Understanding Phi Test Results: A Comprehensive Guide
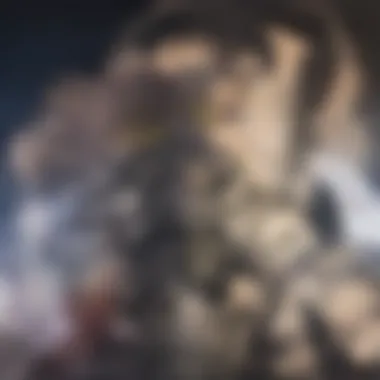
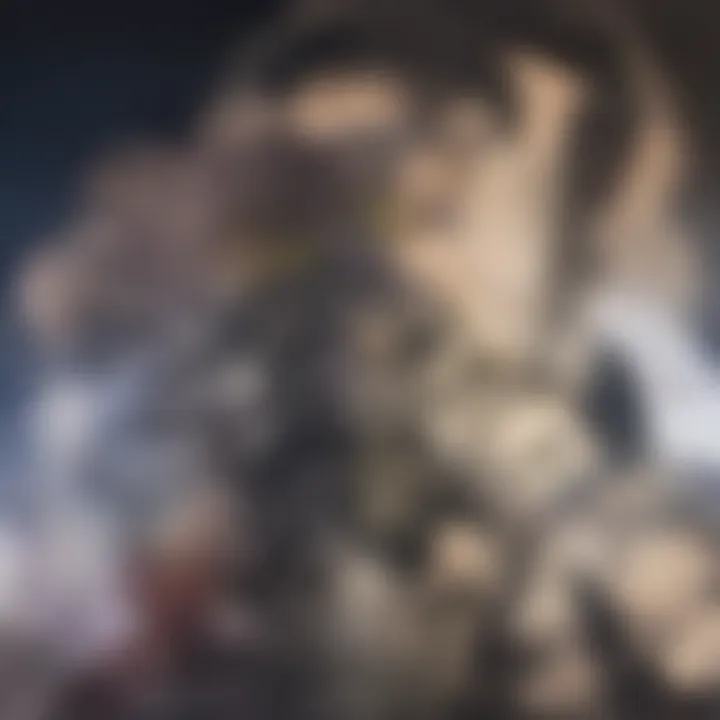
Article Overview
Purpose of the Article
This section aims to provide an understanding of phi test results, an essential tool in statistics. It seeks to clarify the concept, calculation, and significance of the phi coefficient in research involving binary data. By outlining the core principles and methodologies, this article serves as a guide for researchers and analysts aiming to utilize phi in various contexts. Key points will include how to interpret phi test results and their relevance in different fields of study.
Relevance to Multiple Disciplines
The application of the phi coefficient extends across various domains. In psychology, sociology, and health research, understanding relationships among binary variables can lead to more accurate conclusions. For example, health studies may investigate the relationship between a treatment (yes/no) and recovery status (yes/no). These insights allow for better decision-making and improved strategies in many fields.
Research Background
Historical Context
The phi coefficient was developed in the early 20th century. Its origins can be traced back to the work on correlation by Karl Pearson, who laid the groundwork for modern statistical methods. Initially, researchers sought to quantify the strength of association between two categorical variables. Over the years, phi has become a standard measure in statistics, especially useful when dealing with two-dimensional frequency tables.
Key Concepts and Definitions
Understanding the phi test necessitates familiarity with specific terms:
- Phi Coefficient: A measure used to determine the degree of association between two binary variables. Values range between -1 and +1.
- Binary Variables: Variables that can take on exactly two categories or values, such as true/false, presence/absence, or yes/no.
- Contingency Table: A table used to display the frequency distribution of variables, aiding in the calculation of the phi coefficient.
"The phi coefficient serves as a bridge between binary variables and insights crucial for data interpretation."
"The phi coefficient serves as a bridge between binary variables and insights crucial for data interpretation."
