Exploring Scientific Data Analysis Techniques and Insights
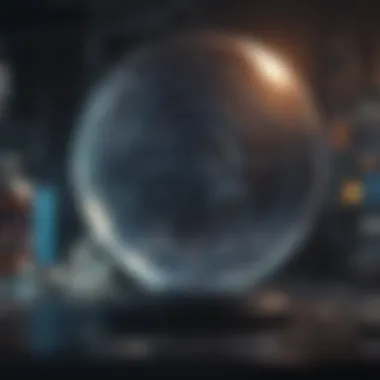
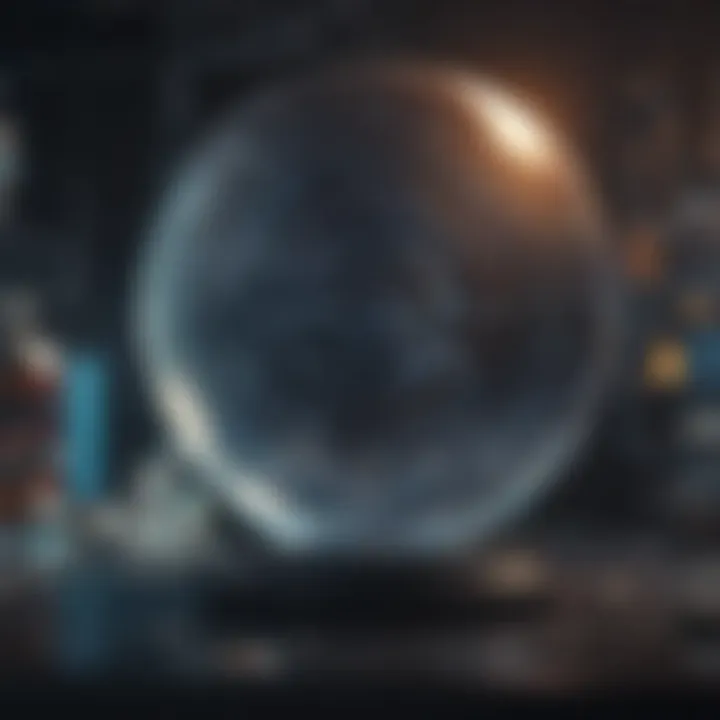
Article Overview
Purpose of the Article
In the ever-expanding universe of scientific research, the analysis of data stands at the core of discovering insights that can propel us toward groundbreaking advancements. This article aims to demystify scientific data analysis, illustrating its methodologies and applications across a wide array of scientific fields. By examining the nuances of various data collection techniques, analytical strategies, and the transformative role of technology, the goal here is not only to inform but to highlight how raw data can be turned into actionable insights.
Relevance to Multiple Disciplines
Scientific data analysis is not a discipline in isolation. It finds its fingers in numerous pies — from life sciences to social sciences. Nearly every scientific field relies on data to back up its findings, be it through experimental research in biology or statistical modeling in economics. Consequently, comprehending the landscape of data analysis is crucial for students, educators, researchers, and professionals alike. With processes and tools constantly evolving, staying abreast of these changes can provide a competitive edge in any scientific endeavor.
Research Background
Historical Context
The journey of data analysis has roots that extend back to the dawn of scientific inquiry. In the early days, researchers relied on rudimentary counting and record-keeping methods. Fast-forward to the 20th century, and we see the emergence of more sophisticated statistical methods, shaped by pioneers like Ronald A. Fisher and Karl Pearson. Their work laid the groundwork for modern data analysis, introducing concepts such as probability theory and inferential statistics, which are crucial for conducting advanced data analyses today.
Key Concepts and Definitions
At the heart of scientific data analysis are several key concepts that form the backbone of its methodologies:
- Data Collection: This involves gathering information from various sources, which could be experimental results, surveys, or observations. The methods used can deeply influence the quality of the data collected.
- Statistical Analysis: After collecting the data, researchers apply tools and techniques to interpret the findings. Methods range from basic descriptive statistics to complex inferential techniques.
- Data Visualization: Presenting data visually can make the findings more comprehensible. Techniques like graphs, charts, and 3D models are often used to convey results effectively.
- Big Data: A term that encapsulates the challenges posed by massive datasets. Techniques to manage, analyze, and draw inferences from big data are crucial, as traditional methods often fall short.
“Data is the new oil.” -- A phrase often used to highlight the value of data in driving insights and innovations.
“Data is the new oil.” -- A phrase often used to highlight the value of data in driving insights and innovations.
By grasping these concepts, individuals can better navigate the sometimes convoluted pathways of scientific data analysis, unlocking new avenues for exploration and understanding.
