlights the significance of low noise environments. They are vital in ensuring data integrity, enhancing reproducibility, and facilitating advancements across disciplines. These findings contribute to the continuous dialogue about the role of low noise in scientific research, urging stakeholders to consider comprehensive noise management strategies.
Future Directions in Low Noise Research
The significance of advancing low noise research cannot be overstated. As scientific inquiry continues to evolve, the drive towards more precise measurements becomes critical. Low noise environments enable researchers to extract insightful data without interference. Future directions in this area promise to enhance our understanding across various domains, ensuring better reliability and innovation in research outcomes.
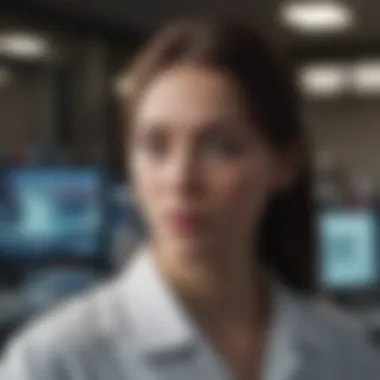
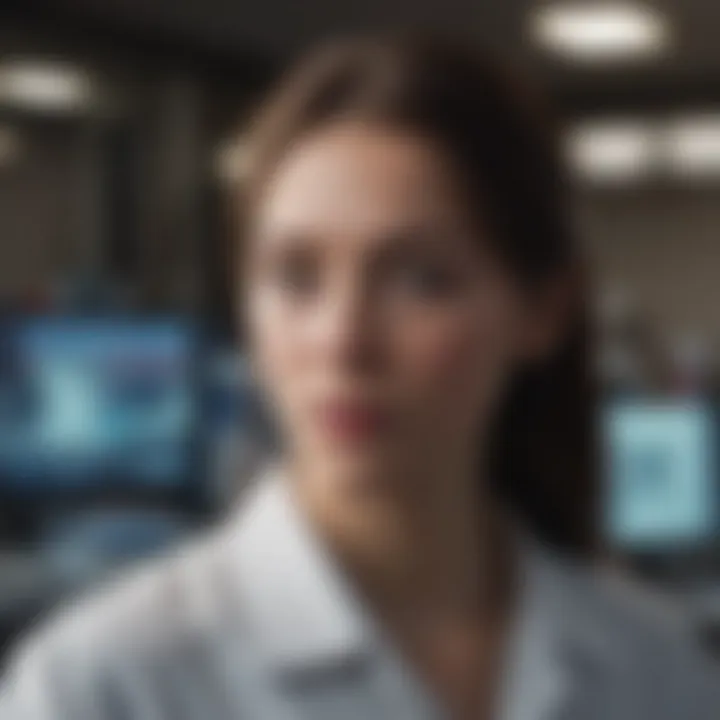
Emerging Technologies
Emerging technologies play a pivotal role in shaping the future of low noise research. Innovations in sensor technology, such as quantum sensors and advanced photonic devices, minimize noise levels significantly. For example, quantum sensors use principles of quantum mechanics to achieve measurements with unprecedented precision.
Another area is the development of electronic systems designed for low noise. These systems include components that have lower thermal noise, thus providing clearer signals. The integration of artificial intelligence in data processing also shows potential. Algorithms that intelligently filter out noise can drastically improve data quality. As these technologies develop, they pave the way for new scientific breakthroughs that were previously unattainable.
The future of low noise measurement can also benefit from developments in materials science. For instance, new materials like graphene have excellent electronic properties that can lead to lower noise in electronic devices. Research into superconductors promises advancements that could further decrease noise levels in laboratory environments.
Interdisciplinary Approaches
Interdisciplinary approaches in low noise research hold great promise. By collaborating across fields, scientists can create more holistic solutions to noise-related challenges. For example, partnerships between physicists, engineers, and biologists can enhance understanding of noise impacts on biological systems.