Understanding these foundational concepts is critical for researchers who aim to apply the phi coefficient effectively. Clear definitions set the stage for deeper analysis in subsequent sections.
Foreword to Phi Test
Understanding the phi test is pivotal for researchers working with categorical data. The phi coefficient serves as a critical statistical measure for evaluating the association between two binary variables. This is especially important in fields such as psychology, sociology, and epidemiology where binary outcomes frequently occur.
The benefits of effectively utilizing the phi test include gaining insights into correlations that may not be immediately apparent. It simplifies complex datasets by focusing on two-variable relationships. Additionally, it aids in hypothesis testing and decision-making processes. Therefore, a solid grasp of the phi test can enhance the quality of research outputs, ensuring accuracy and reliability in conclusions drawn.
Moreover, the phi coefficient has its limitations and assumptions that must be acknowledged. Researchers should acknowledge these factors in their analysis to avoid misinterpretations. A comprehensive understanding encompasses both its strengths and constraints, allowing for a balanced view when applying this statistic to real-world scenarios.
Overview of Statistical Measures
Statistical measures provide essential insights into data relationships. They quantify variables and enable researchers to make informed conclusions. The phi coefficient is but one of many statistical measures available, each serving distinct purposes. Common measures include correlation coefficients, measures of central tendency, and dispersion metrics.
- Correlation coefficients describe the strength and direction of relationships between variables.
- Measures of central tendency like mean and median summarize data distributions.
- Dispersion metrics evaluate data variability, essential for interpreting results accurately.
The phi coefficient is particularly relevant in situations involving binary variables, as it captures the nature of relationships between two discrete categories. This specificity adds value to research in fields where such dichotomous outcomes are common.
Historical Context
The development of the phi coefficient is rooted in the evolution of statistical theory. Introduced by the renowned statistician Karl Pearson in the early 20th century, this measure was initially designed to enhance data analysis methodologies. Pearson's contributions provided a groundwork for future statistical advancements, paving the way for the acceptance of various correlation measures.
Subsequently, the phi coefficient gained popularity through its application in social sciences and medical research. Researchers found it particularly useful in binary classification scenarios, such as disease presence or absence. With the growth of quantitative research methods, the phi test has been continually refined, leading to its widespread use today. Understanding its history allows researchers to appreciate its role in contemporary statistical practices and its ongoing relevance in modern research.
Calculating the Phi Coefficient
Calculating the phi coefficient is a critical process for understanding the relationship between two binary variables. Its role in statistical analysis cannot be understated. Accurately determining the phi value allows researchers to quantify associations, uncover correlations, and derive meaningful insights from data.
The phi coefficient itself is a measure of association for categorical variables, specifically binary categories. The significance of calculating this statistic lies in its ability to condense complex relationships into a single number. This not only simplifies interpretations but also facilitates comparisons between different studies and datasets.
Understanding Binary Variables
Binary variables are fundamental in many fields of research, including social sciences, medical studies, and market analysis. These variables represent two distinct categories that are mutually exclusive. For example, in a study examining the effectiveness of a medication, the variables may be defined as 'improved' or 'not improved'. Understanding the nature of binary variables is essential because the phi coefficient operates strictly with this type of data.
The key characteristics of binary variables include:
- Mutual exclusivity: Each participant or observation falls into one of the two categories without overlap.
- Dichotomy: The data representation is straightforward, simplifying both data collection and analysis.
- Simplicity: The binary system allows for easier computation and interpretation in statistical testing.