Understanding Scientific Data Analysis
Understanding scientific data analysis is crucial for any individual involved in research or any data-driven projects. It serves as the backbone for making informed decisions and deriving insights that can lead to significant advancements in various fields. The ability to transform raw data into meaningful conclusions not only enhances research quality but also fosters improved understanding across disciplines. This aspect of research, while often overlooked, deserves careful examination, as the implications can affect the validity of entire studies and experiments.
The landscape of scientific data analysis isn’t just about crunching numbers. It demands a holistic approach that incorporates proper methodologies, ethical considerations, and a grasp of technological tools. Consider this: while the data collected might be rich with potential, it requires a structured analysis pathway to reveal its true value. Thus, understanding the nuances of data analysis ensures researchers not only collect data effectively but also interpret and apply their findings in a way that adds to the body of knowledge.
Defining Data Analysis in a Scientific Context
At its core, data analysis in a scientific context involves systematically applying statistical and logical techniques to describe, condense, and evaluate data. This encompasses everything from simple descriptive statistics that summarize data sets, to complex inferential statistics that help in making predictions based on the data. The primary goal here is clarity. Researchers and scientists must grasp their data to unravel patterns, test hypotheses, and draw valid conclusions.
For instance, in a study examining the effectiveness of a new cancer treatment, data analysis allows researchers to determine whether observed improvements can be attributed to the treatment or are just random fluctuations. Without robust analysis, the integrity of their findings could be compromised. Therefore, employing sound data analysis techniques is not only a best practice but a necessity for valid scientific discovery.
The Evolution of Data Analysis Techniques
Data analysis hasn't stayed stagnant; it has evolved immensely over the decades, adapting to technological advancements and the ever-growing complexity of datasets. Early approaches were often rudimentary, relying heavily on manual calculations and simple statistical methods. However, with the advent of computers, this landscape shifted dramatically.
Today, analysts have access to an arsenal of sophisticated software and tools that can unravel complex datasets that were previously unmanageable. Techniques such as regression analysis, factor analysis, and cluster analysis enable researchers to glean insights from intricate data structures. Moreover, the rise of machine learning algorithms has taken analysis to new heights, allowing for predictive modeling that can anticipate trends and inform decision-making.
As we advance, understanding these evolving methods is essential for researchers who wish to remain at the forefront of scientific analysis. Being well-versed in both traditional and modern techniques empowers them to choose the most suitable approach for their specific research questions.
As we advance, understanding these evolving methods is essential for researchers who wish to remain at the forefront of scientific analysis. Being well-versed in both traditional and modern techniques empowers them to choose the most suitable approach for their specific research questions.
The Data Lifecycle in Scientific Research
The journey of scientific inquiry is intricately tied to what is known as the data lifecycle. This refers to the various stages that scientific data undergoes, starting from its initial collection and extending through to its application in analysis and conclusions. Understanding this lifecycle is crucial, as it highlights the importance of managing and interpreting data correctly, particularly in a world where the volume of data is growing at an astonishing rate.
Data Collection Practices
Data collection is the cornerstone of any scientific study. It holds the key to ensuring that the information gathered is accurate, reliable, and valid. Different fields rely on various methods, depending on the phenomena they are studying.
- Quantitative Methods: Researchers often employ structured data collection methods, such as surveys or experiments, where specific metrics can be easily measured and analyzed.
- Qualitative Methods: In contrast, qualitative methods involve less structured approaches, what's often seen in interviews or focus groups. This allows for deeper insights into the subject matter, although it typically requires more rigorous interpretation later.
Proper planning and execution in this stage offer several benefits:
- Ensures data integrity, paving the way for valid analysis.
- Maximizes the potential for insightful conclusions, which can lead to significant advancements in your field of study.
- A well-structured approach reduces the chances of collecting irrelevant or erroneous data, thus avoiding complications down the line.
Data Interpretation and Inference
Once data has been collected, the next critical stage is data interpretation and inference. It’s not just about what the data says; it’s about what it means.
Data interpretation involves transforming the raw data into understandable formats. For instance, researchers might create visual representations, such as graphs or charts, to communicate findings effectively. Through these visual aids, patterns and trends emerge. This stage requires not only technical skills but also critical thinking and contextual understanding.