Collaboration also allows for sharing best practices and techniques developed in different domains. Lessons learned in electrical engineering on minimizing noise can inform practices in environmental science and vice versa. As research becomes increasingly cross-disciplinary, the synthesis of ideas will likely lead to innovative solutions.
Researchers can also collaborate with computer scientists to develop sophisticated noise filtering software. Such advancements would offer tremendous value in data analysis, ultimately contributing to higher fidelity results in experiments.
Emphasizing the importance of these approaches can enhance both the awareness and implementation of low noise strategies. In doing so, the scientific community will be better equipped to tackle complex research challenges.
"The convergence of various disciplines is likely to lead to unique insights and methodologies for reducing noise in scientific research."
"The convergence of various disciplines is likely to lead to unique insights and methodologies for reducing noise in scientific research."
Understanding future directions is crucial. As the landscape of scientific research changes, so too must our approach to managing noise. Knowledge exchange across fields, paired with emerging technologies, will enable more reliable experiments and contribute significantly to scientific progress.
Ethical Considerations in Noise Control
Scientific research operates under principles that extend beyond technical precision. Ethical considerations play a crucial role in addressing how noise affects data authenticity and research integrity. As the expectation for accountability in research intensifies, practices regarding how low noise environments are maintained and reported must also evolve.
Noise in experiments can influence conclusions drawn from data. Thus, researchers must navigate the ethical landscape surrounding noise management. This includes establishing protocols that not only minimize noise but also demand transparency in reporting methodologies and findings. The implications of ethical considerations in noise control can significantly shape scientific discourse, credibility, and trustworthiness.
Transparency in Reporting
Transparency is paramount in scientific research. When researchers disclose their methods and the noise levels encountered during experiments, it allows others to evaluate the reliability of the results. Lack of transparency can lead to skepticism about the findings and methodologies used, which can hinder scientific progress. Researchers should publish details about their noise reduction strategies. This practice supports reproducibility and fosters a culture of honesty in research.
Some key aspects of transparent reporting include:
- Documenting Noise Sources: Clearly present where noise originated. This can include environmental factors or instrumental contributions.
- Providing Context: Explain how noise influenced results. This gives a clearer perspective for peers reviewing the work.
- Encouraging Peer Review: Independent assessment can help identify potential oversights in noise management, as peers may catch problems the original researchers missed.
"Transparency not only enhances credibility but cultivates an environment of shared knowledge, allowing for more robust findings and advancements within the scientific community."
"Transparency not only enhances credibility but cultivates an environment of shared knowledge, allowing for more robust findings and advancements within the scientific community."
Ethical Implications of Manipulated Data
The manipulation of data in scientific research raises significant ethical concerns, particularly in the context of noise. With the pressure to produce significant results, some researchers may be tempted to adjust data sets to demonstrate low noise or favorable outcomes. Such actions not only jeopardize the integrity of the research but also the trust placed in the scientific community.
Researchers must remain vigilant against practices that may compromise ethical standards. Some implications of data manipulation include:
- Loss of Public Trust: If manipulated results surface, public perception of scientists and research may deteriorate.
- Scientific Misconduct: Altering data can lead to accusations of fraud, with potential professional and legal consequences.
- Erosion of Collaborative Efforts: Collaboration relies on trust. Manipulated data can harm partnerships, making effective teamwork difficult.
Ultimately, ethical considerations regarding noise control influence the quality and integrity of scientific research. By committing to transparency and ethical standards, the research community can advance knowledge responsibly, ensuring that findings contribute genuinely to their respective fields.
Educational Impact on Low Noise Awareness
Educational initiatives play a vital role in enhancing awareness of low noise in scientific research. Low noise is not just a technical specification; it embodies a foundational principle that underlies the integrity of scientific inquiry. By focusing on education and outreach in this area, researchers and students can better appreciate its significance and application in their work.
Importance in Curriculum Development
Integrating low noise concepts into academic curricula is crucial. Institutions can cultivate a robust understanding of noise management among future scientists. This integration should include theoretical principles of noise, practical applications, and advanced measurement technologies.