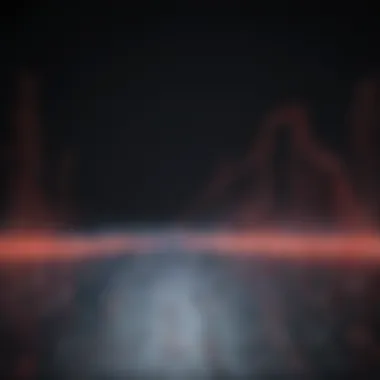
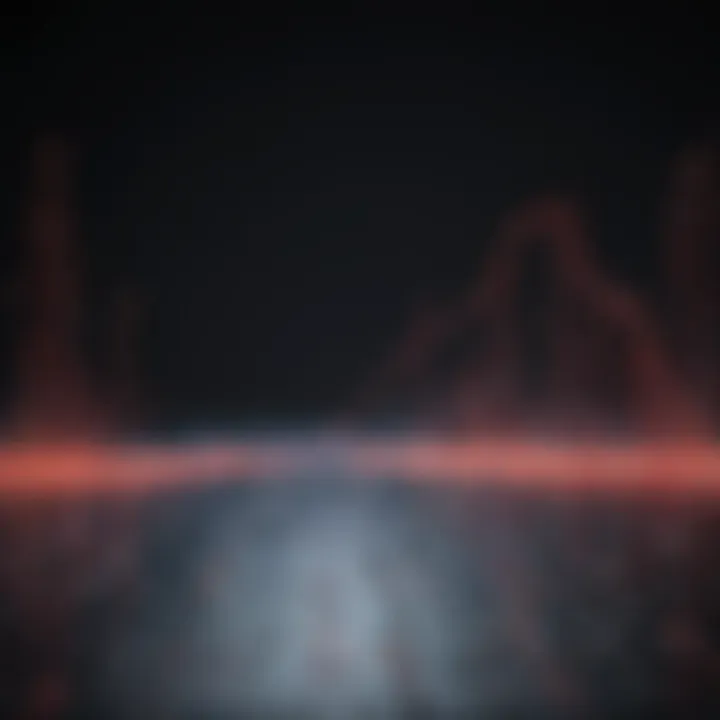
Formula Derivation
Deriving the formula for the phi coefficient involves understanding the concept of a contingency table. A 2x2 table displays the counts of occurrences for each category. The formula for the phi coefficient ( ( \phi )) is as follows:
In this formula, a, b, c, and d represent the counts from the contingency table:
- a: Number of cases in both category 1 (e.g. improved) and category 1.
- b: Number of cases in category 1 but not in category 2.
- c: Number of cases in category 2 but not in category 1.
- d: Number of cases in both category 2.
This formula considers how the occurrences in the different categories relate to one another, providing a robust measure of the strength of the association between the two binary variables in question.
Step-by-Step Calculation
Calculating the phi coefficient involves a systematic approach. Here are the steps:
- Construct a Contingency Table: Gather your data and arrange it into a 2x2 table based on the two binary variables being analyzed.
- Identify Counts: Determine the counts for a, b, c, and d from your table.
- Plug Values into Formula: Insert your counts into the phi coefficient formula mentioned above.
- Compute the Value: Execute the calculations. Ensure accuracy by taking care to follow the order of operations.
- Interpret Results: Based on the calculated value, interpret the strength and direction of the association. A phi value close to 1 indicates a strong positive association, while values close to -1 suggest a strong negative association.
The phi coefficient lends clarity to data interpretation, enabling informed decisions based on statistically validated relationships.
The phi coefficient lends clarity to data interpretation, enabling informed decisions based on statistically validated relationships.
In summary, calculating the phi coefficient is an essential process for researchers aiming to ascertain relationships between binary variables. Accurate calculations and understanding binary variables are paramount to producing valid and useful results.
Interpreting Phi Test Results
Interpreting phi test results is crucial in statistical analysis, particularly when evaluating binary variables. This section provides clarity on how to interpret these results correctly. Misinterpretation can lead to incorrect conclusions about the relationships between variables. Therefore, understanding how to read the phi coefficient and its implications is essential for accurate analysis.
Understanding Interpretation Scale
The phi coefficient ranges from -1 to +1. A positive phi value indicates a positive association between the two variables. This means that as one variable increases, the other variable tends to increase as well. Conversely, a negative value suggests a negative association, indicating that an increase in one variable is associated with a decrease in the other. A phi value close to 0 indicates little to no association between the variables.