Moreover, the step of inference is essential in establishing broader conclusions based on the data collected. From this, researchers can formulate hypotheses and make predictions about future outcomes. It involves:
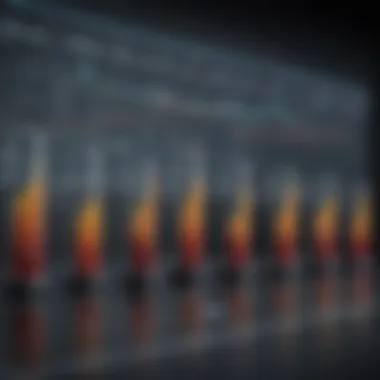
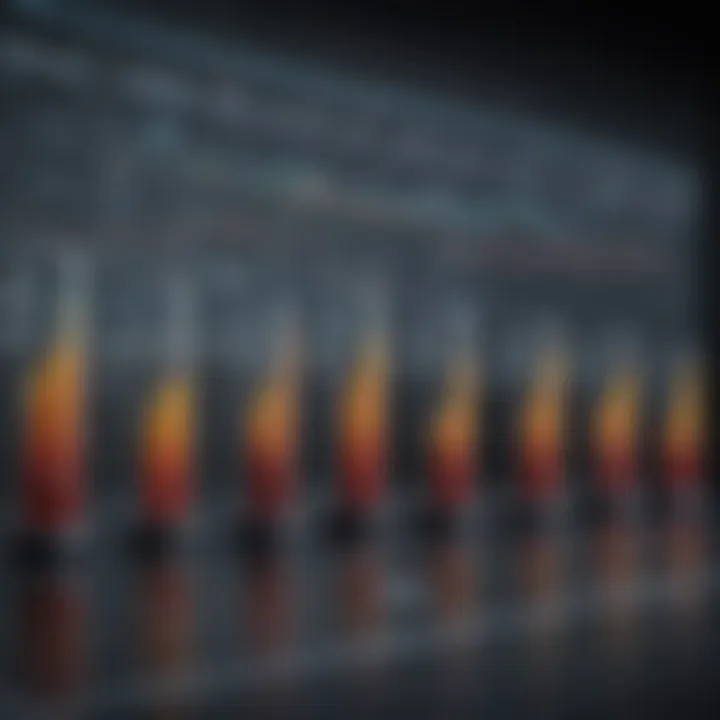
- Drawing meaningful connections between variables observed in the data.
- Making decisions based on statistical analyses, often employing inferential statistics to evaluate the likelihood that a given result could occur by chance.
Importantly, during interpretation, one must remain vigilant against biases and inaccuracies that could cloud judgment. Relying solely on data without considering external variables or potential confounders can lead to misguided conclusions.
"Data has no meaning without context; understanding is everything."
"Data has no meaning without context; understanding is everything."
In essence, navigating the data lifecycle is not a mere checklist; it reflects careful orchestration of various elements. Recognizing the stages of data collection and interpretation fortifies the foundation for robust scientific inquiry. While the landscape of data is complex, mastering it paves the way for impactful discoveries.
Key Methodologies in Data Analysis
Understanding the methodologies used in scientific data analysis is akin to having a map in uncharted territory. As the field evolves, adopting the right set of techniques becomes essential for researchers to draw meaningful insights from vast amounts of data. These methodologies not only determine how data is summarized and interpreted, but they also impact the decisions made based on this analysis. To navigate the intricate landscape of scientific inquiry, some key methodologies warrant particular attention: descriptive statistics, inferential statistics, and machine learning techniques. Each of these plays a vital role in ensuring that data analysis serves its purpose effectively and accurately.
Descriptive Statistics: Summarizing Data
Descriptive statistics are crucial for providing a snapshot of the data at hand. Imagine sifting through a mountain of data; descriptive stats help clear away the noise, allowing researchers to see the big picture right away. These techniques involve calculating various measures that summarize the central tendency, variability, and overall distribution of data sets. Common metrics include mean, median, mode, range, and standard deviation. Through their use, researchers can easily identify patterns that may provoke further investigation.
Moreover, visual aids like histograms, bar charts, or box plots enhance comprehension significantly. They serve not just as informative tools but also as a way to communicate findings effectively. The user-friendly nature of descriptive statistics makes them indispensable, particularly for those who may not be statistically inclined.
"Descriptive statistics serve as the first step in data analysis, paving the way for deeper exploration and understanding."
"Descriptive statistics serve as the first step in data analysis, paving the way for deeper exploration and understanding."
Benefits of Descriptive Statistics:
- Easy to interpret results
- Quick calculations that provide immediate insights
- Acts as a foundation for more sophisticated analysis
Inferential Statistics: Drawing Finales
While descriptive statistics provide a solid foundation, it is inferential statistics that allow researchers to extend their findings beyond the sample to a larger population. This branch of statistics plays a pivotal role in hypothesis testing, prediction, and determining relationships among variables. For instance, when a researcher conducts a t-test or ANOVA, they infer about a larger group of data based on a sample, helping answer questions like "Do these two groups differ significantly?"
The significance of confidence intervals and p-values cannot be understated. They help gauge the reliability of results, informing researchers whether their observations stem from a true effect or mere chance. In a world increasingly driven by data, the ability to draw sound conclusions based on analysis is critical not just for publication but also for real-life applications, such as public policy or clinical practices.