A curriculum that encompasses low noise principles can offer numerous benefits:
- Encourages critical thinking about experimental design.
- Informs students about real-world implications of noise in research findings.
- Prepares future researchers to implement effective noise reduction strategies.
Courses could include practical labs that simulate low noise environments. Such experiences will instill hands-on skills in managing noise, thus reinforcing students' understanding.
Training and Workshops for Researchers
Training and workshops for current researchers are equally important in building low noise awareness. These sessions can focus on innovative noise measurement techniques and the latest advancements in low noise technology. Researchers need continuous education to adapt to evolving scientific landscapes. The workshops can foster:
- Networking Opportunities: Connecting researchers from various fields could encourage collaboration on noise-related challenges.
- Hands-On Experience: Practical demonstrations can lead to a deeper understanding of noise control.
- Real-World Applications: Presenting case studies showcasing successful noise mitigation can inspire new research approaches.
These initiatives stress the growing importance of low noise environments, emphasizing its role in enhancing data integrity and reproducibility across disciplines.
These initiatives stress the growing importance of low noise environments, emphasizing its role in enhancing data integrity and reproducibility across disciplines.
Therefore, both curriculum development and professional training create a solid framework for understanding and tackling noise-related issues in research.
Noise Measurement Techniques
Noise measurement techniques hold significant importance in scientific research as they provide a framework to quantify the disturbances that can impact the integrity of experimental data. Understanding how to measure noise effectively allows researchers to establish baseline conditions, tweak experimental setups, and validate results. It serves to maintain a high standard in data acquisition and ensures that precise information can be drawn from observations, paving the way for reliable scientific conclusions.
Quantitative Assessment Tools
Quantitative assessment tools are invaluable in the measurement of noise levels in various research settings. These tools facilitate objective measurements, allowing scientists to quantify noise in numerical terms. Common instruments used include sound level meters and spectrum analyzers. They help gauge the intensity of noise, its frequency distribution, and other characteristics that define the noise profile in an experiment.
- Sound Level Meters: These devices measure sound in decibels (dB) and provide instant feedback on ambient noise levels. Accurate readings can guide decisions about laboratory design or necessary adjustments to experimental protocols.
- Spectrum Analyzers: These devices visualize the frequency components of noise. This is particularly useful for identifying specific noise sources that could undermine data integrity. For example, a frequency spike may indicate a malfunctioning piece of equipment.
- Data Acquisition Systems: Systems that collect data over time can be employed for long-term monitoring of noise fluctuations. When assessing instruments used in sensitive experiments, such monitoring can lead to valuable insights and help in adjusting methodologies.
Qualitative Analysis of Noise Sources
Qualitative analysis of noise sources offers a different perspective on noise measurement, focusing on identifying and categorizing the types of noise that impact research outcomes. This approach is crucial for a comprehensive understanding of noise in experiments as it can illuminate subtle influences that may not be captured in quantitative assessments.
To carry out qualitative analyses, researchers can:
- Conduct observational studies to note how environmental changes affect noise levels.
- Utilize interviews with laboratory personnel to gather perspectives on perceived noise sources and effects.
- Apply root cause analysis techniques to trace back to specific sources of noise, such as equipment, location, or operator actions.
Understanding the qualitative aspects of noise—rather than solely relying on numerical values—enables researchers to adopt a more holistic approach. This can lead to more informed alterations to experimental designs, ultimately aiming to enhance the overall quality of scientific inquiries and their results.
"Noise measurement is not just about numbers; it's about understanding the environment in which we are working."
"Noise measurement is not just about numbers; it's about understanding the environment in which we are working."