It is important to note some common thresholds for interpreting this scale:
- 0.1 to 0.3 generally indicates a weak association.
- 0.3 to 0.5 indicates a moderate association.
- Above 0.5, a strong association is typically observed.
Notably, the context of the variables is essential when interpreting these thresholds. Different fields may have different standards for what constitutes a strong or weak correlation.
Contextual Relevance
Context plays a vital role in interpreting phi test results. The implications of a given phi value can vary depending on the research area and the specific variables being analyzed. For instance, in health sciences, a small phi value might indicate a critical relationship, while in social sciences, researchers might expect different norms for interpretation.
Furthermore, it is crucial to recognize the potential for confounding factors that may influence the phi value. These factors can create misleading interpretations if not accounted for in the analysis process. Thus, researchers should always consider the broader context when interpreting their results.
Applications of Phi Coefficient
The phi coefficient serves as a pivotal tool in statistical analysis, particularly for examining relationships between two binary variables. Understanding its applications is key for researchers seeking to elucidate complex data relationships. The relevance of the phi coefficient spans numerous disciplines. This versatility can unlock insights across fields such as psychology, medicine, sociology, and even marketing. A closer examination reveals how various sectors implement phi to convert data into actionable insights.
Usage in Various Disciplines
The applications of phi encompass a broad spectrum of fields. Each domain employs this statistic with unique perspectives and purposes. Below are a few prominent fields where the phi coefficient is extensively utilized:
- Psychology: In psychological research, phi aids in analyzing the relationship between behaviors and categorical variables. For example, it can discern the correlation between various mental health disorders and demographic factors.
- Medical Studies: In clinical research, phi can illustrate the association between patient characteristics and treatment outcomes. Understanding how factors like gender or age correlate with the success of specific therapies can significantly influence treatment protocols.
- Sociology: Sociologists often leverage the phi coefficient to explore social behaviors and attitudes. Whether examining voting patterns or social interactions, phi enables the investigation of dependencies between categorical variables.
- Marketing Analytics: Businesses analyze consumer behavior and purchasing patterns. The phi coefficient helps uncover relationships in survey responses, aiding in segmentation and targeting strategies based on customer preferences.
Each discipline approaches the phi coefficient through the lens of its unique questions and objectives. This breadth of use demonstrates phi's importance in transforming categorized data into meaningful insights.
Case Studies
To illustrate the practical implication of phi, consider a few case studies that exemplify its utility.
- Mental Health Research: A study explored the relationship between anxiety levels and gender. The phi coefficient was calculated from survey data, showing a significant association. This finding helped refine therapeutic approaches specifically aimed at different gender groups.
- Clinical Trials: A randomized controlled trial assessed the efficacy of a new medication. Researchers used the phi coefficient to examine the relationship between treatment adherence and patient demographics. The analysis provided insights into which groups were more likely to benefit from additional support.
- Consumer Behavior: A retail chain conducted a survey to assess customer satisfaction regarding a new product line. By applying the phi coefficient, the company could identify significant correlations between demographic variables and satisfaction levels. This knowledge guided marketing strategies to enhance customer engagement.
These examples highlight the significant role of phi in producing actionable results from binary data. As it becomes clear, the phi coefficient not only articulates correlations but also facilitates informed decision-making across diverse fields. This ability to synthesize complex data into available insights underscores the phi coefficient's importance in statistical analysis.