Challenges Typically Found in Inferential Statistics:
- Misinterpretation of p-values leading to incorrect assumptions
- Over-reliance on small sample sizes affecting validity
- Complications arising from data outliers and variability
Machine Learning Techniques
Machine learning has revolutionized data analysis, infusing traditional methods with advanced computational capabilities. These techniques allow for pattern recognition and predictive analysis through algorithms that learn from data. One could think of machine learning as a smart assistant that hones its skills over time, improving the quality and precision of its insights. Common methods like regression analysis, decision trees, and neural networks serve to automate processes that, in the past, demanded meticulous human intervention.
The versatility of machine learning shows itself across various domains—from predicting health outcomes in medical research to recognizing trends in economic data. This methodology democratizes data analysis, enabling even those without specific statistical training to derive significant insights from the data they possess.
Considerations with Machine Learning Techniques:
- Requires a significant amount of clean data to function effectively
- Overfitting can lead to misleading models
- Interpretability of complex models can be challenging
In sum, the effective application of these methodologies not only equips researchers with the tools needed for robust data analysis but also underlines the importance of a meticulous approach. Distilling complex data into actionable insights enriches the scientific discourse, paving the way for innovations that can influence every corner of our understanding.
The Role of Technology in Data Analysis
In the modern scientific landscape, technology serves as the backbone of data analysis, revolutionizing the way we interpret and understand vast quantities of information. The shift from manual methods to technology-driven approaches has not only expanded the tools available but also increased the efficiency and effectiveness of scientific inquiry.
Technological advancements have paved the way for various methodologies, enabling researchers and practitioners to tackle complex problems with greater precision. The integration of software, data visualization tools, and advanced computational methods has made data analysis accessible and manageable, even for intricate datasets. This technological synergy is essential for drawing accurate conclusions from data, which can ultimately influence various scientific fields ranging from biology to climate science.
Understanding the pivotal role that technology plays in data analysis is not just essential for current researchers, but also for students and educators who aim to cultivate a data-driven mindset in future generations.
Data Analysis Software and Tools
Data analysis software and tools are fundamental elements that significantly enhance data-handling capabilities. From statistical packages to specialized programming languages, the options available enable analysts to sift through extensive datasets efficiently. Programs like R and Python have gained immense popularity due to their versatility and extensive libraries, which cater to diverse analytical needs.
Some key software used in scientific data analysis includes:
- R: Highly powerful for statistical analysis and visualization.
- Python: Its libraries like Pandas and NumPy facilitate data manipulation and analysis.
- MATLAB: Often used in academia for numerical computations and algorithm development.
Additionally, there are specific tools designed for certain applications, such as Tableau for data visualization or SAS for advanced analytics. Each tool has its strengths and weaknesses, and the choice often hinges on the type of data being analyzed and the specific requirements of the research.
Leveraging these tools allows scientists to automate processes that would otherwise be time-consuming. With built-in functions and user-friendly interfaces, researchers can focus on the interpretation of results rather than the arduous task of data handling.
The Impact of Cloud Computing
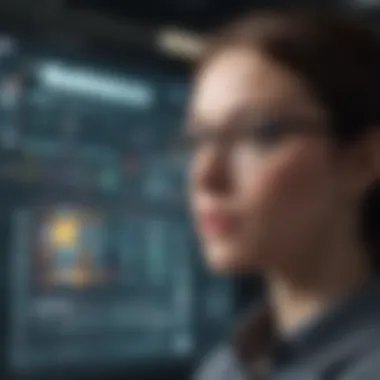
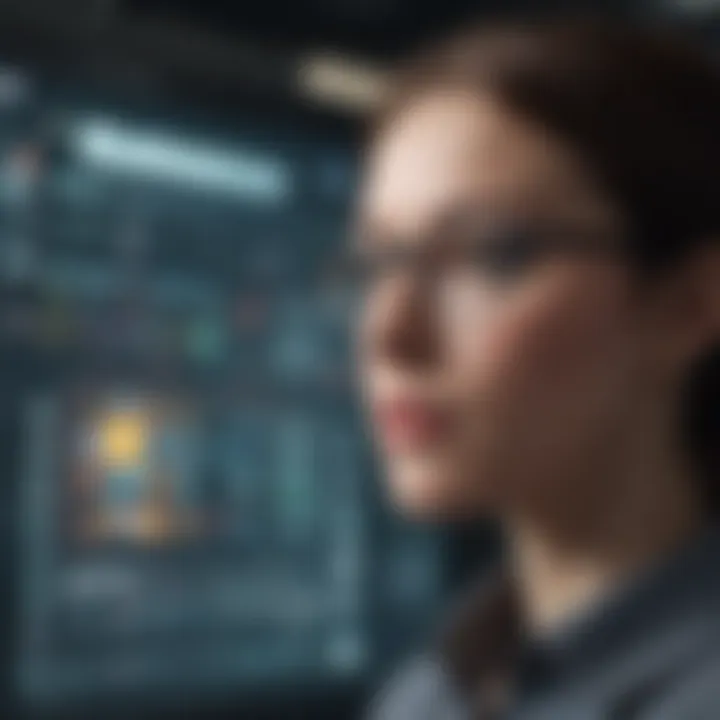
Cloud computing is another significant player in the data analysis arena, transforming how data is stored, processed, and accessed. The scalability offered by cloud services allows researchers to manage large datasets without the constraints of local storage capacity.