Collaborative Efforts to Address Noise in Research
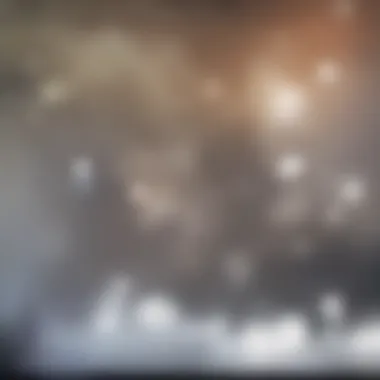
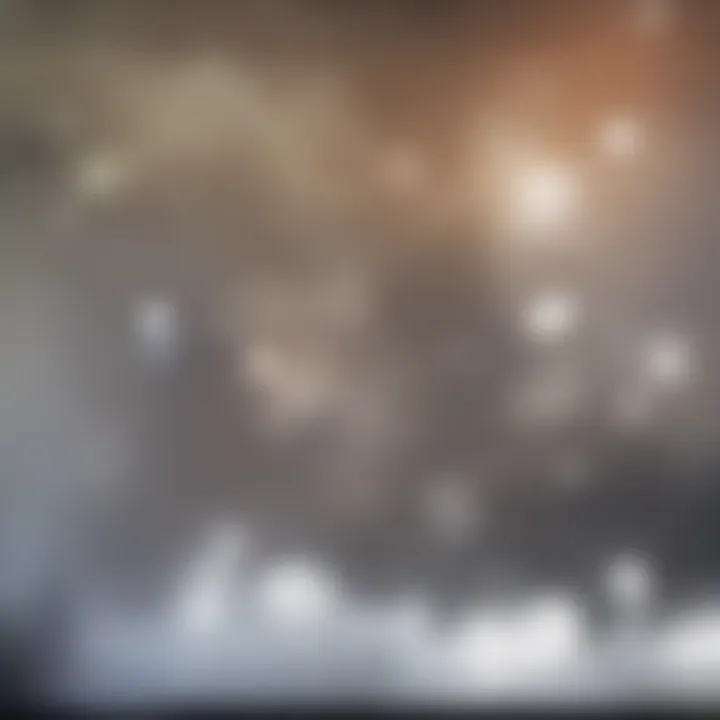
Collaborative efforts in research enhance the capability to tackle the challenge of low noise effectively. Noise reduction is not just a technical issue; it requires a shared vision across various fields. By integrating resources and knowledge from different research domains, scientists can develop better strategies for mitigating noise. Collaboration helps in pooling expertise and sharing best practices that can lead to innovative solutions. Ultimately, this cooperation could significantly improve the reliability of scientific discoveries, leading to better outcomes in various studies.
Industry and Academic Collaborations
Collaboration between industry and academia is crucial in addressing noise within scientific research. These partnerships facilitate the exchange of ideas, techniques, and resources. Companies that produce scientific equipment often have access to advanced technologies and insights that researchers can leverage. For instance, an academic researcher working on noise reduction can benefit from the latest sensor technologies being developed in the industry. In return, industry partners can gain access to cutting-edge research that may inform product development.
Some examples of notable partnerships include collaborations between universities and firms such as Siemens, which focus on precision measurement technologies. These efforts can lead to practical advancements that reduce noise in laboratory environments.
Collaborations can lead to solutions that may not have been possible in isolation.
Funding and Resources for Low Noise Initiatives
Securing funding is critical for noise reduction initiatives in research. Financial backing from both private and public sources can significantly enhance the capacity for noise management. Funding agencies are increasingly recognizing the importance of low noise environments in scientific research. Consequently, they are more likely to provide grants that focus on noise reduction technologies and methodologies.
In addition to government grants, private foundations and industry sponsorship can also boost financial support. Programs targeted at fostering innovation in noise reduction, such as National Science Foundation initiatives, may offer resources to researchers aiming to minimize noise.
Moreover, collaborations in funding can result in larger grants, allowing multidisciplinary teams to work together on comprehensive projects. This pooling of resources can facilitate the development of noise-resistant laboratory equipment or advanced data analysis software, ultimately leading to richer research outcomes.
Policy Framework Surrounding Noise Management
The policy framework surrounding noise management is crucial for ensuring that scientific research maintains integrity, precision, and reliability. Without a well-structured approach, noise can severely hinder the outcomes of experiments. This framework establishes guidelines and protocols which help researchers identify, measure, and mitigate noise effectively throughout their work.