Comparing Phi with Other Correlation Measures
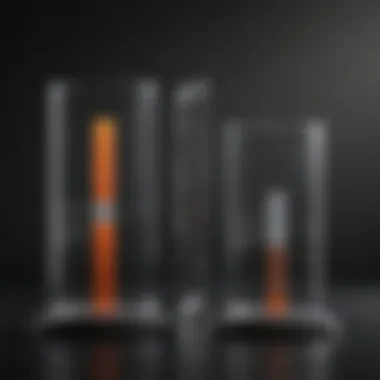
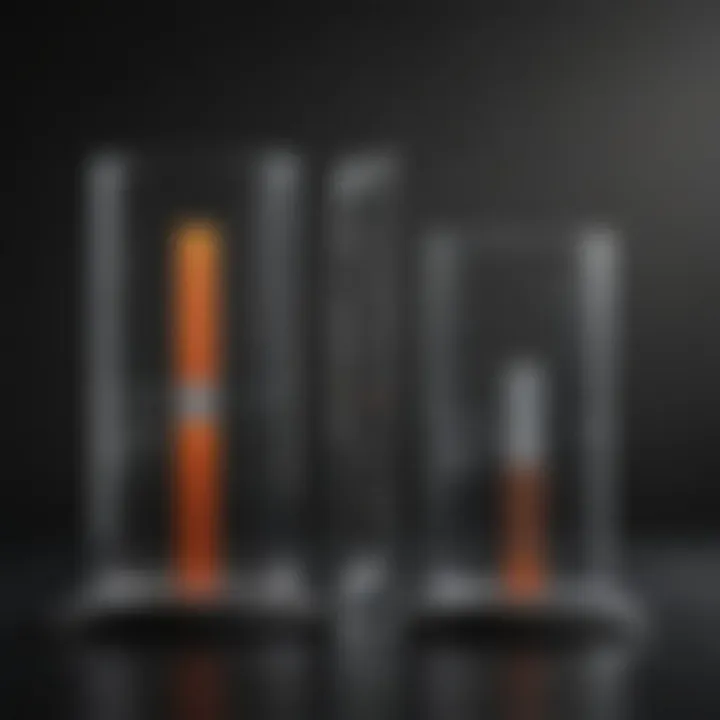
When examining the relationships between binary variables, the phi coefficient stands out as a fundamental metric for statistical analysis. However, it is crucial to consider how this measure interacts with other correlation coefficients like Spearman’s Rank Correlation and the Pearson Correlation Coefficient. Understanding these comparisons helps in selecting the most appropriate statistical tool for a given analysis, depending on the data characteristics and the research objectives. This section will outline important aspects regarding each correlation method and highlight specific benefits and considerations.
Spearman’s Rank Correlation
Spearman’s Rank Correlation is a non-parametric test that assesses how well the relationship between two variables can be described using a monotonic function. Unlike the phi coefficient, which is best suited for binary data, Spearman’s is applicable to ordinal and continuous data sets without assuming a linear relationship. The primary feature of Spearman's correlation is that it ranks the data points; thus, it is less sensitive to outliers than Pearson's method.
A few key points about Spearman’s Rank Correlation include:
- Data types: It can handle ordinal data, making it versatile across different data types.
- Monotonic relationships: It captures monotonic trends which are not necessarily linear.
- Performance with outliers: Outliers have a lesser impact due to ranking, which can lead to more reliable correlation estimates.
In specific scenarios, particularly in social sciences, Spearman’s is favored when data cannot meet the assumptions of normality required by other tests.
Pearson Correlation Coefficient
The Pearson Correlation Coefficient measures the linear relationship between two continuous variables. Unlike Phi, which is binary-focused, Pearson assumes that the underlying data distributions are normal and that there is a linear association between the variables. When these assumptions hold true, Pearson can effectively reveal the strength and direction of correlations.
Key characteristics of the Pearson Correlation Coefficient include:
- Assumptions: It requires interval or ratio data and assumes that both variables are normally distributed.
- Sensitivity to linear relationships: Pearson will effectively identify linear relationships, but it may miss non-linear associations.
- Impact of outliers: Pearson's measures can be easily skewed by outliers, which are less impactful in Spearman's method.
Limitations of Phi
Understanding the limitations of the phi coefficient is crucial for both researchers and analysts. While it is a widely used statistical measure for examining the association between two binary variables, it is not without its challenges. Recognizing these limitations enables more accurate interpretations of findings and promotes better methodological approaches.