The impact of cloud computing can be summarized in several vital aspects:
- Accessibility: Data stored in the cloud can be accessed from anywhere, which enables collaboration among researchers globally. Remote work has become a standard practice, facilitating diverse teams to communicate and analyze data effectively.
- Cost-Effectiveness: Rather than investing heavily in physical infrastructure, scientists and organizations can utilize pay-as-you-go pricing models offered by various cloud services. This flexibility can be crucial for funding-limited projects.
- Enhanced Data Security: While concerns exist regarding data privacy, reputable cloud providers invest significantly in robust security measures, helping to protect sensitive data from breaches.
- Real-Time Analysis: The ability to analyze data in real-time allows for quick decision-making. For instance, researchers can monitor experiments live and adjust parameters as needed, optimizing results.
Overall, the integration of cloud computing into scientific data analysis represents a pivotal evolution that addresses challenges inherent in traditional data processing methods. Researchers who embrace these technologies are not just keeping up with the times; they are significantly enhancing their analytical capabilities, opening doors to new discoveries and insights.
"In the realm of scientific research, those who harness the power of technology hold the keys to unlocking untold answers to age-old questions."
"In the realm of scientific research, those who harness the power of technology hold the keys to unlocking untold answers to age-old questions."
Through the utilization of varied software and the advantages offered by cloud computing, data analysis becomes a more dynamic practice, thereby advancing science as a whole.
Challenges in Scientific Data Analysis
Scientific data analysis is not a straight path; it has its own set of bumps and potholes. These challenges can cause significant hurdles for researchers trying to derive meaningful insights from data. Understanding these issues is essential, as they not only affect the accuracy and completeness of research findings but also influence our broader understanding of scientific inquiry itself.
The importance of addressing these challenges lies in their potential to undermine the value of conclusions drawn from data. When researchers face issues like data quality, it can lead to misleading interpretations. Similarly, the complexities surrounding big data add layers of difficulty, making analysis cumbersome and potentially erroneous. Researchers need to be aware of these obstacles to ensure they navigate the landscape effectively.
Data Quality and Integrity Issues
Data quality is fundamental in scientific research. Poor data quality can skew results and lead to incorrect conclusions. Several factors contribute to data integrity issues:
- Data Acquisition: The methods and instruments used to collect data can introduce errors. If the equipment isn't calibrated correctly or if data is collected from unreliable sources, the viability of the data comes into question.
- Data Processing: Even after data is collected, processing errors can occur. Whether it’s data entry mistakes or software bugs, inaccuracies can creep in unnoticed.
- Data Loss: With large datasets, it’s common for users to face data loss issues. Not having backups or failing to secure data can jeopardize entire studies.
These challenges can manifest in various ways. Researchers might find themselves spending valuable time trying to rectify issues rather than advancing their analyses. Collaboration among scientists and statisticians can help here, as discussions on methodologies can shed light on persistent flaws in data collection and processing. Keeping an eye on data integrity is crucial for ensuring the reliability of scientific conclusions.
Big Data Challenges
The term "big data" refers to data sets that are so large and complex that traditional data processing applications are inadequate. In the realm of scientific data analysis, big data brings both opportunities and challenges. Among the challenges are:
- Storage: Storing immense datasets requires massive infrastructure, which may not be accessible to all researchers. Additionally, these storage solutions must be secure and efficient.
- Computational Power: Analyzing vast amounts of data demands powerful computing resources that can be prohibitively expensive. Handling this analytics complexity can drain time and resources.
- Data Heterogeneity: Big data often comes from various sources and formats, presenting difficulties in integration. Variations in data types can complicate analyses, forcing researchers to spend more time on data cleaning than on drawing insights.
Research teams have to collaborate not just within their field but also with experts in computer science and data management. Adopting newer algorithms designed for big data can help, but it requires a shift in perspective for many researchers.
Ethical Considerations in Data Analysis
In today's data-driven world, navigating the ethical considerations in data analysis is paramount. Researchers, educators, and professionals face the challenge of not only collecting and interpreting data but also ensuring that their methods align with ethical standards that protect individuals and promote scientific integrity. The importance of ethics in data analysis cannot be understated, as it encompasses several elements that influence public trust, uphold societal norms, and advance the credibility of scientific research.