A systematic policy framework enhances collaboration among various stakeholders. Researchers, institutions, and regulatory bodies must work together under a mutually agreed framework. This mutual understanding helps in establishing clear objectives for noise control in research environments.
Regulatory Guidelines
Regulatory guidelines provide a necessary structure for noise management. These guidelines ensure that all research conducted adheres to established standards. They encompass a variety of areas, including environmental considerations and specific requirements for laboratory settings. For instance, the regulations often recommend acceptable noise levels within laboratories and outline the steps to measure and report these levels.
Adherence to these guidelines is not just about legality; it is also about fostering a culture of responsibility within research communities. Researchers must have a clear understanding of their obligations, which helps to standardize practices across multiple disciplines. This standardization can lead to improved data quality and enhanced reproducibility of research findings.
Best Practices for Compliance
Implementing best practices for compliance is essential for researchers and institutions aiming to manage noise effectively. First, establishing a routine noise assessment protocol is critical. Regular monitoring can identify potential noise sources early, allowing for timely interventions.
Moreover, training personnel on the significance of noise management can foster a more conscientious approach to research practices. Providing workshops and creating educational resources can raise awareness among researchers about the impacts of noise on data integrity.
Additionally, investing in noise-reducing technology can also play a role in compliance. Upgrading laboratory equipment to reduce ambient noise and using sound-proof facilities will contribute significantly to maintaining a low-noise environment.
Ensuring compliance with these best practices will not only meet regulatory requirements but will also reinforce the reliability of research data, which is essential for advancing scientific knowledge.
"Noise control is not just an option; it is a necessity for quality research."
"Noise control is not just an option; it is a necessity for quality research."
By focusing on these policies and practices, the scientific community can continue to progress in creating a conducive environment for research that prioritizes accuracy and reliability.
Low Noise Technological Advancements
Innovations in Laboratory Equipment
Recent advancements in laboratory equipment emphasize the significance of maintaining low noise levels during scientific investigations. Equipment manufacturers have become increasingly aware of the need for precision, and as a result, they have developed various technologies specifically targeting noise reduction. For instance, electronic amplifiers optimized for minimal thermal noise allow for clearer data collection in sensitive experiments. Additionally, vibration-damping materials are now common in the design of setups to mitigate environmental interferences.
In the domain of microscopy, advances such as super-resolution imaging techniques have shown how low noise conditions can improve image clarity and resolution. This is essential in biological research, where image accuracy can influence interpretation of cellular processes. The use of advanced laser systems that minimize quantum noise presents a new direction for improving experimental outcomes as well.
Furthermore, reducing noise in devices such as microbalances and spectrophotometers ensures that measurements remain consistent and reliable. This directly impacts the reproducibility of experiments, a fundamental aspect of scientific research.
Software Solutions for Noise Analysis
Software solutions dedicated to noise analysis play a critical role in contemporary research. These programs help scientists understand the noise profiles of their data and identify potential sources of interference. Advanced data processing algorithms can filter out noise, thereby enhancing the signal being studied. For example, software like MATLAB or Python libraries can be employed to perform Fourier analysis, enabling researchers to discern patterns in data that might otherwise be masked by noise.
Moreover, machine learning algorithms can be integrated into noise analysis workflows. Such systems can efficiently categorize noise types and aid in predictive modeling, allowing researchers to anticipate and account for variations in their results. This proves especially beneficial for large-scale data sets, where manual data cleaning would be impractical and time-consuming.
Maintaining low noise levels enhances not only data integrity but also the overall efficiency of the research process. Thus, the investment in both hardware and software innovations is crucial for scientists aiming to produce high-quality research outputs.
The Role of Low Noise in Data Science
The role of low noise in data science is crucial. In an era dominated by big data and advanced analytical techniques, the clarity of data becomes paramount to ensure meaningful insights. Low noise levels enhance reliability, accuracy, and the overall integrity of data-driven results. By minimizing noise, researchers can focus on the true signals within the data, leading to better decision-making and predictive capabilities.