Assumptions and Constraints
The phi coefficient is predicated on certain assumptions that must be met for its results to be valid. One primary assumption is that the data must be comprised of two categorical variables that are dichotomous in nature. This means the variables must have only two categories, such as yes/no or success/failure. Additionally, independence of observations is essential; each case should only belong to one category without any interference from other observations.
Another constraint is the sensitivity of phi to the distribution of the data. In datasets with more severe imbalances between the categories, phi can yield misleading results. For instance, if one category has significantly more observations than the other, the phi value may falsely suggest a strong correlation when the relationship may not be as substantial as indicated.
It is imperative to assess the context of the data and whether the assumptions of the phi test are being upheld.
It is imperative to assess the context of the data and whether the assumptions of the phi test are being upheld.
Impacts of Sample Size
Sample size plays a crucial role in the reliability of phi test results. A small sample size can lead to unstable estimates of the phi coefficient, increasing the possibility of Type I and Type II errors. With limited data points, even trivial associations may appear statistically significant, which can misguide interpretations. Conversely, a larger sample size generally provides a more accurate estimation of the phi coefficient by better capturing the underlying relationship between the variables.
This amplification effect of sample size on statistical power is particularly notable when testing for significance. As sample size increases, the confidence interval around the phi coefficient narrows, lending more credibility to the results. Researchers should therefore prioritize adequate sample sizes to attain valid and reliable phi coefficients, ensuring robust statistical conclusions.
Addressing Potential Pitfalls
Understanding the potential pitfalls in analyzing phi test results is crucial for accurate interpretation and reporting. This section highlights the key aspects that researchers must consider to avoid erroneous conclusions. By addressing these issues, analysts can maintain the integrity of their findings and contribute valuable insights to their respective fields.
Common Misinterpretations
Many researchers misinterpret the phi coefficient's results. One common misconception is that a high phi value indicates a strong causal relationship between the variables in question. In reality, phi measures association, not causation. Correlation does not imply that changes in one variable directly influence the other. Analysts should be clear about this distinction when presenting results to prevent misleading implications.
Another misinterpretation involves the numeric value of the phi coefficient. Some believe that the scale from -1 to 1 operates similarly to other correlation measures. However, for phi, values close to 0 signify weak associations, while values approaching 1 or -1 denote strong associations. This confusion can lead to overestimation of variable connections and misinformed decisions. Ensuring that the audience fully understands these facets is necessary for robust scientific communication.
Ensure Data Quality and Integrity
The quality of data used in phi calculations is fundamental to obtaining reliable results. To maintain high-quality data, analysts should adhere to rigorous data collection methods. This involves verifying that the binary variables are correctly defined and consistently measured. Inaccuracies in coding or categorizing data can lead to distorted phi values.
Once collected, data must also be cleaned rigorously. This step includes removing outliers or erroneous entries that may skew results. Employing statistical tests prior to phi calculations can help identify potential inconsistencies within the dataset.
Moreover, analysts should consider sample size carefully. A small sample can result in unstable phi values, leading to misinterpretation. Statistical power analysis may help determine the appropriate sample size required for valid conclusions.
Ensuring the quality and integrity of data leads to a more accurate phi calculation and reliable test results. Researchers should prioritize these practices to enhance the trustworthiness of their findings.
Contextual Factors Influencing Phi Results
Understanding the context in which phi test results are generated is critical. This section explores the various contextual factors that can affect the interpretation and reliability of phi coefficient values. Two key categories of these factors include domain-specific applications and temporal and spatial considerations. Each of these elements enhances our grasp of how phi can be utilized effectively in different research scenarios.