One significant aspect is data privacy and security. With the increasing prevalence of big data, vast amounts of personal information are collected, often without participants' full awareness. This raised alarms regarding how data is stored, accessed, and shared. Researchers must ensure that data handling practices comply with legal standards like the General Data Protection Regulation (GDPR). Failure to do so can lead to severe repercussions, including legal penalties and loss of public trust. Researchers often benefit from integrating cutting-edge encryption methods, anonymization techniques, and clear data usage policies, fostering a sense of security and trust among participants.
"Data without ethics is like a ship without sails – it will drift into dangerous waters."
"Data without ethics is like a ship without sails – it will drift into dangerous waters."
Furthermore, ethical data analysis hinges on bias and fairness in data interpretation. Different interpretations can influence conclusions drawn from data and may affect major decisions impacting lives, environments, and economies. A notorious example can be found in predictive policing algorithms, which, if inherently biased, could lead to systemic discrimination against certain demographics.
To combat bias, it is crucial to:
- Engage diverse teams in the data analysis process to gather multiple perspectives.
- Regularly audit data sources for potential biases before analysis.
- Use inclusive methodologies that ensure fairness across various groups.
By implementing these strategies, researchers can work towards delivering more accurate and equitable interpretations of their findings. Additionally, emphasizing the role of ethics can enhance the validity of the analysis and thereby bolster the overall impact of scientific work on society.
Applications of Scientific Data Analysis
Scientific data analysis is not just a theoretical exercise; it serves as a cornerstone for practical applications across a broad array of disciplines. The importance of applying data analysis in scientific research cannot be overstated. This process helps in drawing meaningful insights from raw data, which can lead to significant discoveries, inform policy decisions, and foster innovation. Understanding how to effectively analyze data allows researchers to interpret complex phenomena and share findings with the wider community.
Benefits of Applications
- Enhanced Decision-Making: By sifting through data, scientists can make informed predictions and decisions. This accuracy is fundamental when trying to address real-world problems. For instance, epidemiologists analyze health data to track disease outbreaks, guiding public health responses.
- Optimization of Resources: In fields like agriculture, data analysis helps optimize planting schedules and resource use. Farmers utilize data-driven models to predict weather patterns and improve yield without exhausting their land’s resources.
- Interdisciplinary Collaboration: Scientific data analysis fosters collaboration among different fields. For example, biochemists might partner with computer scientists to analyze protein structures, bridging the gap between disciplines and inviting innovative solutions.
- Understanding Complex Systems: Many scientific inquiries involve complex systems — ecosystems, human bodies, or even social structures. Data analysis unveils relationships and interactions within these systems, which might otherwise remain hidden.
Considerations
While applications of scientific data analysis offer numerous benefits, some considerations mustn’t be overlooked:
- Data Quality: The effectiveness of data analysis largely hinges on the quality of input data. Ensuring accuracy and reliability is crucial to prevent misleading results.
- Interpretation Complexity: Even the most thorough analysis may lead to misinterpretation. Researchers must be careful when drawing conclusions, ensuring results align with established knowledge.
- Ethical Implications: As we’ve noted in earlier sections, ethical considerations remain paramount. Data privacy, consent, and bias in data collection and interpretation can skew analyses and must be addressed rigorously.
"Data is like garbage. You’d better know what you are going to do with it before you collect it." – Mark Twain
"Data is like garbage. You’d better know what you are going to do with it before you collect it." – Mark Twain
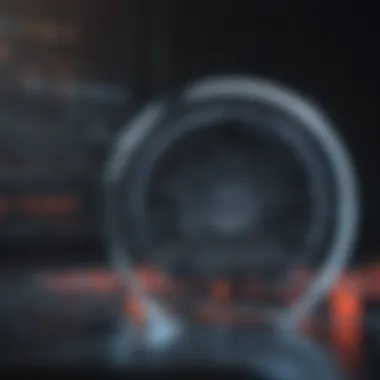
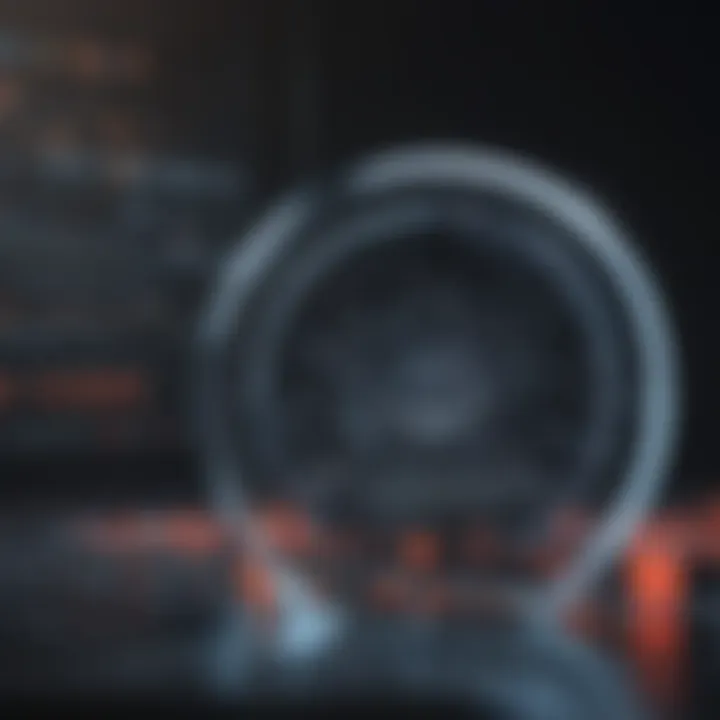
In sum, the realm of scientific data analysis is rich with applications that can significantly impact research and society. Understanding these applications allows researchers and professionals to harness the power of data effectively.