Data Cleaning and Preprocessing Techniques
Data often contains noise that can obscure meaningful patterns. Effective data cleaning and preprocessing techniques aim to address this issue. Here are some notable strategies:
- Outlier Detection: Identifying and removing anomalies in the data helps in improving the accuracy of statistical analyses.
- Smoothing Techniques: Techniques like moving averages or Gaussian filters can be employed to reduce random noise in time-series data.
- Normalization: Standardizing data ensures consistency across variables, thus reducing the impact of noise.
- Feature Selection: Choosing relevant features while eliminating irrelevant ones decreases the indirect noise from less informative data points.
These techniques are essential for ensuring that subsequent analyses reflect the underlying phenomena accurately.
Implications for Machine Learning Models
Low noise levels have significant implications in machine learning. Noise can hinder the performance of models, leading to overfitting or inaccurate predictions. Here are key considerations:
- Model Training: When models are trained on noisy datasets, they may learn from irrelevant features, which compromise their predictive power.
- Generalization: Models exposed to noise during training may fail to generalize to unseen data, undermining their practical application.
- Validation: Low noise allows for more reliable model evaluation because it minimizes random variation that can mislead performance metrics.
- Feature Engineering: Sound features crafted from low noise data can enhance model robustness and efficacy.
"Noise is the enemy of predictive analysis. Reducing it enhances model performance and makes outcomes more reliable."
"Noise is the enemy of predictive analysis. Reducing it enhances model performance and makes outcomes more reliable."
The absence of noise in data science leads to better predictive models and more accurate conclusions. As research continues to evolve, understanding the influence of noise remains essential in developing robust approaches for analysis and machine learning.
Concluding Thoughts on Low Noise Research
Low noise in scientific research is a critical consideration that affects the validity of experimental findings. As this article has shown, noise can come from numerous sources and can significantly impact data integrity. Understanding the importance of maintaining low noise levels can benefit various research disciplines. It allows for more accurate data and strengthens the reproducibility of scientific results. Low noise environments support high-precision measurements, ensuring that the findings reflect genuine phenomena rather than artifacts of the experimental setup.
Summation of Key Findings
The investigation into low noise has led to several key findings:
- Definition and Importance: Low noise refers to minimal unwanted variations in data that could distort the results of an experiment. This article has highlighted that maintaining low noise is fundamental for precision in measurements and reproducibility of results across disciplines.
- Sources of Noise: Various sources, including environmental factors and instrumental contributions, contribute to noise in experiments. Recognizing these sources is crucial for implementing effective noise reduction strategies.
- Impact on Data Integrity: The presence of noise can yield inaccuracies in data, potentially leading to flawed conclusions. This article illustrated how noise can challenge the reliability of the scientific process.
- Strategies for Control: Effective experimental design considerations and noise reduction techniques are essential. By implementing systematic approaches, researchers can minimize noise and enhance the reliability of their findings.
"Reducing noise is not merely about improving data clarity; it is about ensuring that the scientific inquiry remains robust and credible."
"Reducing noise is not merely about improving data clarity; it is about ensuring that the scientific inquiry remains robust and credible."
Recommendations for Future Research
The delicate nature of noise involves ongoing research and development. Future investigations should consider the following aspects:
- Emerging Technologies: As technology advances, new methods for noise reduction are likely to emerge. Research should focus on the application of these technologies across various fields.
- Collaborative Interdisciplinary Projects: Collaborations between physicists, biologists, and engineers can lead to innovative solutions in noise management.
- Training and Education: Increasing awareness of noise control methods in academic curricula will prepare the next generation of researchers to tackle these challenges effectively.
- Standardization of Measurement Techniques: Establishing standardized protocols for measuring and reporting noise will enhance the comparability of research findings across different studies.
- Longitudinal Studies on Noise Impacts: Further research should be conducted to understand how long-term exposure to different noise levels affects experimental outcomes.
The trajectory of low noise research will significantly influence scientific inquiry, ensuring that future discoveries are based on sound and reliable data.