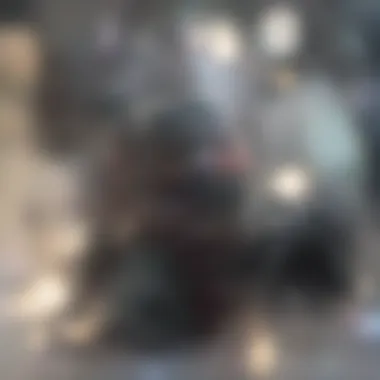
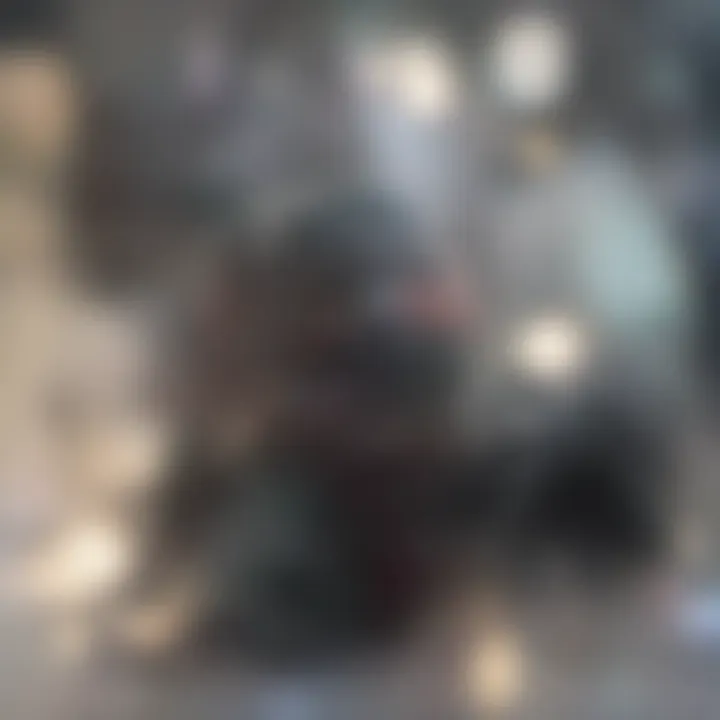
Domain-Specific Applications
In various fields, the phi coefficient may take on distinct meanings based on its application. The specifics of each domain can provide crucial insights into how these test results are understood. For instance:
- Medicine: In a medical context, phi can illustrate associations between symptoms and diagnoses. If a study shows a phi coefficient of 0.5 between smoking and lung cancer diagnosis, it suggests a moderate correlation, informing public health strategies.
- Social Sciences: Researchers often use phi to examine the relationship between demographic variables and behavioral outcomes. In this context, a high phi value could indicate significant effects of social factors on certain behaviors.
- Education: Educational researchers may use phi to examine correlations between student demographics and performance metrics. Understanding these relationships can influence policy and intervention strategies in educational institutions.
Recognizing these variations in domain applications allows researchers to contextualize the significance of their results better.
Temporal and Spatial Considerations
Time and location are critical when interpreting phi coefficients. Changes in data collection methods or temporal events can lead to different phi outcomes. For instance:
- Temporal Factors: Research conducted over a short period may yield different results compared to longitudinal studies. In a study examining patient recovery rates over time, a phi coefficient calculated at one point may not represent trends effectively.
- Spatial Factors: The geographical context can shape how phi results are perceived. A strong association observed in one region may not hold in another due to cultural or environmental factors affecting the studied variables.
Integrating Phi Test Findings into Research
Integrating the findings from phi tests into larger research frameworks is important for several reasons. First, this statistical measure provides insights into the relationship between two binary variables. These relationships can be pivotal in various fields, including psychology, sociology, and medical research. When handled correctly, the phi coefficient adds depth to research findings, making them more robust and easier to interpret.
Careful integration also ensures that researchers communicate their results effectively. This involves more than simply presenting statistical values; it requires putting phi findings in context to show their significance. This context not only aids understanding among peer researchers but also improves the clarity of findings in public or educational settings.
Additionally, several factors should be considered when integrating phi findings. It is crucial to account for the data's integrity, the method of analysis, and how the findings relate to existing literature. By synthesizing raw data through proper analysis and interpretation, researchers can make informed conclusions and recommendations based on their phi test results.
"Integration of statistical findings with research narratives enriches the overall impact of the study."