Case Studies in Biology
Biology is a field ripe with data — from genomics to ecology. By diving into specific case studies, we gain insights into how data analysis can lead to remarkable discoveries. One prominent example is the Human Genome Project. This monumental effort utilized complex data analysis to map the entire human genome, helping identify genetic markers for diseases. The advancements in bioinformatics tools enabled analysis at unprecedented speeds and accuracy.
Researchers now have access to vast data sets from different biological studies, leading to breakthroughs in understanding genetic disorders and identifying potential therapeutic targets.
Case Studies in Chemistry
In chemistry, data analysis plays a vital role in everything from drug discovery to environmental monitoring. One exemplary case study is the use of cheminformatics – where data analysis integrates chemical information. A practical illustration is how predictive models were developed to identify promising compounds for new medication. Here, machine learning techniques sift through myriad chemical structures, predicting their efficacy based on historical data. This boosts efficiency significantly in the drug development process, allowing researchers to focus on the most viable candidates.
Case Studies in Earth Sciences
Earth sciences heavily rely on data analysis to comprehend climate change, geological processes, and natural disaster predictions. Take, for instance, the use of satellite data to track deforestation rates. Using time series analysis, researchers can quantify changes in forest cover over decades, providing crucial data for conservation efforts. Similarly, seismic data analysis plays an invaluable role in earthquake prediction and risk assessment, helping communities prepare for potential disasters.
The various applications of data analysis across biology, chemistry, and earth sciences demonstrate its critical role in advancing scientific knowledge and addressing challenges facing our world today.
Future Trends in Data Analysis
The realm of scientific data analysis is continuously morphing, driven by technological advancements and the growing complexities of data itself. In the current narrative of research, understanding future trends is not merely beneficial—it’s imperative. Proficiently navigating these trends ensures that data analysts and researchers remain at the forefront, capable of tackling emerging challenges and harnessing novel opportunities.
Three significant elements emerge from this discussion: the rise of artificial intelligence, the push towards interdisciplinary approaches, and the necessity of adaptable methodologies. Each of these facets interlocks, creating a tapestry of interconnected strategies that aim to amplify the effectiveness and efficiency of data analysis in various scientific fields.
The Rise of Artificial Intelligence
Artificial intelligence (AI) has undoubtedly emerged as a powerhouse in data analysis. It transcends the capabilities of traditional methods by leveraging algorithms that can learn from and make decisions based on data. AI's contributions can be seen across disciplines, reshaping how researchers approach data interpretation and model building.
In practical terms, here’s what makes AI so influential:
- Automation of repetitive tasks: AI can handle large volumes of data processing, freeing researchers to focus on more complex analytical tasks. For instance, in genomics, AI algorithms sift through genetic sequences at unprecedented speeds, revealing patterns unseen by the naked eye.
- Predictive analytics: AI systems can generate predictive models that aid in anticipating future outcomes based on historical data. This element proves invaluable in fields like climate science, where understanding long-term trends is vital.
- Enhanced accuracy: The integration of machine learning techniques contributes to more accurate predictions and classifications, which is crucial when making scientific determinations.
"AI is reshaping how scientists approach research, making data exploration less about sifting through numbers and more about extracting meaningful insights."
"AI is reshaping how scientists approach research, making data exploration less about sifting through numbers and more about extracting meaningful insights."
As researchers begin to embrace these tools, the question arises: how do we integrate AI responsibly? The ethical landscape grows more intricate. Decisions made by AI models must be transparent and justified to avoid biases that can skew scientific conclusions.
Interdisciplinary Approaches to Data Analysis
The complexities of real-world problems call for cross-disciplinary collaboration. Data analysis is reaching across borders, blending insights from various fields, creating something entirely new. This approach fosters a kind of synergy that can generate innovative solutions to challenging questions.