"Integration of statistical findings with research narratives enriches the overall impact of the study."
Reporting Standards and Practices
Reporting phi test findings must adhere to rigorous standards. Clear communication of results is fundamental to ensure that other researchers understand and can replicate studies. This clarity enhances the credibility of the research and supports its acceptance in the academic community.
To report phi test findings:
- State the phi coefficient clearly, including its value and confidence intervals.
- Discuss the p-value and its implications regarding the statistical significance of findings.
- Describe the sample size and its implications on the interpretation of phi results. A larger sample size may suggest a more reliable coefficient.
- Use appropriate tables or graphs to illustrate the binary variables in the study.
Such standards enhance transparency and allow for a more comprehensive evaluation of research validity.
Citations and References
Accurate citations and references are essential for academic integrity in reporting phi test findings. Proper crediting of sources allows for the validation of findings and bolsters the study's reliability. Researchers must ensure that they cite relevant literature that assists in understanding the relationship assessed by the phi test.
Here are some guidelines for citing sources effectively:
- Always follow citation styles such as APA, MLA, or Chicago, as required by your field.
- Include primary and secondary sources where the phi coefficient has been discussed or applied.
- Use reputable journals and online databases, such as en.wikipedia.org or britannica.com, for foundational information.
By maintaining rigorous citation practices, researchers contribute to the academic discourse surrounding phi tests and facilitate further investigation into binary variable relationships.
Future Outlook on Phi and Statistical Analysis
The future outlook on phi and statistical analysis is crucial for navigating the evolving landscape of data interpretation and statistical modeling. As research progresses, the demand for reliable and comprehensive methods to analyze binary variables grows. Understanding the phi test results equips researchers with a fundamental tool for deciphering relationships within datasets. This importance cannot be overstated, particularly when considering the significant role statistics play in decision-making across disciplines.
Advancements in Statistical Methods
Advancements in statistical methods continually reshape the way researchers approach data analysis. These innovations often enhance the robustness and applicability of the phi coefficient. Some key areas of progress include:
- Increased computational power: With advances in technology, the ability to handle large datasets has vastly improved. This allows for more complex analyses that can incorporate the phi coefficient in nuanced ways.
- Integration of machine learning: Renowned for providing predictive insights, machine learning methods can complement traditional statistical measures. By integrating phi with machine learning frameworks, researchers can improve the interpretability of binary data across diverse fields.
- Bayesian approaches: A shift towards Bayesian statistics enables researchers to incorporate prior knowledge, enhancing the analysis of relationships reflected by the phi coefficient. This adds context and depth to the results, increasing their informational value.
These advancements serve not only to refine existing methods but also to broaden the applicability of the phi coefficient beyond conventional settings.
Emerging Trends in Analytical Practices
Emerging trends in analytical practices point towards a more holistic approach in statistical evaluation. Such shifts can redefine how the phi coefficient is utilized in research. Key trends include:
- Focus on reproducibility: There is a growing emphasis on producing research that is reproducible. Adhering to strict methodological standards enhances the reliability of phi test results. This practice builds trust in findings across the scientific community.
- Interdisciplinary collaboration: Collaborations across disciplines bring in varying perspectives, enriching the context in which the phi coefficient is applied. This fosters innovative methodologies, encouraging the exploration of the relationships between binary variables in novel ways.
- Data transparency: Open data initiatives promote transparency in research methods and results. By sharing datasets, researchers provide others the tool for independent verification of phi test results, thereby reinforcing their validity.
As researchers adopt these emerging trends, the relevance and importance of the phi coefficient in statistical analysis will likely continue to rise.
As researchers adopt these emerging trends, the relevance and importance of the phi coefficient in statistical analysis will likely continue to rise.
Overall, both advancements in statistical methods and emerging trends in analytical practices highlight an exciting future for the phi coefficient. Researchers must remain adaptable, leveraging these developments to enhance their understanding and application of phi test results in varying contexts.