Here are several points illustrating the benefits of interdisciplinary collaboration in data analysis:
- Broader Perspectives: A team composed of experts from biology, computer science, and social sciences can approach a problem from differing angles, allowing multifaceted insights.
- Resource Sharing: Different disciplines often have access to varied datasets or analytical tools. By pooling these resources, researchers can overcome data limitations that one discipline alone might face.
- Novel Methodologies: Creating new analytical frameworks can emerge from combining methodologies of distinct fields. For example, integrating social science models with computational analytics could yield fresh perspectives in behavioral research.
However, blending distinct disciplines is not without its challenges. Effective communication becomes paramount, as jargon and methodologies may clash. Building a common ground is crucial for any collaborative endeavor, ensuring teams leverage their strengths rather than allowing misunderstandings to hamper progress.
In summary, anticipating future trends in scientific data analysis opens the door to revolutionary advancements. With AI reshaping analytical landscapes and interdisciplinary collaboration enabling innovative solutions, researchers can look forward to a more interconnected and insightful approach to analysis, one that not only keeps pace with the complexities of data but enhances the scientific inquiry itself.
Ultimately, embracing these trends will not only improve analytical rigor but also foster a culture of innovation that catapults scientific understanding into new territories.
Culmination and Implications for Research
In wrapping up the discussion on scientific data analysis, it becomes clear that a thoughtful approach to data can lead to groundbreaking discoveries. The implications of effective data analysis stretch far and wide, influencing not only the outcomes of research but also the methodologies employed across various disciplines. One of the most significant insights is the necessity of integrating data analysis deeply into the research process.
With the rapid evolution of technology and methodologies, researchers must adapt their practices to stay at the cutting edge. This demands a keen understanding of both the benefits and challenges that come with analyzing scientific data.
- Reinforcement of Evidence-Based Decision Making: The insights derived from data enable researchers to ground their findings in evidence rather than intuition. This moves the culture of research towards a more rigorous and scientifically substantiated framework.
- Encouraging Reproducibility: With standardized data analysis processes, replicating research studies becomes significantly easier. Enhanced reproducibility strengthens trust in scientific findings and fosters a collaborative spirit within the research community.
- Informed Policy and Practice: Decision-makers benefit immensely from the insights provided by robust data analysis. By applying these insights, policies can be shaped to tackle the very real challenges facing contemporary society, particularly in health, environmental, and technological realms.
- Promoting Interdisciplinary Collaboration: As research fields converge, data analysis can serve as a common language that breaks down silos, enabling different disciplines to work together towards shared goals. Such collaboration often leads to innovative approaches and solutions.
"The most profound discoveries often lie hidden within the data, waiting to be brought to light."
"The most profound discoveries often lie hidden within the data, waiting to be brought to light."
While it’s essential to remain vigilant about the ethical considerations surrounding data use and analysis, the benefits of ensuring a well-rounded research culture that embraces data are numerous. Researchers, educators, and students alike must consider the implications of their data analysis to inspire progressive research methods that yield impactful results.
Summarizing Key Insights
To draw everything together, it is critical to remember several pivotal points from our exploration:
- The Centrality of Data: Data serves as the backbone of informed research across disciplines. Whether it be in environmental studies, health sciences or technology, the method of analyzing data plays a crucial role in shaping outcomes.
- Evolving Methodologies Require Adaptability: With the introduction of machine learning and advanced analytics tools, researchers must remain flexible and open to integrating novel methodologies into their work.
- Ethics and Rigor Matter: We live in an age where misuse of data can lead to misinformation and a loss of public trust in science. Therefore, keeping ethical practices at the forefront of data analysis is non-negotiable.
- Continuous Learning is Key: Data analysis is not static. Continuous education and workshops can help researchers keep up-to-date with tools and best practices.
Fostering a Data-Driven Research Culture
The importance of cultivating a data-driven research culture cannot be overstated. This involves:
- Investing in Training: Institutions must prioritize training for researchers in data analysis tools and techniques. Curating workshops and courses can empower individuals to utilize data to its full potential.
- Creating Cross-Functional Teams: By facilitating collaboration among individuals with diverse skill sets—from statisticians to domain experts—institutions can enrich the quality of data analysis and its application in research.
- Promoting Open Data: Encouraging transparency by making datasets publicly accessible allows for external verification and inspires others to engage in meaningful analysis, reinforcing the community’s commitment to rigorous research.
- Sharing Insights Widely: Engaging with broader audiences, including policymakers and the general public, about data findings can bridge the gap between specialized knowledge and real-world application. This encourages meaningful dialogue and action.
In the final analysis, the future of scientific inquiry lies in our ability to effectively navigate and leverage the landscape of data analysis. It is a journey that calls for vigilance, innovation, and most importantly, collaboration between all participants in the scientific endeavor.